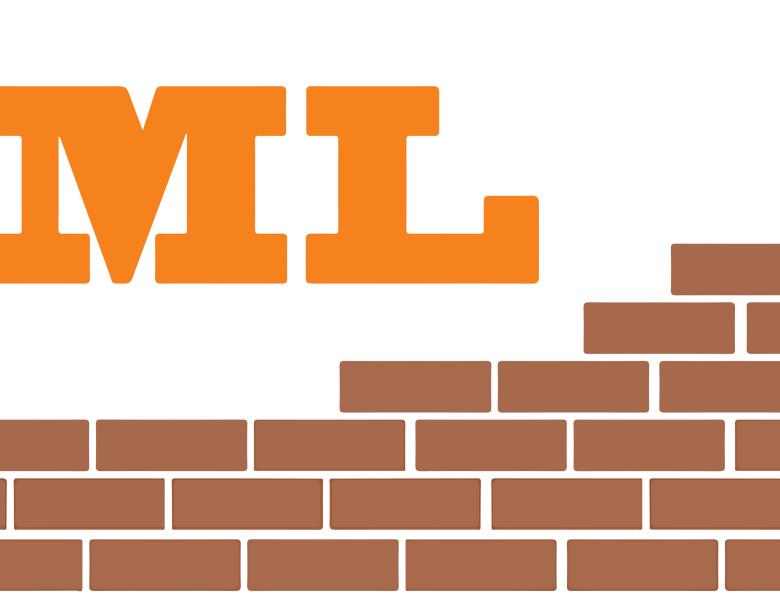
About
The goal of this program was to grow the reach and impact of computer science theory within machine learning.
One central component of the program was formalizing basic questions in developing areas of practice and gaining fundamental insights into these. Target areas of particular interest were interactive learning and representation learning. Interactive learning consists of scenarios in which the communication between human and learner is richer than a one-way transmission of labeled examples; this happens, for instance, in teaching, or explanation-based learning, and in crowdsourcing. Representation learning studies intermediate-
A second component of the program was advancing the algorithmic frontier of machine learning. Target areas included Bayesian statistics, in which many of the core algorithmic problems bear similarity to problems that have been studied intensively in the theoretical computer science community, and large-scale
A final component of the program was understanding heuristics: what works in practice and why. The most popular algorithms for a variety of basic statistical tasks —