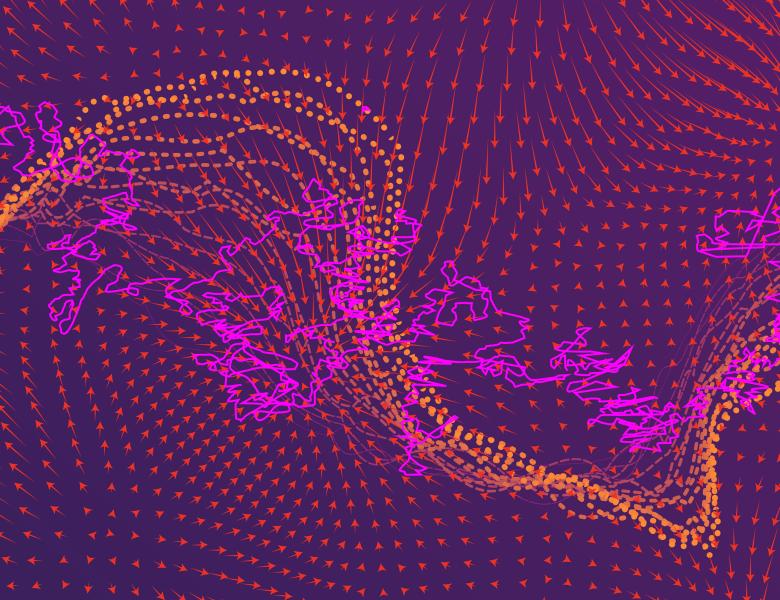
About
Optimization and sampling are two of the most important mathematical topics at the interface of data science and computation. The two questions are, in fact, connected mathematically through a powerful framework articulated around the geometry of probability distributions. The geometric toolbox that underlies optimization and sampling was initiated in the study of partial differential equations (PDEs) and has evolved into different mathematical disciplines: probability, calculus of variations, analysis and geometry. While connections are slowly beginning to percolate across disciplines, this program is aimed to be a catalyst for new and interdisciplinary ideas using a principled and unified approach to optimization and sampling.
A central goal of this program is to develop and promote a geometric approach to various computational problems in sampling, optimization, and PDEs. For example, the geometry of Optimal Transport has been instrumental to establish fruitful connections between diffusion processes, gradient flows, and diffusive PDEs by eliciting hidden convexity. This success calls for a versatile toolbox to tackle algorithmic questions arising in sampling, optimization, and particle methods for solving PDEs by leveraging the hidden geometric structure of each problem in a systematic way. Moreover, in a large class of problems this geometric structure is supplemented by additional symmetries or other algebraic structures that can be exploited to design better algorithms.
These recent connections between sampling, optimization, and PDEs have placed the fields in a unique position for mutual impact. This program aims at bringing together researchers from various backgrounds to tackle these challenging problems using a unified approach by focusing on the following aspects:
- Sampling as an optimization problem
- Geometry and optimal transport
- The PDE perspective on sampling and optimization
- Eliciting convexity via geometry in sampling and optimization
- The interplay of algebra and geometry in optimization