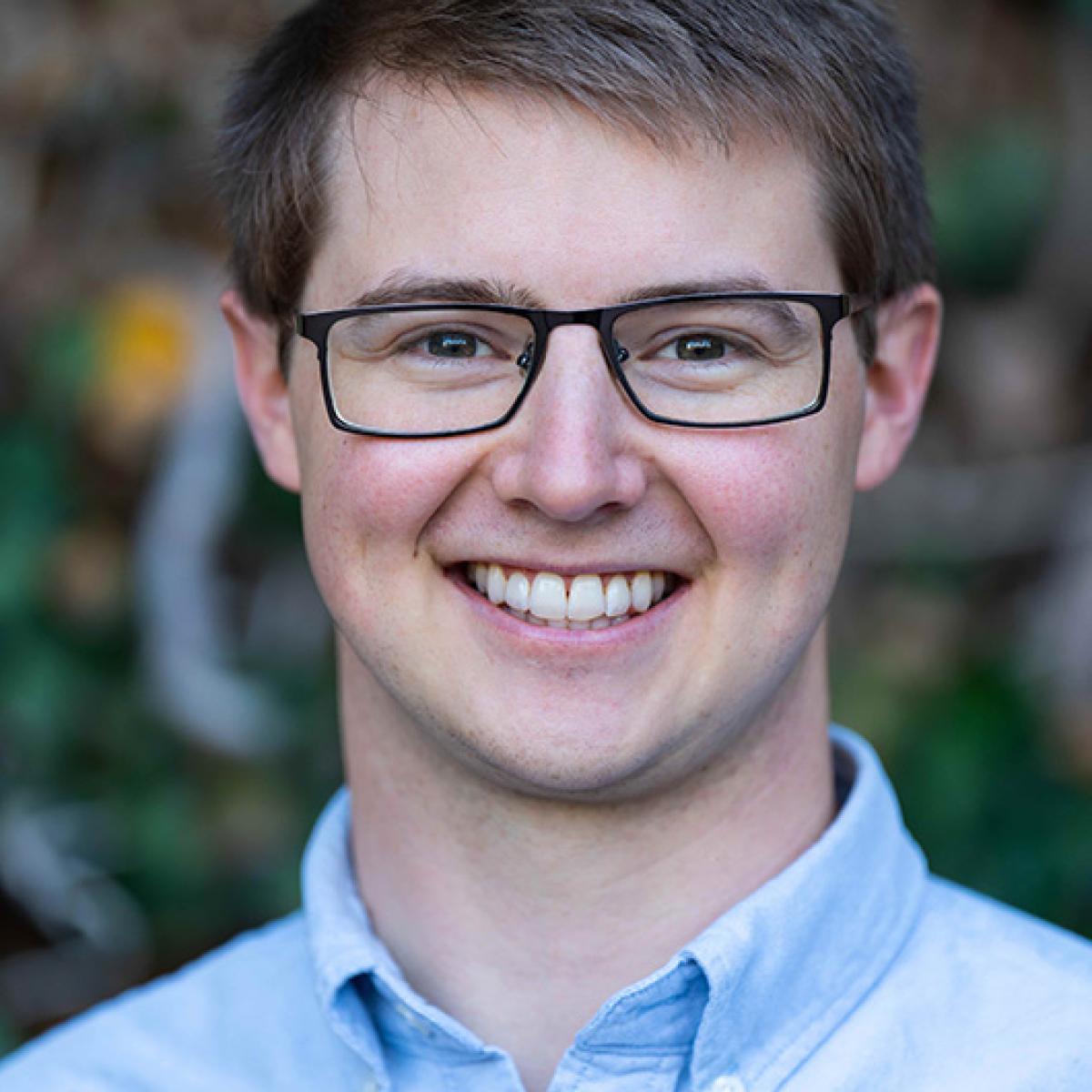
Stephen Bates
Stephen Bates is a postdoctoral researcher with Michael I. Jordan in the Statistics and EECS departments at UC Berkeley. He works on developing methods to analyze modern scientific data sets, leveraging sophisticated black box models while providing rigorous statistical guarantees. Specifically, Stephen works on problems in high-dimensional statistics (especially false discovery rate control), statistical machine learning, conformal prediction and causal inference.
Previously, Stephen completed his PhD in the Stanford Department of Statistics advised by Emmanuel Candès. His thesis introduced methods for conditional independence testing and false discovery rate control in genomics, and he received the Ric Weiland Graduate Fellowship and the Theodore W. Anderson Theory of Statistics Dissertation Award for this work. Before his PhD, he studied statistics and mathematics at Harvard University.