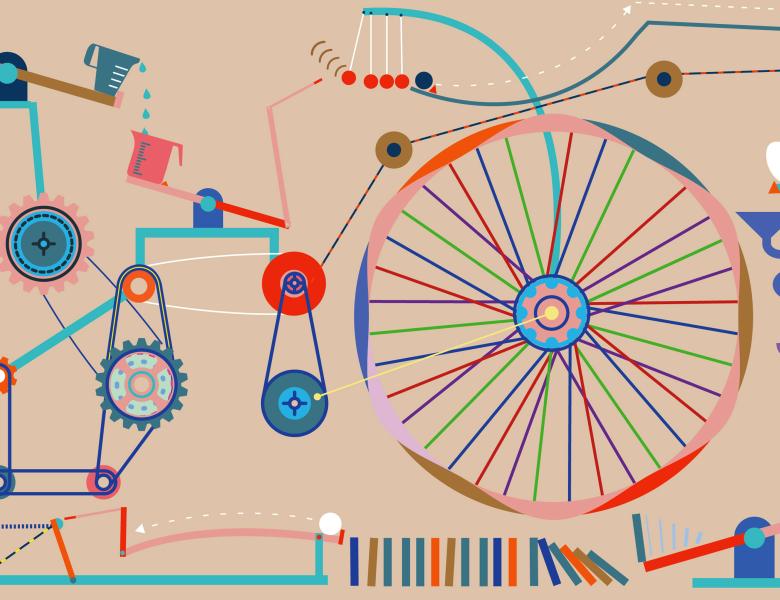
Playlist: 25 videos
Algorithmic Aspects of Causal Inference
0:27:40
Angela Zhou (Berkeley)
https://simons.berkeley.edu/talks/confounding-robust-policy-evaluation-infinite-horizon-reinforcement-learning
Algorithmic Aspects of Causal Inference
Off-policy evaluation of sequential decision policies from observational data is necessary in applications of batch reinforcement learning such as education and healthcare. In such settings, however, unobserved variables confound observed actions, rendering exact evaluation of new policies impossible, i.e., unidentifiable. We develop a robust approach that estimates sharp bounds on the (unidentifiable) value of a given policy in an infinite-horizon problem given data from another policy with unobserved confounding, subject to a sensitivity model. We consider stationary or baseline unobserved confounding and compute bounds by optimizing over the set of all stationary state-occupancy ratios that agree with a new partially identified estimating equation and the sensitivity model. We prove convergence to the sharp bounds as we collect more confounded data. Although checking set membership is a linear program, the support function is given by a difficult nonconvex optimization problem. We develop approximations based on nonconvex projected gradient descent and demonstrate the resulting bounds empirically.
Visit talk page
https://simons.berkeley.edu/talks/confounding-robust-policy-evaluation-infinite-horizon-reinforcement-learning
Algorithmic Aspects of Causal Inference
Off-policy evaluation of sequential decision policies from observational data is necessary in applications of batch reinforcement learning such as education and healthcare. In such settings, however, unobserved variables confound observed actions, rendering exact evaluation of new policies impossible, i.e., unidentifiable. We develop a robust approach that estimates sharp bounds on the (unidentifiable) value of a given policy in an infinite-horizon problem given data from another policy with unobserved confounding, subject to a sensitivity model. We consider stationary or baseline unobserved confounding and compute bounds by optimizing over the set of all stationary state-occupancy ratios that agree with a new partially identified estimating equation and the sensitivity model. We prove convergence to the sharp bounds as we collect more confounded data. Although checking set membership is a linear program, the support function is given by a difficult nonconvex optimization problem. We develop approximations based on nonconvex projected gradient descent and demonstrate the resulting bounds empirically.
0:29:21
Piyush Srivastava (Tata Institute of Fundamental Research)
https://simons.berkeley.edu/talks/stability-causal-identification-perspective-condition-numbers
Algorithmic Aspects of Causal Inference
An important achievement in the field of causal inference was a complete characterization of when a causal effect, in a system modeled by a causal graph, can be determined uniquely from purely observational data. The identification algorithms resulting from this work produce exact symbolic expressions for causal effects, in terms of the observational probabilities. This talk will focus on the numerical properties of these expressions, in particular using the classical notion of the condition number. In its classical interpretation, the condition number quantifies the sensitivity of the output values of these expressions to small numerical perturbations in the input observational probabilities. In the context of the causal identification, we will discuss how the condition number is related not just to stability against numerical uncertainty, but also to stability against certain kinds of uncertainties in the *structure* of the model. We then give upper bounds on the condition number of causal identification for some special cases, including in particular the case of causal graphical models with small "confounded components". Using a tight characterization of this condition number, we then show that even "equivalent" formulas for causal identification can behave very differently with respect to their numerical stability properties. This suggests that this characterization of the condition number may be useful in choosing between "equivalent" causal identification expressions. Joint work with Spencer Gordon, Vinayak Kumar and Leonard J. Schulman.
