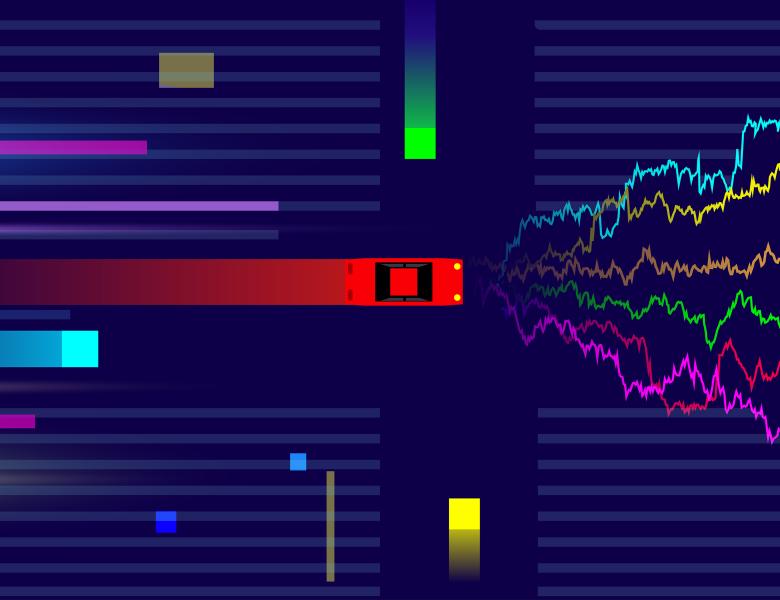
Playlist: 27 videos
Quantifying Uncertainty: Stochastic, Adversarial, and Beyond
0:54:21
Annie Liang (Northwestern University)
https://simons.berkeley.edu/talks/tbd-464
Quantifying Uncertainty: Stochastic, Adversarial, and Beyond
An agent has access to multiple information sources, each modeled as a Brownian motion whose drift provides information about a different component of an unknown Gaussian state. Information is acquired continuously—where the agent chooses both which sources to sample from, and also how to allocate attention across them—until an endogenously chosen time, at which point a decision is taken. We demonstrate conditions on the agent’s prior belief under which it is possible to exactly characterize the optimal information acquisition strategy. We then apply this characterization to derive new results regarding: (1) endogenous information acquisition for binary choice, (2) the dynamic consequences of attention manipulation, and (3) strategic information provision by biased news sources.
Visit talk page
https://simons.berkeley.edu/talks/tbd-464
Quantifying Uncertainty: Stochastic, Adversarial, and Beyond
An agent has access to multiple information sources, each modeled as a Brownian motion whose drift provides information about a different component of an unknown Gaussian state. Information is acquired continuously—where the agent chooses both which sources to sample from, and also how to allocate attention across them—until an endogenously chosen time, at which point a decision is taken. We demonstrate conditions on the agent’s prior belief under which it is possible to exactly characterize the optimal information acquisition strategy. We then apply this characterization to derive new results regarding: (1) endogenous information acquisition for binary choice, (2) the dynamic consequences of attention manipulation, and (3) strategic information provision by biased news sources.
0:43:55
Can Urgun (Princeton University)
https://simons.berkeley.edu/talks/tbd-465
Quantifying Uncertainty: Stochastic Adversarial and Beyond
We study a model of retrospective search in which an agent—a researcher, an online shopper, or a politician—tracks the value of a product. Discoveries beget discoveries and their observations are correlated over time, which we model using a Brownian motion. The agent, a standard exponential discounter, chooses the speed and length of search. We fully characterize the optimal search policy. The optimal search speed is U-shaped, with the agent searching most intensely when approaching a breakthrough or when nearing search termination. A drawdown stopping boundary is optimal, where the agent ceases search whenever current observations fall a constant amount below the maximal achieved alternative. We also show special features that emerge from contracting with a retrospective searcher.
Visit talk page
https://simons.berkeley.edu/talks/tbd-465
Quantifying Uncertainty: Stochastic Adversarial and Beyond
We study a model of retrospective search in which an agent—a researcher, an online shopper, or a politician—tracks the value of a product. Discoveries beget discoveries and their observations are correlated over time, which we model using a Brownian motion. The agent, a standard exponential discounter, chooses the speed and length of search. We fully characterize the optimal search policy. The optimal search speed is U-shaped, with the agent searching most intensely when approaching a breakthrough or when nearing search termination. A drawdown stopping boundary is optimal, where the agent ceases search whenever current observations fall a constant amount below the maximal achieved alternative. We also show special features that emerge from contracting with a retrospective searcher.
