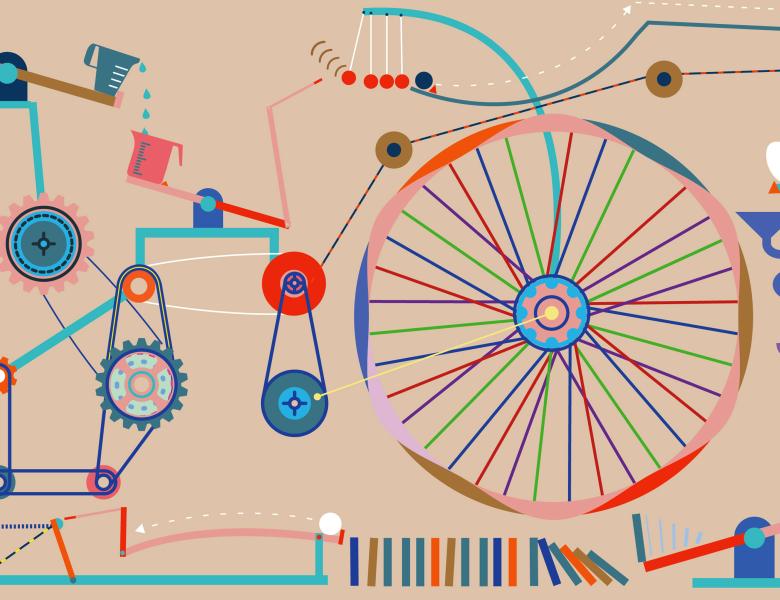
Playlist: 25 videos
Algorithmic Aspects of Causal Inference
0:47:35
Moritz Hardt (Max Planck Institute for Intelligent Systems)
https://simons.berkeley.edu/talks/challenges-causal-inference-digital-platforms
Algorithmic Aspects of Causal Inference
In this talk, I'll discuss a couple of recent efforts that apply causal inference to study the effects of algorithmic interventions on digital platforms, specifically, the Twitter platform. The first work is an extensive audit of the algorithmic amplification of political content on Twitter based on a long-running, massive-scale randomized experiment. I'll focus on what the study design shows and what it cannot show. While some challenges, such as network effects and interference, are widely recognized, others are more subtle. The second work is about the difficulty of making causal inferences based on user choices on the platform. While user choices gives rise to a host of intriguing observational designs, I'll explain why these may be inherently difficult to deconfound. Based on these two studies, I'll discuss some shortcomings of causal inference in practice and avenues for future work. The first part is joint work with Ferenc Huszár, Sofia Ira Ktena, Conor O’Brien, Luca Belli, and Andrew Schlaikjer. The second is joint work with Smitha Milli and Luca Belli.
Visit talk page
https://simons.berkeley.edu/talks/challenges-causal-inference-digital-platforms
Algorithmic Aspects of Causal Inference
In this talk, I'll discuss a couple of recent efforts that apply causal inference to study the effects of algorithmic interventions on digital platforms, specifically, the Twitter platform. The first work is an extensive audit of the algorithmic amplification of political content on Twitter based on a long-running, massive-scale randomized experiment. I'll focus on what the study design shows and what it cannot show. While some challenges, such as network effects and interference, are widely recognized, others are more subtle. The second work is about the difficulty of making causal inferences based on user choices on the platform. While user choices gives rise to a host of intriguing observational designs, I'll explain why these may be inherently difficult to deconfound. Based on these two studies, I'll discuss some shortcomings of causal inference in practice and avenues for future work. The first part is joint work with Ferenc Huszár, Sofia Ira Ktena, Conor O’Brien, Luca Belli, and Andrew Schlaikjer. The second is joint work with Smitha Milli and Luca Belli.