Visit talk page
https://simons.berkeley.edu/talks/stability-causal-identification-perspective-condition-numbers
Algorithmic Aspects of Causal Inference
An important achievement in the field of causal inference was a complete characterization of when a causal effect, in a system modeled by a causal graph, can be determined uniquely from purely observational data. The identification algorithms resulting from this work produce exact symbolic expressions for causal effects, in terms of the observational probabilities. This talk will focus on the numerical properties of these expressions, in particular using the classical notion of the condition number. In its classical interpretation, the condition number quantifies the sensitivity of the output values of these expressions to small numerical perturbations in the input observational probabilities. In the context of the causal identification, we will discuss how the condition number is related not just to stability against numerical uncertainty, but also to stability against certain kinds of uncertainties in the *structure* of the model. We then give upper bounds on the condition number of causal identification for some special cases, including in particular the case of causal graphical models with small "confounded components". Using a tight characterization of this condition number, we then show that even "equivalent" formulas for causal identification can behave very differently with respect to their numerical stability properties. This suggests that this characterization of the condition number may be useful in choosing between "equivalent" causal identification expressions. Joint work with Spencer Gordon, Vinayak Kumar and Leonard J. Schulman.
0:31:26
Devavrat Shah (MIT)
https://simons.berkeley.edu/talks/causalsim-trace-driven-simulation-network-protocols
Algorithmic Aspects of Causal Inference
Evaluating the real-world performance of network protocols is challenging. Randomized control trials (RCT) are expensive and inaccessible to most, while simulators fail to capture complex behaviors in real networks. In this talk, we introduce CausalSim, a trace-driven counterfactual simulator for network protocols that addresses this challenge. Counterfactual simulation aims to predict what would happen using different protocols under the same conditions as a given trace. This is complicated due to the bias introduced by the protocols used during trace collection. CausalSim uses traces from an initial RCT under a set of protocols to learn a causal network model, effectively removing the biases present in the data. Key to CausalSim is mapping the task of counterfactual simulation to that of tensor completion with extremely sparse observations. Through an adversarial neural network training technique that exploits distributional invariances that are present in training data coming from an RCT, CausalSim enables a novel tensor completion method despite the sparsity of observations. We will discuss empirical evaluation of the CausalSim.
Visit talk page
https://simons.berkeley.edu/talks/causalsim-trace-driven-simulation-network-protocols
Algorithmic Aspects of Causal Inference
Evaluating the real-world performance of network protocols is challenging. Randomized control trials (RCT) are expensive and inaccessible to most, while simulators fail to capture complex behaviors in real networks. In this talk, we introduce CausalSim, a trace-driven counterfactual simulator for network protocols that addresses this challenge. Counterfactual simulation aims to predict what would happen using different protocols under the same conditions as a given trace. This is complicated due to the bias introduced by the protocols used during trace collection. CausalSim uses traces from an initial RCT under a set of protocols to learn a causal network model, effectively removing the biases present in the data. Key to CausalSim is mapping the task of counterfactual simulation to that of tensor completion with extremely sparse observations. Through an adversarial neural network training technique that exploits distributional invariances that are present in training data coming from an RCT, CausalSim enables a novel tensor completion method despite the sparsity of observations. We will discuss empirical evaluation of the CausalSim.
0:50:25
Breakthroughs
Guy Rothblum (Weizmann Institute of Science)
https://simons.berkeley.edu/talks/multi-group-approach-algorithmic-fairness
Algorithmic Aspects of Causal Inference
As algorithms increasingly inform and influence decisions made about individuals, it becomes increasingly important to address concerns that these algorithms might be discriminatory. We develop and study multi-group fairness, a new approach to algorithmic fairness that aims to provide fairness guarantees for every subpopulation in a rich class of overlapping subgroups. We focus on guarantees that are aligned with obtaining predictions that are accurate w.r.t. the training data, such as subgroup calibration or subgroup loss-minimization. We present new algorithms for learning multi-group fair predictors, study the computational complexity of this task, and draw connections to the theory of agnostic learning.