0:51:15
Steve Callander (Stanford University)
https://simons.berkeley.edu/talks/tbd-466
Quantifying Uncertainty: Stochastic, Adversarial, and Beyond
The standard approach of economic theory is to simplify, simplify, simplify. In many contexts, from experimentation to search to communication, this implies a state of the world that is often as simple as a single piece of information. Reality is much messier, and typically there is much that decision makers don’t know. This distinction is particularly stark in models of expert advice. The insight from classic models of cheap talk is that communication is imperfect when the expert is biased, and the outcome is both inefficient and unfavorable to the expert, whereas in practice, experts hold considerable power over uninformed decision-makers. We show that this dissonance is due to simplification of expertise in classic models. We introduce a model of expert advice in which the mapping from actions to outcomes is given by the realized path of a Brownian motion. We show in this environment that strategic communication can be efficient and highly favorable to the expert. Communication is efficient not despite the complexity, but rather because of it.
Visit talk page
https://simons.berkeley.edu/talks/tbd-466
Quantifying Uncertainty: Stochastic, Adversarial, and Beyond
The standard approach of economic theory is to simplify, simplify, simplify. In many contexts, from experimentation to search to communication, this implies a state of the world that is often as simple as a single piece of information. Reality is much messier, and typically there is much that decision makers don’t know. This distinction is particularly stark in models of expert advice. The insight from classic models of cheap talk is that communication is imperfect when the expert is biased, and the outcome is both inefficient and unfavorable to the expert, whereas in practice, experts hold considerable power over uninformed decision-makers. We show that this dissonance is due to simplification of expertise in classic models. We introduce a model of expert advice in which the mapping from actions to outcomes is given by the realized path of a Brownian motion. We show in this environment that strategic communication can be efficient and highly favorable to the expert. Communication is efficient not despite the complexity, but rather because of it.
0:43:6
Kyra Gan (Harvard University)
https://simons.berkeley.edu/talks/tbd-467
Quantifying Uncertainty: Stochastic, Adversarial, and Beyond
In the problem of active sequential hypothesis testing (ASHT), a learner seeks to identify the true hypothesis from among a known set of hypotheses. The learner is given a set of actions and knows the random distribution of the outcome of any action under any true hypothesis. Given a target error δ greater than 0, the goal is to sequentially select the fewest number of actions so as to identify the true hypothesis with probability at least 1 - δ. Motivated by applications in which the number of hypotheses or actions is massive (e.g., genomics-based cancer detection), we propose efficient (greedy, in fact) algorithms and provide the first approximation guarantees for ASHT, under two types of adaptivity. Both of our guarantees are independent of the number of actions and logarithmic in the number of hypotheses. We numerically evaluate the performance of our algorithms using both synthetic and real-world DNA mutation data, demonstrating that our algorithms outperform previously proposed heuristic policies by large margins.
Visit talk page
https://simons.berkeley.edu/talks/tbd-467
Quantifying Uncertainty: Stochastic, Adversarial, and Beyond
In the problem of active sequential hypothesis testing (ASHT), a learner seeks to identify the true hypothesis from among a known set of hypotheses. The learner is given a set of actions and knows the random distribution of the outcome of any action under any true hypothesis. Given a target error δ greater than 0, the goal is to sequentially select the fewest number of actions so as to identify the true hypothesis with probability at least 1 - δ. Motivated by applications in which the number of hypotheses or actions is massive (e.g., genomics-based cancer detection), we propose efficient (greedy, in fact) algorithms and provide the first approximation guarantees for ASHT, under two types of adaptivity. Both of our guarantees are independent of the number of actions and logarithmic in the number of hypotheses. We numerically evaluate the performance of our algorithms using both synthetic and real-world DNA mutation data, demonstrating that our algorithms outperform previously proposed heuristic policies by large margins.