0:39:40
Christina Heinze-Deml (Apple Health AI)
https://simons.berkeley.edu/talks/active-invariant-causal-prediction-experiment-selection-through-stability
Algorithmic Aspects of Causal Inference
Causal inference plays a key role in many scientific disciplines. Often the underlying causal graph is unknown and needs to be estimated from data. In this context, one fundamental difficulty is that causal models can generally not be fully identified based on observational data only. Interventional data, that is, data originating from different experimental environments, improves identifiability. By exploiting the invariance of the causal model the method “Invariant Causal Prediction” (Peters et al., 2016) exploits data from different environments to estimate the causal parents of a response variable of interest. However, the improvement in identifiability compared to the observational setting depends critically on the target and nature of the interventions carried out in each experiment. Since in real applications experiments tend to be costly, there is a need to perform the right interventions such that as few as possible are required. In this talk, I will present new active learning (i.e. experiment selection) strategies based on causal invariance, which select intervention targets that quickly reveal the direct causes of a response variable of interest in the causal graph.
Visit talk page
https://simons.berkeley.edu/talks/active-invariant-causal-prediction-experiment-selection-through-stability
Algorithmic Aspects of Causal Inference
Causal inference plays a key role in many scientific disciplines. Often the underlying causal graph is unknown and needs to be estimated from data. In this context, one fundamental difficulty is that causal models can generally not be fully identified based on observational data only. Interventional data, that is, data originating from different experimental environments, improves identifiability. By exploiting the invariance of the causal model the method “Invariant Causal Prediction” (Peters et al., 2016) exploits data from different environments to estimate the causal parents of a response variable of interest. However, the improvement in identifiability compared to the observational setting depends critically on the target and nature of the interventions carried out in each experiment. Since in real applications experiments tend to be costly, there is a need to perform the right interventions such that as few as possible are required. In this talk, I will present new active learning (i.e. experiment selection) strategies based on causal invariance, which select intervention targets that quickly reveal the direct causes of a response variable of interest in the causal graph.
0:33:6
Christina Yu (Cornell University)
https://simons.berkeley.edu/talks/simple-yet-efficient-estimators-network-causal-inference-beyond-linear-low-degree-polynomials
Algorithmic Aspects of Causal Inference
Classical approaches to experimental design rely on critical independence assumptions that are violated when the outcome of an individual i may be affected by the treatment of another individual j, referred to as network interference. This interference introduces computational and statistical challenges to causal inference. We present a new hierarchy of models and estimators that enable statistically efficient and computationally simple solutions under nonparametric polynomial models, with theoretical guarantees even in settings without graph knowledge, or under model misspecification.
Visit talk page
https://simons.berkeley.edu/talks/simple-yet-efficient-estimators-network-causal-inference-beyond-linear-low-degree-polynomials
Algorithmic Aspects of Causal Inference
Classical approaches to experimental design rely on critical independence assumptions that are violated when the outcome of an individual i may be affected by the treatment of another individual j, referred to as network interference. This interference introduces computational and statistical challenges to causal inference. We present a new hierarchy of models and estimators that enable statistically efficient and computationally simple solutions under nonparametric polynomial models, with theoretical guarantees even in settings without graph knowledge, or under model misspecification.
0:31:21
Shiva Kasiviswanathan (Amazon)
https://simons.berkeley.edu/talks/collaborative-causal-discovery-atomic-interventions
Algorithmic Aspects of Causal Inference
In this talk, we will introduce a Collaborative Causal Discovery problem to model a common scenario in which we have multiple independent entities each with their own causal graph, and the goal is to simultaneously learn all these causal graphs. We study this problem without the causal sufficiency assumption, using Maximal Ancestral Graphs (MAG) to model the causal graphs, and assuming that we have the ability to actively perform independent single vertex (or atomic) interventions on the entities. If the M underlying (unknown) causal graphs of the entities satisfy a natural notion of clustering, we give algorithms that leverage this property, and recovers all the causal graphs using roughly logarithmic in M number of graphs atomic interventions per entity. We complement our results with a lower bound and discuss various extensions of our collaborative setting. Ideas from randomized algorithms play an important role in both our upper and lower bounds. Joint work with Raghavendra Addanki.