Visit talk page
https://simons.berkeley.edu/talks/multi-group-approach-algorithmic-fairness
Algorithmic Aspects of Causal Inference
As algorithms increasingly inform and influence decisions made about individuals, it becomes increasingly important to address concerns that these algorithms might be discriminatory. We develop and study multi-group fairness, a new approach to algorithmic fairness that aims to provide fairness guarantees for every subpopulation in a rich class of overlapping subgroups. We focus on guarantees that are aligned with obtaining predictions that are accurate w.r.t. the training data, such as subgroup calibration or subgroup loss-minimization. We present new algorithms for learning multi-group fair predictors, study the computational complexity of this task, and draw connections to the theory of agnostic learning.
0:40:21
Gal Yona (Weizmann Institute)
https://simons.berkeley.edu/talks/decision-making-under-miscalibration
Algorithmic Aspects of Causal Inference
ML-based predictions are used to inform consequential decisions about individuals. How should we use predictions (e.g., risk of heart attack) to inform downstream binary classification decisions (e.g., undergoing a medical procedure)? When the risk estimates are perfectly calibrated, the answer is well understood: a classification problem’s cost structure induces an optimal treatment threshold. In practice, however, some amount of miscalibration is unavoidable, raising a fundamental question: how should one use potentially miscalibrated predictions to inform binary decisions? We formalize a natural (distribution-free) solution concept: given a level of anticipated miscalibration $\alpha$, we propose using the threshold that minimizes the worst-case regret over all $\alpha$-miscalibrated predictors, where the regret is the difference in clinical utility between using the threshold in question and using the optimal threshold in hindsight. We provide closed form expressions for the regret minimizing threshold when miscalibration is measured using both expected and maximum calibration error, which reveal that it indeed differs from the optimal threshold under perfect calibration, and validate our theoretical findings on real data.
Visit talk page
https://simons.berkeley.edu/talks/decision-making-under-miscalibration
Algorithmic Aspects of Causal Inference
ML-based predictions are used to inform consequential decisions about individuals. How should we use predictions (e.g., risk of heart attack) to inform downstream binary classification decisions (e.g., undergoing a medical procedure)? When the risk estimates are perfectly calibrated, the answer is well understood: a classification problem’s cost structure induces an optimal treatment threshold. In practice, however, some amount of miscalibration is unavoidable, raising a fundamental question: how should one use potentially miscalibrated predictions to inform binary decisions? We formalize a natural (distribution-free) solution concept: given a level of anticipated miscalibration $\alpha$, we propose using the threshold that minimizes the worst-case regret over all $\alpha$-miscalibrated predictors, where the regret is the difference in clinical utility between using the threshold in question and using the optimal threshold in hindsight. We provide closed form expressions for the regret minimizing threshold when miscalibration is measured using both expected and maximum calibration error, which reveal that it indeed differs from the optimal threshold under perfect calibration, and validate our theoretical findings on real data.
0:45:26
Omer Reingold (Stanford University)
https://simons.berkeley.edu/talks/tbd-396
Algorithmic Aspects of Causal Inference
A key challenge in modern statistics is to ensure statistically-valid inferences across diverse target populations from a fixed source of training data. Statistical techniques that guarantee this type of adaptability not only make statistical conclusions more robust to sampling bias, but can also extend the benefits of evidence-based decision-making to communities that do not have the resources to collect high-quality data or computationally-intensive estimation on their own. In this talk, we describe a surprising technical connection between the statistical inference problem and multicalibration, a technique developed in the context of algorithmic fairness. Exploiting this connection, we derive a single-source estimator that provides inferences that are *universally-adaptable* to any downstream target population. The performance of the estimator is comparable to the performance of propensity score reweighting, a widely-used technique that explicitly models the underlying source-target shift, *for every target*.
We will discuss universal adaptability for prediction tasks, and its extensions to treatment effect. Finally, we will speculate on possible connections between multicalibration and causality.
Mostly based on joint work with Michael Kim, Christoph Kern, Shafi Goldwasser and Frauke Kreuter.
