Image
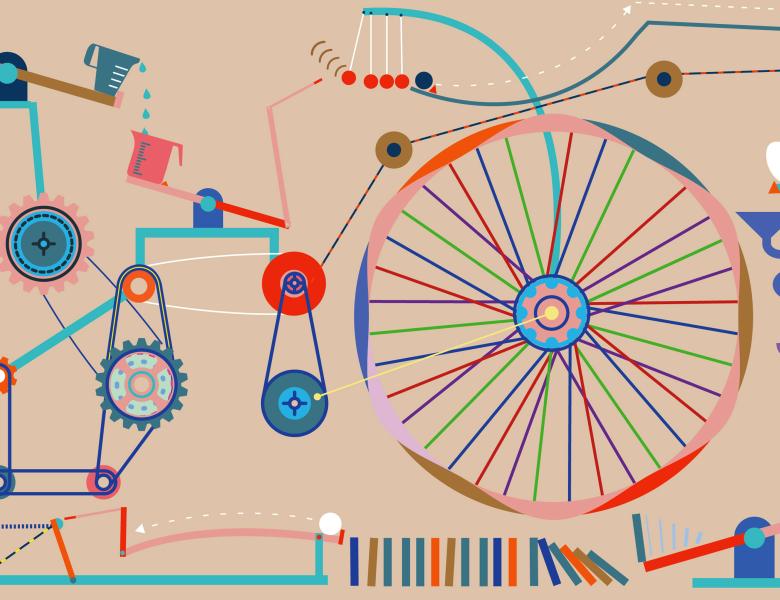
As algorithms increasingly inform and influence decisions made about individuals, it becomes increasingly important to address concerns that these algorithms might be discriminatory. We develop and study multi-group fairness, a new approach to algorithmic fairness that aims to provide fairness guarantees for every subpopulation in a rich class of overlapping subgroups. We focus on guarantees that are aligned with obtaining predictions that are accurate w.r.t. the training data, such as subgroup calibration or subgroup loss-minimization. We present new algorithms for learning multi-group fair predictors, study the computational complexity of this task, and draw connections to the theory of agnostic learning.