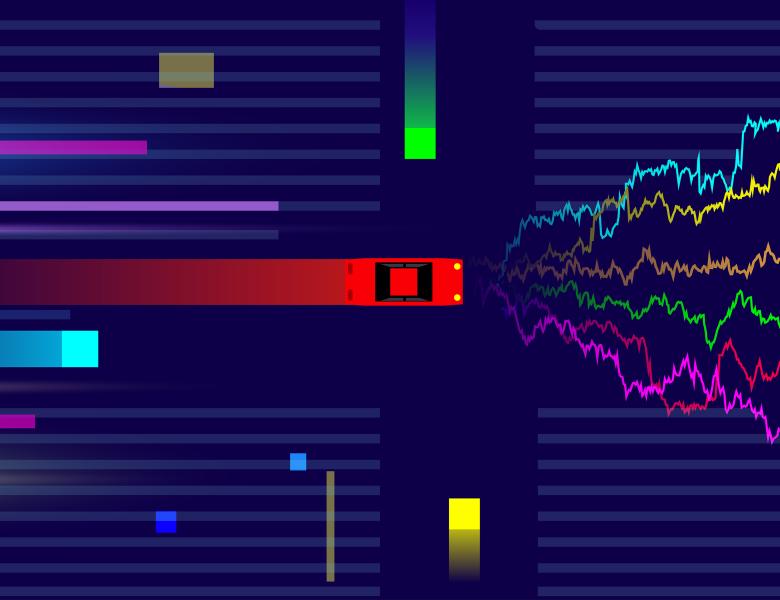
Abstract
We consider experiments in dynamical systems where interventions on some experimental units may, over time, impact other units (say, through a limiting constraint such as a limited inventory). Despite outsize practical importance, the best estimators for this `Markovian' interference problem are largely heuristic in nature, and their bias is not well understood. We formalize the problem of inference in such experiments as one of policy evaluation. Off-policy estimators, while unbiased, apparently incur a large penalty in variance relative to state-of-the-art heuristics. We introduce an on-policy estimator: the Differences-In-Q's (DQ) estimator. We show that the DQ estimator can in general have exponentially smaller variance than off-policy evaluation. At the same time, its bias is second order in the impact of the intervention. This yields a striking bias-variance tradeoff so that the DQ estimator effectively dominates state-of-the-art alternatives. Our empirical evaluation includes a set of experiments on a city-scale ride-hailing simulator. Joint work with Andrew Li (CMU), Tianyi Peng (MIT) and Andy Zheng (MIT).