Visit talk page
https://simons.berkeley.edu/talks/collaborative-causal-discovery-atomic-interventions
Algorithmic Aspects of Causal Inference
In this talk, we will introduce a Collaborative Causal Discovery problem to model a common scenario in which we have multiple independent entities each with their own causal graph, and the goal is to simultaneously learn all these causal graphs. We study this problem without the causal sufficiency assumption, using Maximal Ancestral Graphs (MAG) to model the causal graphs, and assuming that we have the ability to actively perform independent single vertex (or atomic) interventions on the entities. If the M underlying (unknown) causal graphs of the entities satisfy a natural notion of clustering, we give algorithms that leverage this property, and recovers all the causal graphs using roughly logarithmic in M number of graphs atomic interventions per entity. We complement our results with a lower bound and discuss various extensions of our collaborative setting. Ideas from randomized algorithms play an important role in both our upper and lower bounds. Joint work with Raghavendra Addanki.
0:32:15
Bryon Aragam (University of Chicago)
https://simons.berkeley.edu/talks/new-approaches-learning-nonparametric-latent-causal-graphical-models
Algorithmic Aspects of Causal Inference
Interpretability and causality have been acknowledged as key ingredients to the success and evolution of modern machine learning systems. Graphical models, and more specifically directed acyclic graphs (DAGs, also known as Bayesian networks), are an established tool for learning and representing interpretable causal models. Unfortunately, estimating the structure of DAGs from data is a notoriously difficult problem, coming with a host of identifiability issues. We will discuss our recent work towards overcoming these challenges in distribution-free and nonparametric models. Starting with the fully observed case, we will discuss how known identifiability results in the linear Gaussian case can be generalized to nonparametric models. We will then discuss more difficult problems involving latent variables, and show how to identify latent causal graphs without linear or parametric assumptions. In both cases, the theory leads to efficient, polynomial time algorithms that are easily implemented in practice.
Visit talk page
https://simons.berkeley.edu/talks/new-approaches-learning-nonparametric-latent-causal-graphical-models
Algorithmic Aspects of Causal Inference
Interpretability and causality have been acknowledged as key ingredients to the success and evolution of modern machine learning systems. Graphical models, and more specifically directed acyclic graphs (DAGs, also known as Bayesian networks), are an established tool for learning and representing interpretable causal models. Unfortunately, estimating the structure of DAGs from data is a notoriously difficult problem, coming with a host of identifiability issues. We will discuss our recent work towards overcoming these challenges in distribution-free and nonparametric models. Starting with the fully observed case, we will discuss how known identifiability results in the linear Gaussian case can be generalized to nonparametric models. We will then discuss more difficult problems involving latent variables, and show how to identify latent causal graphs without linear or parametric assumptions. In both cases, the theory leads to efficient, polynomial time algorithms that are easily implemented in practice.
0:29:36
Rahul Singh (MIT)
https://simons.berkeley.edu/talks/kernel-methods-causal-inference
Algorithmic Aspects of Causal Inference
We propose a family of estimators based on kernel ridge regression for nonparametric dose response curves and semiparametric treatment effects. Treatment and covariates may be discrete or continuous, and low, high, or infinite dimensional. We reduce causal estimation and inference to combinations of kernel ridge regressions, which have closed form solutions and are easily computed by matrix operations, unlike other machine learning paradigms. This computational simplicity allows us to extend the framework to several settings: (i) heterogeneous effects and distribution shift; (ii) instruments and proxies; (iii) mediation and dynamic effects; (iv) sample selection and data fusion. For dose responses, we prove uniform consistency with finite sample rates. For treatment effects, we prove root-n consistency, Gaussian approximation, and semiparametric efficiency with a new double spectral robustness property.
Visit talk page
https://simons.berkeley.edu/talks/kernel-methods-causal-inference
Algorithmic Aspects of Causal Inference
We propose a family of estimators based on kernel ridge regression for nonparametric dose response curves and semiparametric treatment effects. Treatment and covariates may be discrete or continuous, and low, high, or infinite dimensional. We reduce causal estimation and inference to combinations of kernel ridge regressions, which have closed form solutions and are easily computed by matrix operations, unlike other machine learning paradigms. This computational simplicity allows us to extend the framework to several settings: (i) heterogeneous effects and distribution shift; (ii) instruments and proxies; (iii) mediation and dynamic effects; (iv) sample selection and data fusion. For dose responses, we prove uniform consistency with finite sample rates. For treatment effects, we prove root-n consistency, Gaussian approximation, and semiparametric efficiency with a new double spectral robustness property.