Visit talk page
https://simons.berkeley.edu/talks/tbd-396
Algorithmic Aspects of Causal Inference
A key challenge in modern statistics is to ensure statistically-valid inferences across diverse target populations from a fixed source of training data. Statistical techniques that guarantee this type of adaptability not only make statistical conclusions more robust to sampling bias, but can also extend the benefits of evidence-based decision-making to communities that do not have the resources to collect high-quality data or computationally-intensive estimation on their own. In this talk, we describe a surprising technical connection between the statistical inference problem and multicalibration, a technique developed in the context of algorithmic fairness. Exploiting this connection, we derive a single-source estimator that provides inferences that are *universally-adaptable* to any downstream target population. The performance of the estimator is comparable to the performance of propensity score reweighting, a widely-used technique that explicitly models the underlying source-target shift, *for every target*.
We will discuss universal adaptability for prediction tasks, and its extensions to treatment effect. Finally, we will speculate on possible connections between multicalibration and causality.
Mostly based on joint work with Michael Kim, Christoph Kern, Shafi Goldwasser and Frauke Kreuter.
Sanghamitra Dutta (JP Morgan AI Research)
https://simons.berkeley.edu/talks/algorithmic-fairness-lens-causality-and-information-theory
Algorithmic Aspects of Causal Inference
When it comes to resolving legal disputes or even informing policies and interventions, only identifying bias/disparity in a model's decision is insufficient. We really need to dig deeper and understand how it arose. E.g., disparities in hiring that can be explained by an occupational necessity (code-writing skills for software engineering) may be exempt by law, but the disparity arising due to an aptitude test may not be (Ref: Griggs v. Duke Power `71). This leads us to a question that bridges the fields of fairness, explainability, and law: How can we identify and explain the sources of disparity in ML models, e.g., did the disparity entirely arise due to the critical occupational necessities? In this talk, I propose a systematic measure of "non-exempt disparity," i.e., the bias which cannot be explained by the occupational necessities. To arrive at a measure for the non-exempt disparity, I adopt a rigorous axiomatic approach that brings together concepts in information theory (in particular, an emerging body of work called Partial Information Decomposition) with causality. In the second part of the talk, I will also discuss another recent work: the quantification of accuracy-fairness trade-offs using another tool from information theory, namely Chernoff Information. Based on interest, I can spend more time on either the first or the second work.
Visit talk page
https://simons.berkeley.edu/talks/algorithmic-fairness-lens-causality-and-information-theory
Algorithmic Aspects of Causal Inference
When it comes to resolving legal disputes or even informing policies and interventions, only identifying bias/disparity in a model's decision is insufficient. We really need to dig deeper and understand how it arose. E.g., disparities in hiring that can be explained by an occupational necessity (code-writing skills for software engineering) may be exempt by law, but the disparity arising due to an aptitude test may not be (Ref: Griggs v. Duke Power `71). This leads us to a question that bridges the fields of fairness, explainability, and law: How can we identify and explain the sources of disparity in ML models, e.g., did the disparity entirely arise due to the critical occupational necessities? In this talk, I propose a systematic measure of "non-exempt disparity," i.e., the bias which cannot be explained by the occupational necessities. To arrive at a measure for the non-exempt disparity, I adopt a rigorous axiomatic approach that brings together concepts in information theory (in particular, an emerging body of work called Partial Information Decomposition) with causality. In the second part of the talk, I will also discuss another recent work: the quantification of accuracy-fairness trade-offs using another tool from information theory, namely Chernoff Information. Based on interest, I can spend more time on either the first or the second work.
0:45:21
Theoretically Speaking
Vasilis Syrgkanis (Microsoft Research)
https://simons.berkeley.edu/talks/orthogonal-statistical-learning
Algorithmic Aspects of Causal Inference
We provide non-asymptotic excess risk guarantees for statistical learning in a setting where the population risk with respect to which we evaluate the target parameter depends on an unknown nuisance parameter that must be estimated from data. We analyze a two-stage sample splitting meta-algorithm that takes as input two arbitrary estimation algorithms: one for the target parameter and one for the nuisance parameter. We show that if the population risk satisfies a condition called Neyman orthogonality, the impact of the nuisance estimation error on the excess risk bound achieved by the meta-algorithm is of second order. Our theorem is agnostic to the particular algorithms used for the target and nuisance and only makes an assumption on their individual performance. This enables the use of a plethora of existing results from statistical learning and machine learning to give new guarantees for learning with a nuisance component. Moreover, by focusing on excess risk rather than parameter estimation, we can give guarantees under weaker assumptions than in previous works and accommodate settings in which the target parameter belongs to a complex nonparametric class. We provide conditions on the metric entropy of the nuisance and target classes such that oracle rates---rates of the same order as if we knew the nuisance parameter---are achieved. We also derive new rates for specific estimation algorithms such as variance-penalized empirical risk minimization, neural network estimation and sparse high-dimensional linear model estimation. We highlight the applicability of our results in four settings of central importance: 1) heterogeneous treatment effect estimation, 2) offline policy optimization, 3) domain adaptation, and 4) learning with missing data.
