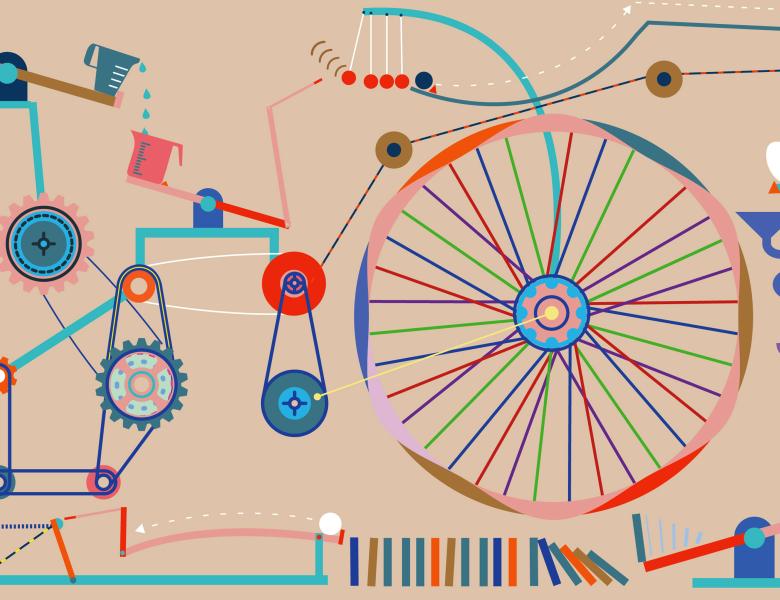
Abstract
An important achievement in the field of causal inference was a complete characterization of when a causal effect, in a system modeled by a causal graph, can be determined uniquely from purely observational data. The identification algorithms resulting from this work produce exact symbolic expressions for causal effects, in terms of the observational probabilities. This talk will focus on the numerical properties of these expressions, in particular using the classical notion of the condition number. In its classical interpretation, the condition number quantifies the sensitivity of the output values of these expressions to small numerical perturbations in the input observational probabilities. In the context of the causal identification, we will discuss how the condition number is related not just to stability against numerical uncertainty, but also to stability against certain kinds of uncertainties in the *structure* of the model. We then give upper bounds on the condition number of causal identification for some special cases, including in particular the case of causal graphical models with small "confounded components". Using a tight characterization of this condition number, we then show that even "equivalent" formulas for causal identification can behave very differently with respect to their numerical stability properties. This suggests that this characterization of the condition number may be useful in choosing between "equivalent" causal identification expressions. Joint work with Spencer Gordon, Vinayak Kumar and Leonard J. Schulman.