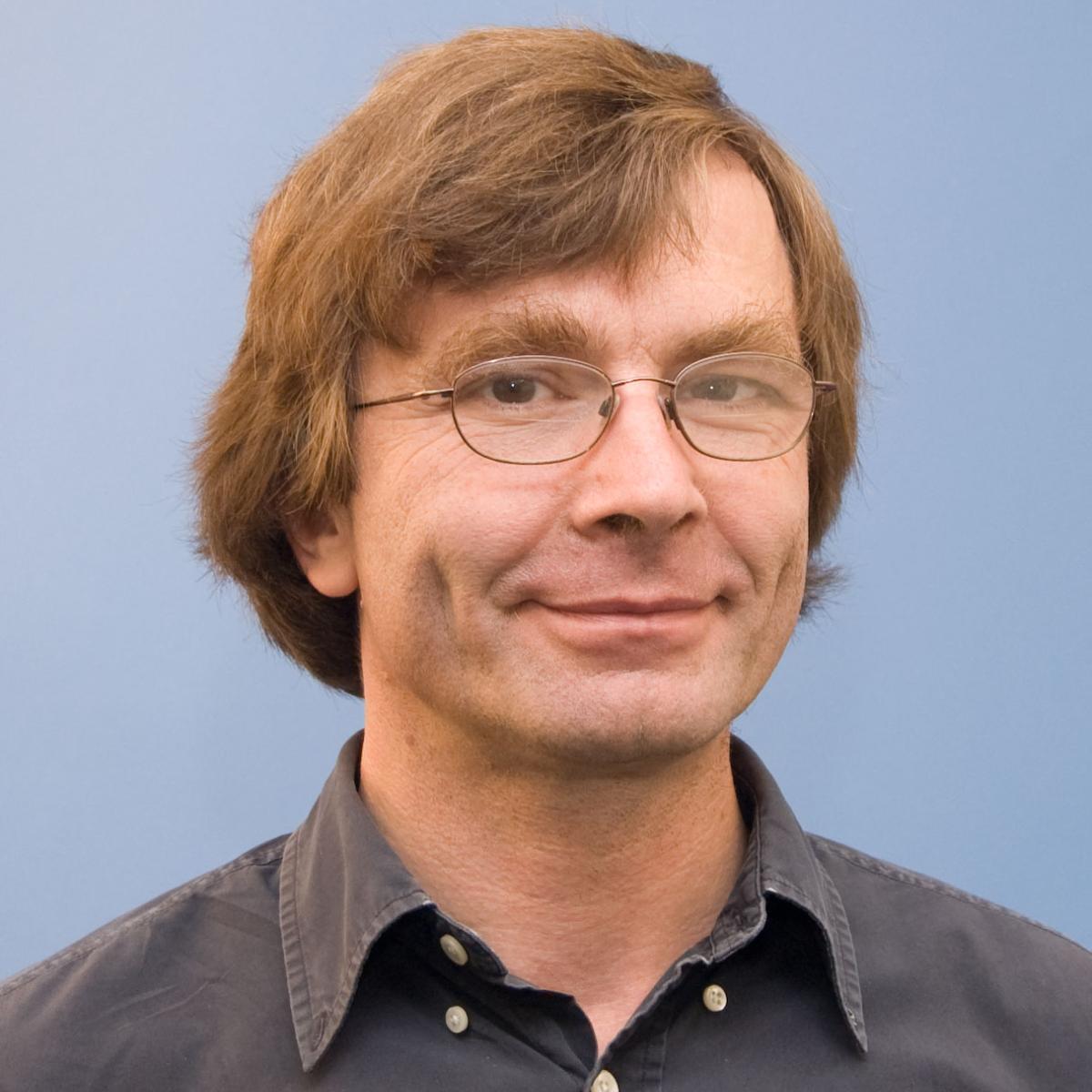
Manfred Warmuth
Manfred Warmuth is one of the founding members of the COLT community. He is known for developing a number of key online learning algorithms, such as the Weighted Majority and Exponentiated Gradient algorithms. He pioneered the derivation and analysis of online algorithms based on Bregman divergences, and developed a number of methods with his collaborators that make online algorithms robust to changing data. Much of his research contrasts the advantages of the additive versus multiplicative updates. More recently, he has focused on developing online algorithms using matrix parameters. In particular, he has generalized with his collaborators the multiplicative updates from probability vectors to density matrices, and developed a Bayesian probability calculus for density matrices. He is also well known for generating many open problems for the Machine Learning community. One example is the compression scheme conjecture, which states that samples of concepts from a concept class of VC dimension d can be compressed to subsamples of up to d many points.