Visit talk page
https://simons.berkeley.edu/talks/orthogonal-statistical-learning
Algorithmic Aspects of Causal Inference
We provide non-asymptotic excess risk guarantees for statistical learning in a setting where the population risk with respect to which we evaluate the target parameter depends on an unknown nuisance parameter that must be estimated from data. We analyze a two-stage sample splitting meta-algorithm that takes as input two arbitrary estimation algorithms: one for the target parameter and one for the nuisance parameter. We show that if the population risk satisfies a condition called Neyman orthogonality, the impact of the nuisance estimation error on the excess risk bound achieved by the meta-algorithm is of second order. Our theorem is agnostic to the particular algorithms used for the target and nuisance and only makes an assumption on their individual performance. This enables the use of a plethora of existing results from statistical learning and machine learning to give new guarantees for learning with a nuisance component. Moreover, by focusing on excess risk rather than parameter estimation, we can give guarantees under weaker assumptions than in previous works and accommodate settings in which the target parameter belongs to a complex nonparametric class. We provide conditions on the metric entropy of the nuisance and target classes such that oracle rates---rates of the same order as if we knew the nuisance parameter---are achieved. We also derive new rates for specific estimation algorithms such as variance-penalized empirical risk minimization, neural network estimation and sparse high-dimensional linear model estimation. We highlight the applicability of our results in four settings of central importance: 1) heterogeneous treatment effect estimation, 2) offline policy optimization, 3) domain adaptation, and 4) learning with missing data.
1:27:36
Theoretically Speaking
Noa Dagan (Harvard, Clalit Research Institute and Ben-Gurion University)
Noam Barda (Harvard, Tel-HaShomer Medical Center and Ben-Gurion University)
https://simons.berkeley.edu/talks/theoretically-speaking-opportunities-application-quantitative-models-fully-integrated
Theoretically Speaking
Health insurance in Israel is mandatory, comprehensive in its list of services, and provided by four integrated payer-provider organizations. Clalit Health Services is the largest of these organizations — responsible for the care of over half of the Israeli population. Most of this care (outpatient and inpatient) is directly provided by Clalit, and the rest is purchased by Clalit. All services provided or purchased are stored in a single comprehensive analytic data warehouse. This talk will focus on the opportunities that such an integrated system and its data offer in using quantitative models for state-of-the-art research and digital health care interventions.
Noam Barda and Noa Dagan will discuss the two main quantitative tools used for digital health care — causal inference and prediction models. They will show how the depth and immediacy of the data allowed for causal research that provided necessary and timely information regarding the effectiveness and safety of mRNA COVID-19 vaccines. They will also show how such unique data enabled them to study an often-overlooked aspect of vaccination — indirect protective effects. They will also demonstrate how this data can be used for promoting predictive, proactive, and personalized care. They will demonstrate how prediction models are created and how they are integrated into the point of care.
Noam Barda holds an MD from Tel Aviv University, a PhD in public health and computer science from Ben-Gurion University, and a BSc in computer science from the Open University. He completed his postdoctorate in the Department of Biomedical Informatics (DBMI) at Harvard Medical School. He is the head of the Real-World Evidence Research and Innovation Lab at Tel HaShomer medical center, Israel’s largest hospital, and co-heads the Digital Healthcare Laboratory in the Department of Software and Information Systems Engineering at Ben-Gurion University.
Noa Dagan holds an MD and an MPH from the Hebrew University, and a PhD in computer science from Ben-Gurion University. She completed her postdoctorate in the Department of Biomedical Informatics (DBMI) at Harvard Medical School. She is the director of the AI-Driven Medicine Department at Clalit Innovation and the Clalit Research Institute, and co-heads the Digital Healthcare Laboratory in the Department of Software and Information Systems Engineering at Ben-Gurion University.
