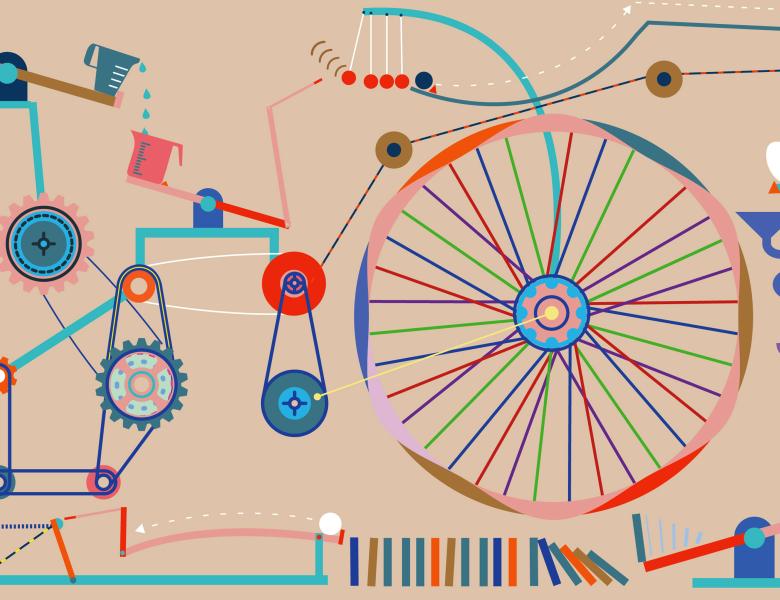
About
This program aims to integrate advances and techniques from theoretical computer science into methods for causal inference and discovery.
Although attempts to characterize causal relations can be found in some of the oldest written records, the history of the usage of causal concepts within scientific discussions over the past 100 years has been rocky, varying from the outright denial of any role of causality in mature scientific theories to a disingenuous usage of ambiguous terms that obscure the role of cause and effect (e.g., "link," "connection," etc.).
A substantive development of new formal approaches to causality in the 1970s and 1980s precipitated a change in attitude toward the scientific investigation of causal questions. The change was led by the development of two largely intertranslatable mathematical frameworks: the potential outcome framework and the causal graphical models framework. These frameworks integrated three concepts central to the notion of causation: (1) the connection between the underlying causal relations and observed data, (2) the difference that interventions can make to a causal system, and (3) counterfactual statements about a system. All these aspects of causality play a central role in scientific testing and explanation, often constituting the goal of scientific inquiry itself.
The mathematization of questions of causality has resulted in the development of inference techniques and learning methods to infer causal relations from data. These formal approaches are now starting to spread throughout the applied sciences, where just about any field of study is seeing a renewed and explicit interest in tackling causality.
Broad application of these theoretical frameworks in scientific domains requires not only conceptual clarity and "in principle" methods, but a detailed understanding of how the methods behave in practice, how to scale and approximate the ideally desired computations, and how to optimize methods for the particular constraints present in a domain.
This program will bring together theoretical and applied researchers from a broad variety of domains with the goal of understanding the complexity, optimizations, and possible approximation regimes required to turn the methods of causal inference into a broadly applicable scientific toolbox.
This program is supported in part by the Alfred P. Sloan Foundation.
This program is partially supported by the Foundations of Data Science Institute. We gratefully acknowledge the support of the NSF through grant DMS-2023505.