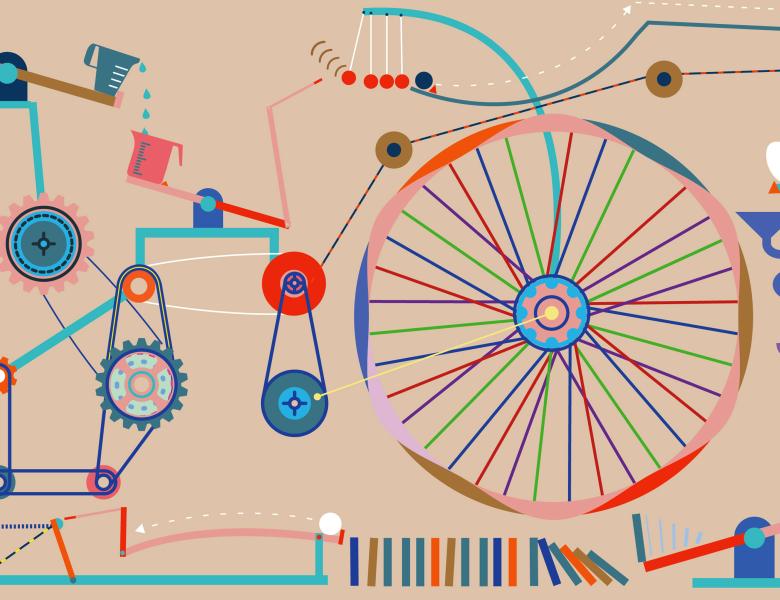
Abstract
When it comes to resolving legal disputes or even informing policies and interventions, only identifying bias/disparity in a model's decision is insufficient. We really need to dig deeper and understand how it arose. E.g., disparities in hiring that can be explained by an occupational necessity (code-writing skills for software engineering) may be exempt by law, but the disparity arising due to an aptitude test may not be (Ref: Griggs v. Duke Power `71). This leads us to a question that bridges the fields of fairness, explainability, and law: How can we identify and explain the sources of disparity in ML models, e.g., did the disparity entirely arise due to the critical occupational necessities? In this talk, I propose a systematic measure of "non-exempt disparity," i.e., the bias which cannot be explained by the occupational necessities. To arrive at a measure for the non-exempt disparity, I adopt a rigorous axiomatic approach that brings together concepts in information theory (in particular, an emerging body of work called Partial Information Decomposition) with causality. In the second part of the talk, I will also discuss another recent work: the quantification of accuracy-fairness trade-offs using another tool from information theory, namely Chernoff Information. Based on interest, I can spend more time on either the first or the second work.