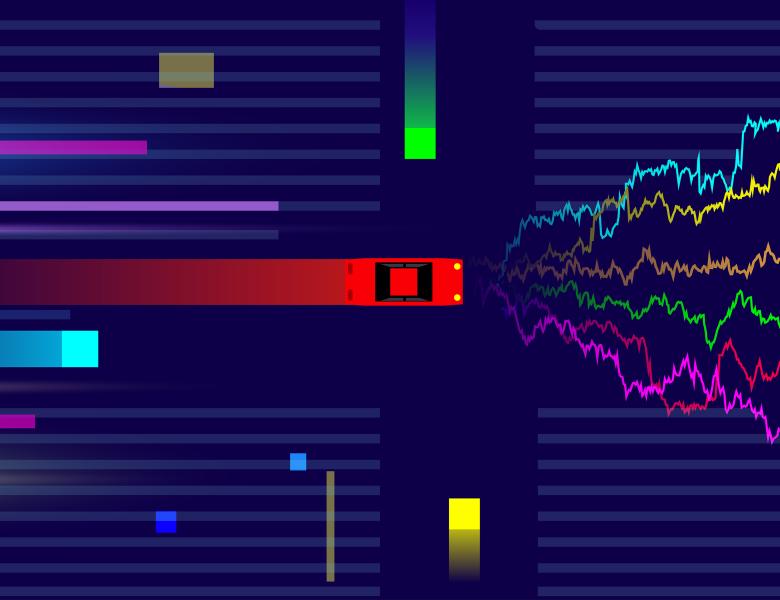
Abstract
The standard frameworks of causal inference, either based on potential outcomes or causal graphs, rely on a stability assumption of no interference. This means that an individual's outcomes may depend only on the individual's treatment. This assumption is realistic in some restricted settings, e.g., "my headache outcome should not depend on my neighbor taking an aspirin". However, it is too simplistic for many other applied settings; e.g., "my infection outcome actually depends on my neighbor's vaccination status". In this talk, I will present some recent work on tackling the challenges behind causal inference under interference. Specifically, I will consider settings where it is assumed that interference occurs through a pre-specified network between units (e.g., a network of social ties). Despite the complications from interference, these settings are amenable to analysis because the network is usually considered fixed and exogeneous. My focus will be on experimental studies, where the randomization of treatment is controlled. In this context, I will talk about the randomization method of inference. This method is not as well-known in fields outside of statistics, but it deserves attention as it offers a powerful approach to robust, "distribution-free" estimation of treatment effects. I will conclude with examples of interference stemming from dynamics or strategic interactions between agents in a market, which largely remain unresolved.