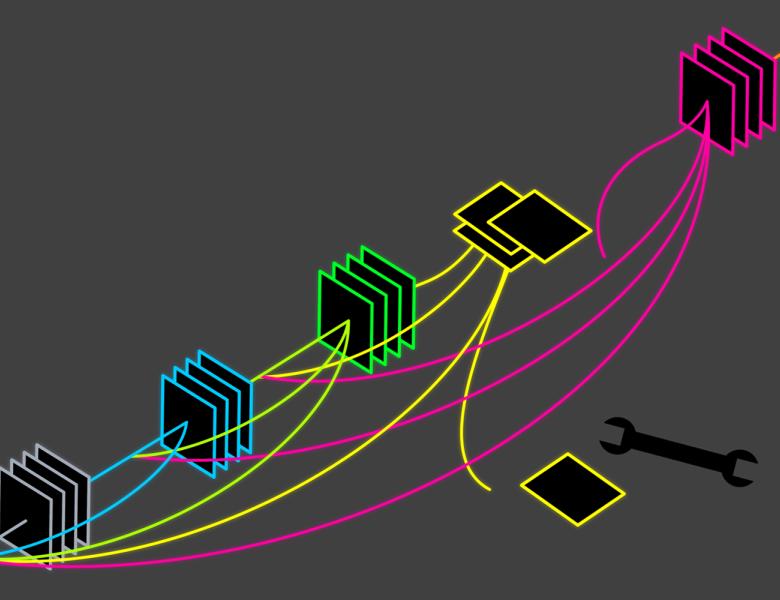
About
Deep learning is the engine powering many of the recent successes of artificial intelligence. These advances stem from a research effort spanning academia and industry; this effort is not limited only to computer science, statistics, and optimization, but also involves neuroscience, physics, and essentially all of the sciences. Despite this intense research activity, a satisfactory understanding of deep learning methodology — and, more importantly, its failure modes — continues to elude us. As deep learning enters sensitive domains, such as autonomous driving and health care, the need for building this kind of understanding becomes even more pressing.
The goal of this program was to address this need by aligning and focusing theoretical and applied researchers on the common purpose of building empirically relevant theoretical foundations of deep learning. Specifically, the intention was to identify and make progress on challenges that, on one hand, are key to guiding the real-world use of deep learning and, on the other hand, can be approached using theoretical methodology.
The program focused on the following four themes:
- Optimization: How and why can deep models be fit to observed (training) data?
- Generalization: Why do these trained models work well on similar but unobserved (test) data?
- Robustness: How can we analyze and improve the performance of these models when applied outside their intended conditions?
- Generative methods: How can deep learning be used to model probability distributions?
An integral feature of the program was bridging activities that aimed to strengthen the connections between academia and industry. In particular, in addition to workshops and other weekly events, the program hosted weekly bridging days that brought together local Bay Area industry researchers and regular program participants.
This program was supported in part by the Patrick J. McGovern Foundation.
List of Weekly Visitors:
Anima Anandkumar (California Institute of Technology and Nvidia), Yasaman Bahri (Google Brain), Samy Bengio (Google), Paul Christiano (OpenAI), Shalini De Mello (NVIDIA), Inderjit Dhillon (Amazon), Vitaly Feldman (Google Brain), Jonathan Frankle (Facebook), Mohammad Ghavamzadeh (Facebook AI Research), Dan Hill (Amazon), T.S. Jayram (IBM Almaden Research), Tomer Koren (Google Research), Ming-Yu Liu (Nvidia), Philip Long (Google Brain), Nimrod Meggido (IBM Almaden Research Center), Ofer Meshi (Google), Ilya Mironov (Google Brain), Hossein Mobahi (Google), Qie Hu (Amazon), Jakub Pachocki (OpenAI), Rina Panigrahy (Google Brain), Maithra Raghu (Google Brain), Nima Reyhani (AirBnB), Sujay Sanghavi (Amazon), Sam Schoenholz (Google Brain), Hanie Sedghi (Google Brain), Rajat Sen (Amazon), Szymon Sidor (OpenAI), Yoram Singer (Google Brain), Jascha Sohl-Dickstein (Google Brain), Kunal Talwar (Google), Felix Juefei Xu (Alibaba Group), Laura Zaremba (Groq), Kai Zhong (Amazon)