0:46:45
Anish Agarwal (MIT)
https://simons.berkeley.edu/talks/tbd-468
Quantifying Uncertainty: Stochastic, Adversarial, and Beyond
Matrix completion is the study of recovering an underlying matrix from a sparse subset of noisy observations. Traditionally, it is assumed entries of the matrix are “missing completely at random” (MCAR), i.e., each entry is revealed at random, independent of everything else, with uniform probability. This is likely unrealistic due to the presence of “latent confounders”, i.e., unobserved factors that determine both the entries of the underlying matrix and the missingness pattern in the observed matrix. For example, in the context of movie recommender systems—a canonical application for matrix completion—a user who vehemently dislikes horror films is unlikely to ever watch horror films. In general, these confounders yield “missing not at random” (MNAR) data, which can severely impact any inference procedure. We develop a formal causal model for matrix completion through the language of potential outcomes, and provide novel identification arguments for a variety of causal estimands of interest. We combine a nearest neighbors approach for matrix completion —popularly known as collaborative filtering—with the synthetic controls approach for panel data, to design a simple two-step algorithm, which we call “synthetic nearest neighbors” (SNN) to estimate these causal estimands. We prove entry-wise finite-sample consistency and asymptotic normality of the SNN estimator for matrix completion with MNAR data. Lastly, we show how algorithms for reinforcement learning, where the data is collected offline and there is potentially unobserved confounding in how actions are picked, can be effectively designed through the lens of causal tensor completion, an extension of matrix completion to higher dimensions.
Visit talk page
https://simons.berkeley.edu/talks/tbd-468
Quantifying Uncertainty: Stochastic, Adversarial, and Beyond
Matrix completion is the study of recovering an underlying matrix from a sparse subset of noisy observations. Traditionally, it is assumed entries of the matrix are “missing completely at random” (MCAR), i.e., each entry is revealed at random, independent of everything else, with uniform probability. This is likely unrealistic due to the presence of “latent confounders”, i.e., unobserved factors that determine both the entries of the underlying matrix and the missingness pattern in the observed matrix. For example, in the context of movie recommender systems—a canonical application for matrix completion—a user who vehemently dislikes horror films is unlikely to ever watch horror films. In general, these confounders yield “missing not at random” (MNAR) data, which can severely impact any inference procedure. We develop a formal causal model for matrix completion through the language of potential outcomes, and provide novel identification arguments for a variety of causal estimands of interest. We combine a nearest neighbors approach for matrix completion —popularly known as collaborative filtering—with the synthetic controls approach for panel data, to design a simple two-step algorithm, which we call “synthetic nearest neighbors” (SNN) to estimate these causal estimands. We prove entry-wise finite-sample consistency and asymptotic normality of the SNN estimator for matrix completion with MNAR data. Lastly, we show how algorithms for reinforcement learning, where the data is collected offline and there is potentially unobserved confounding in how actions are picked, can be effectively designed through the lens of causal tensor completion, an extension of matrix completion to higher dimensions.
0:45:15
Hannah Li (Stanford)
https://simons.berkeley.edu/talks/tbd-469
Quantifying Uncertainty: Stochastic, Adversarial, and Beyond
Online marketplace platforms rely on experiments to aid decision-making. Due to interference effects, however, common estimators may be biased in these market settings. Prior work has focused on the biases that arise in the treatment effect estimates, but there is also bias in the typical standard error estimates, which can cause the platform to be under or over-confident in its estimates. In this work we study the standard error bias and its impact on the resulting platform decisions. We utilize a dynamic market model which captures the marketplace interference effects. We show that commonly used standard error estimators are biased in market settings. We explore practical methods to reduce the standard error bias. Finally, using calibrations to real marketplace data, we assess the quality of the ultimate decisions made using these biased estimates.
Visit talk page
https://simons.berkeley.edu/talks/tbd-469
Quantifying Uncertainty: Stochastic, Adversarial, and Beyond
Online marketplace platforms rely on experiments to aid decision-making. Due to interference effects, however, common estimators may be biased in these market settings. Prior work has focused on the biases that arise in the treatment effect estimates, but there is also bias in the typical standard error estimates, which can cause the platform to be under or over-confident in its estimates. In this work we study the standard error bias and its impact on the resulting platform decisions. We utilize a dynamic market model which captures the marketplace interference effects. We show that commonly used standard error estimators are biased in market settings. We explore practical methods to reduce the standard error bias. Finally, using calibrations to real marketplace data, we assess the quality of the ultimate decisions made using these biased estimates.
0:47:56
Vivek Farias (Massachusetts Institute of Technology)
https://simons.berkeley.edu/talks/tbd-470
Quantifying Uncertainty: Stochastic, Adversarial, and Beyond
We consider experiments in dynamical systems where interventions on some experimental units may, over time, impact other units (say, through a limiting constraint such as a limited inventory). Despite outsize practical importance, the best estimators for this `Markovian' interference problem are largely heuristic in nature, and their bias is not well understood. We formalize the problem of inference in such experiments as one of policy evaluation. Off-policy estimators, while unbiased, apparently incur a large penalty in variance relative to state-of-the-art heuristics. We introduce an on-policy estimator: the Differences-In-Q's (DQ) estimator. We show that the DQ estimator can in general have exponentially smaller variance than off-policy evaluation. At the same time, its bias is second order in the impact of the intervention. This yields a striking bias-variance tradeoff so that the DQ estimator effectively dominates state-of-the-art alternatives. Our empirical evaluation includes a set of experiments on a city-scale ride-hailing simulator. Joint work with Andrew Li (CMU), Tianyi Peng (MIT) and Andy Zheng (MIT).
Visit talk page
https://simons.berkeley.edu/talks/tbd-470
Quantifying Uncertainty: Stochastic, Adversarial, and Beyond
We consider experiments in dynamical systems where interventions on some experimental units may, over time, impact other units (say, through a limiting constraint such as a limited inventory). Despite outsize practical importance, the best estimators for this `Markovian' interference problem are largely heuristic in nature, and their bias is not well understood. We formalize the problem of inference in such experiments as one of policy evaluation. Off-policy estimators, while unbiased, apparently incur a large penalty in variance relative to state-of-the-art heuristics. We introduce an on-policy estimator: the Differences-In-Q's (DQ) estimator. We show that the DQ estimator can in general have exponentially smaller variance than off-policy evaluation. At the same time, its bias is second order in the impact of the intervention. This yields a striking bias-variance tradeoff so that the DQ estimator effectively dominates state-of-the-art alternatives. Our empirical evaluation includes a set of experiments on a city-scale ride-hailing simulator. Joint work with Andrew Li (CMU), Tianyi Peng (MIT) and Andy Zheng (MIT).
0:48:56
Panos Toulis (University of Chicago)
https://simons.berkeley.edu/talks/tbd-471
Quantifying Uncertainty: Stochastic, Adversarial, and Beyond
The standard frameworks of causal inference, either based on potential outcomes or causal graphs, rely on a stability assumption of no interference. This means that an individual's outcomes may depend only on the individual's treatment. This assumption is realistic in some restricted settings, e.g., "my headache outcome should not depend on my neighbor taking an aspirin". However, it is too simplistic for many other applied settings; e.g., "my infection outcome actually depends on my neighbor's vaccination status". In this talk, I will present some recent work on tackling the challenges behind causal inference under interference. Specifically, I will consider settings where it is assumed that interference occurs through a pre-specified network between units (e.g., a network of social ties). Despite the complications from interference, these settings are amenable to analysis because the network is usually considered fixed and exogeneous. My focus will be on experimental studies, where the randomization of treatment is controlled. In this context, I will talk about the randomization method of inference. This method is not as well-known in fields outside of statistics, but it deserves attention as it offers a powerful approach to robust, "distribution-free" estimation of treatment effects. I will conclude with examples of interference stemming from dynamics or strategic interactions between agents in a market, which largely remain unresolved.
Visit talk page
https://simons.berkeley.edu/talks/tbd-471
Quantifying Uncertainty: Stochastic, Adversarial, and Beyond
The standard frameworks of causal inference, either based on potential outcomes or causal graphs, rely on a stability assumption of no interference. This means that an individual's outcomes may depend only on the individual's treatment. This assumption is realistic in some restricted settings, e.g., "my headache outcome should not depend on my neighbor taking an aspirin". However, it is too simplistic for many other applied settings; e.g., "my infection outcome actually depends on my neighbor's vaccination status". In this talk, I will present some recent work on tackling the challenges behind causal inference under interference. Specifically, I will consider settings where it is assumed that interference occurs through a pre-specified network between units (e.g., a network of social ties). Despite the complications from interference, these settings are amenable to analysis because the network is usually considered fixed and exogeneous. My focus will be on experimental studies, where the randomization of treatment is controlled. In this context, I will talk about the randomization method of inference. This method is not as well-known in fields outside of statistics, but it deserves attention as it offers a powerful approach to robust, "distribution-free" estimation of treatment effects. I will conclude with examples of interference stemming from dynamics or strategic interactions between agents in a market, which largely remain unresolved.
0:42:31
Ilan Lobel (NYU Stern)
https://simons.berkeley.edu/talks/contextual-inverse-optimization-offline-and-online-learning
Quantifying Uncertainty: Stochastic, Adversarial, and Beyond
We study the problems of offline and online contextual optimization with feedback information, where instead of observing the loss, we observe, after-the-fact, the optimal action an oracle with full knowledge of the objective function would have taken. We aim to minimize regret, which is defined as the difference between our losses and the ones incurred by an all-knowing oracle. In the offline setting, the decision-maker has information available from past periods and needs to make one decision, while in the online setting, the decision-maker optimizes decisions dynamically over time based a new set of feasible actions and contextual functions in each period. For the offline setting, we characterize the optimal minimax policy, establishing the performance that can be achieved as a function of the underlying geometry of the information induced by the data. In the online setting, we leverage this geometric characterization to optimize the cumulative regret. We develop an algorithm that yields the first regret bound for this problem that is logarithmic in the time horizon. Finally, we show via simulation that our proposed algorithms outperform previous methods from the literature.
Visit talk page
https://simons.berkeley.edu/talks/contextual-inverse-optimization-offline-and-online-learning
Quantifying Uncertainty: Stochastic, Adversarial, and Beyond
We study the problems of offline and online contextual optimization with feedback information, where instead of observing the loss, we observe, after-the-fact, the optimal action an oracle with full knowledge of the objective function would have taken. We aim to minimize regret, which is defined as the difference between our losses and the ones incurred by an all-knowing oracle. In the offline setting, the decision-maker has information available from past periods and needs to make one decision, while in the online setting, the decision-maker optimizes decisions dynamically over time based a new set of feasible actions and contextual functions in each period. For the offline setting, we characterize the optimal minimax policy, establishing the performance that can be achieved as a function of the underlying geometry of the information induced by the data. In the online setting, we leverage this geometric characterization to optimize the cumulative regret. We develop an algorithm that yields the first regret bound for this problem that is logarithmic in the time horizon. Finally, we show via simulation that our proposed algorithms outperform previous methods from the literature.
0:45:35
Chara Podimata (UC Berkeley)
https://simons.berkeley.edu/talks/tbd-472
Quantifying Uncertainty: Stochastic, Adversarial, and Beyond
Contextual search is a generalization of binary search in higher dimensions, which captures settings such as feature-based dynamic pricing. Standard formulations of this problem assume that agents act in accordance with a specific homogeneous response model. In practice, however, some responses may be adversarially corrupted. Existing algorithms heavily depend on the assumed response model being (approximately) accurate for all agents and have poor performance in the presence of even a few such arbitrary misspecifications. In the corruption-robust version of the problem, we wish to model misspecifications as corruptions and create algorithms that scale nearly optimally both when corruptions are present and when they are not.
This talk will cover the original formulation of the problem as proposed by Krishnamurthy, Lykouris, Podimata, and Schapire in STOC21/OR22 and the nearly optimal algorithms attained by Paes Leme, Podimata, and Schneider in COLT22.
Visit talk page
https://simons.berkeley.edu/talks/tbd-472
Quantifying Uncertainty: Stochastic, Adversarial, and Beyond
Contextual search is a generalization of binary search in higher dimensions, which captures settings such as feature-based dynamic pricing. Standard formulations of this problem assume that agents act in accordance with a specific homogeneous response model. In practice, however, some responses may be adversarially corrupted. Existing algorithms heavily depend on the assumed response model being (approximately) accurate for all agents and have poor performance in the presence of even a few such arbitrary misspecifications. In the corruption-robust version of the problem, we wish to model misspecifications as corruptions and create algorithms that scale nearly optimally both when corruptions are present and when they are not.
This talk will cover the original formulation of the problem as proposed by Krishnamurthy, Lykouris, Podimata, and Schapire in STOC21/OR22 and the nearly optimal algorithms attained by Paes Leme, Podimata, and Schneider in COLT22.