Visit talk page
Noam Barda (Harvard, Tel-HaShomer Medical Center and Ben-Gurion University)
https://simons.berkeley.edu/talks/theoretically-speaking-opportunities-application-quantitative-models-fully-integrated
Theoretically Speaking
Health insurance in Israel is mandatory, comprehensive in its list of services, and provided by four integrated payer-provider organizations. Clalit Health Services is the largest of these organizations — responsible for the care of over half of the Israeli population. Most of this care (outpatient and inpatient) is directly provided by Clalit, and the rest is purchased by Clalit. All services provided or purchased are stored in a single comprehensive analytic data warehouse. This talk will focus on the opportunities that such an integrated system and its data offer in using quantitative models for state-of-the-art research and digital health care interventions.
Noam Barda and Noa Dagan will discuss the two main quantitative tools used for digital health care — causal inference and prediction models. They will show how the depth and immediacy of the data allowed for causal research that provided necessary and timely information regarding the effectiveness and safety of mRNA COVID-19 vaccines. They will also show how such unique data enabled them to study an often-overlooked aspect of vaccination — indirect protective effects. They will also demonstrate how this data can be used for promoting predictive, proactive, and personalized care. They will demonstrate how prediction models are created and how they are integrated into the point of care.
Noam Barda holds an MD from Tel Aviv University, a PhD in public health and computer science from Ben-Gurion University, and a BSc in computer science from the Open University. He completed his postdoctorate in the Department of Biomedical Informatics (DBMI) at Harvard Medical School. He is the head of the Real-World Evidence Research and Innovation Lab at Tel HaShomer medical center, Israel’s largest hospital, and co-heads the Digital Healthcare Laboratory in the Department of Software and Information Systems Engineering at Ben-Gurion University.
Noa Dagan holds an MD and an MPH from the Hebrew University, and a PhD in computer science from Ben-Gurion University. She completed her postdoctorate in the Department of Biomedical Informatics (DBMI) at Harvard Medical School. She is the director of the AI-Driven Medicine Department at Clalit Innovation and the Clalit Research Institute, and co-heads the Digital Healthcare Laboratory in the Department of Software and Information Systems Engineering at Ben-Gurion University.
0:47:56
Theory Shorts
Batya Kenig (Technion, Israel Institute of Technology)
https://simons.berkeley.edu/talks/approximate-implication-problem-probabilistic-graphical-models
Algorithmic Aspects of Causal Inference
The implication problem studies whether a set of conditional independence (CI) statements (antecedents) implies another CI (consequent), and has been extensively studied in the AI literature, under the assumption that all CIs hold exactly. A common example of implication is the well-known d-separation algorithm that infers conditional independence relations based on a ground set of CIs used to construct the graphical structure. However, many applications today need to consider CIs that hold only approximately. We define an approximate implication as a linear inequality between the degree of satisfaction of the antecedents and consequent, and we study the relaxation problem: when does an exact implication relax to an approximate implication? More precisely, what guarantee can we provide on the inferred CI when the set of CIs that entailed it hold only approximately? We use information theory to define the degree of satisfaction, and prove several results. In the general case, no such guarantee can be provided. We prove that such a guarantee exists for the set of CIs inferred in directed graphical models, making the d-separation algorithm a sound and complete system for inferring approximate CIs. We also prove an approximation guarantee for independence relations derived from marginal and saturated CIs. Next, we show how information-theoretic inequalities can be applied to the task of learning approximate decomposable models from observations.
Visit talk page
https://simons.berkeley.edu/talks/approximate-implication-problem-probabilistic-graphical-models
Algorithmic Aspects of Causal Inference
The implication problem studies whether a set of conditional independence (CI) statements (antecedents) implies another CI (consequent), and has been extensively studied in the AI literature, under the assumption that all CIs hold exactly. A common example of implication is the well-known d-separation algorithm that infers conditional independence relations based on a ground set of CIs used to construct the graphical structure. However, many applications today need to consider CIs that hold only approximately. We define an approximate implication as a linear inequality between the degree of satisfaction of the antecedents and consequent, and we study the relaxation problem: when does an exact implication relax to an approximate implication? More precisely, what guarantee can we provide on the inferred CI when the set of CIs that entailed it hold only approximately? We use information theory to define the degree of satisfaction, and prove several results. In the general case, no such guarantee can be provided. We prove that such a guarantee exists for the set of CIs inferred in directed graphical models, making the d-separation algorithm a sound and complete system for inferring approximate CIs. We also prove an approximation guarantee for independence relations derived from marginal and saturated CIs. Next, we show how information-theoretic inequalities can be applied to the task of learning approximate decomposable models from observations.