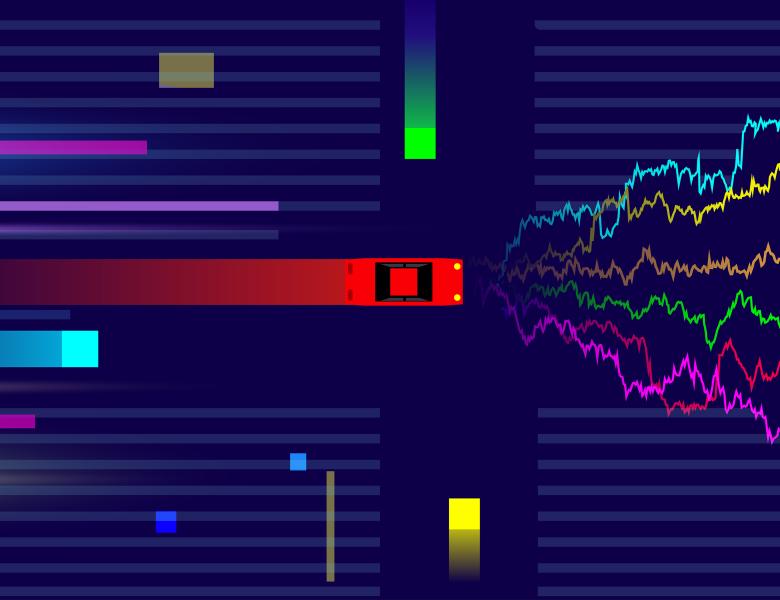
Abstract
Online marketplace platforms rely on experiments to aid decision-making. Due to interference effects, however, common estimators may be biased in these market settings. Prior work has focused on the biases that arise in the treatment effect estimates, but there is also bias in the typical standard error estimates, which can cause the platform to be under or over-confident in its estimates. In this work we study the standard error bias and its impact on the resulting platform decisions. We utilize a dynamic market model which captures the marketplace interference effects. We show that commonly used standard error estimators are biased in market settings. We explore practical methods to reduce the standard error bias. Finally, using calibrations to real marketplace data, we assess the quality of the ultimate decisions made using these biased estimates.