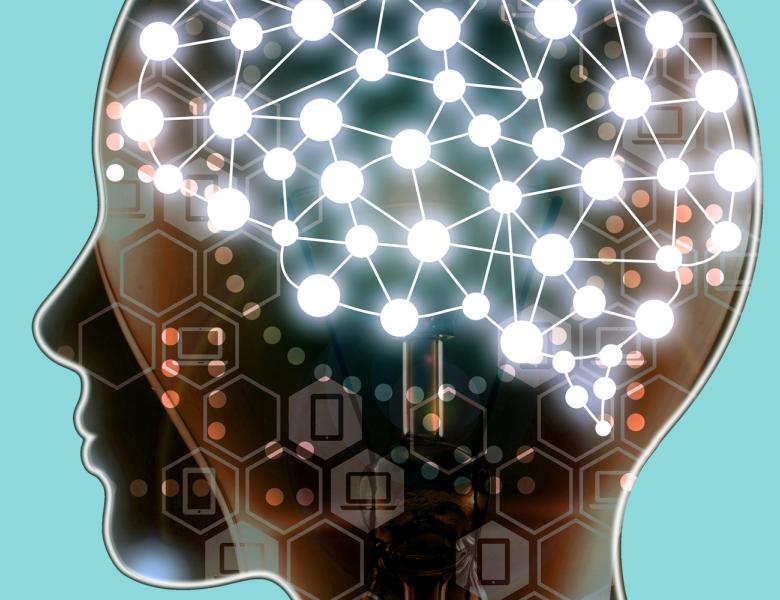
About
The Research Pod in Machine Learning brings together researchers from theoretical computer science, mathematics, statistics, electrical engineering, and economics to develop the theoretical foundations of machine learning and data science. Led by Peter Bartlett, this pod is partially funded by a $12.5 million award made under the National Science Foundation's program on Transdisciplinary Research in Principles of Data Science to establish the Foundations of Data Science Institute (FODSI). This institute, a collaboration between UC Berkeley and MIT, partnering with Boston, Northeastern, Harvard and Howard universities, as well as Bryn Mawr College, aims to improve our understanding of critical issues in data science, including modeling, statistical inference, computational efficiency, and societal impacts. NSF, together with the Simons Foundation, is also supporting the activities of the pod through the Collaboration on the Theoretical Foundations of Deep Learning. This is a collaboration of 11 PIs from eight institutions around the world that aims to understand the mathematical mechanisms that underpin the practical success of deep learning. The Simons Institute will act as the convening center for many of these activities, hosting public events such as summer schools, research workshops, and other collaborative research opportunities.
To participate as a fellow in the Simons ML Pod, apply directly to one of the following two programs:
- Foundations of Data Science Institute (FODSI)
- Collaboration on the Theoretical Foundations of Deep Learning
Also, the Simons Institute programs in AY 24-25 may be of special interest to machine learning researchers. Information on applying for a fellow position in one of the semester-long programs is here.
Supported by: