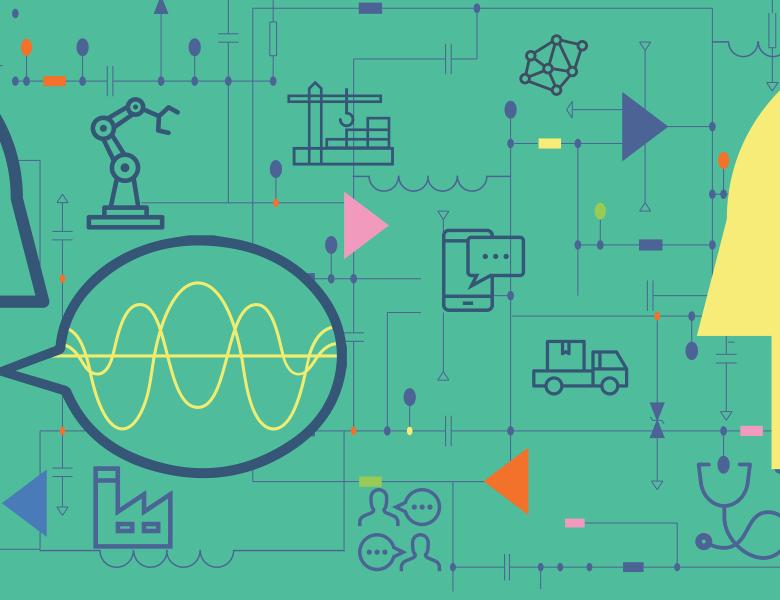
About
This program aims to advance the theoretical foundations of reinforcement learning (RL) and foster new collaborations between researchers across RL and computer science.
Recent years have seen a surge of interest in reinforcement learning, fueled by exciting new applications of RL techniques to various problems in artificial intelligence, robotics, and natural sciences. Many of these advances were made possible by a combination of large-scale computation, innovative use of flexible neural network architectures and training methods, and new and classical RL algorithms. However, we lack a solid understanding of when, why, and to what extent these algorithms work.
Reinforcement learning's core issues, such as efficiency of exploration and the trade-off between the scale and the difficulty of learning and planning, have received concerted study over the last few decades within many disciplines and communities, including computer science, numerical analysis, artificial intelligence, control theory, operations research, and statistics. The result has been a solid body of work that has built and resolved some of the core problems; yet, the most pressing problems, concerning how one can design highly scalable algorithms, still remain open.
This program aims to reunite researchers across disciplines that have played a role in developing the theory of reinforcement learning. It will review past developments and identify promising directions of research, with an emphasis on addressing existing open problems, ranging from the design of efficient, scalable algorithms for exploration to how to control learning and planning. It also aims to deepen the understanding of model-free vs. model-based learning and control, and the design of efficient methods to exploit structure and adapt to easier environments.