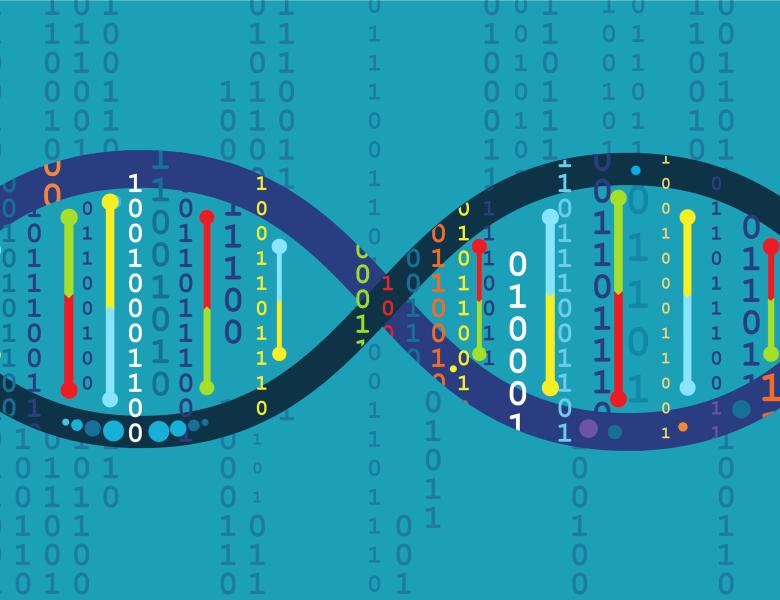
0:42:25
Hagen Tilgner (Weill Cornell Medicine)
https://simons.berkeley.edu/talks/single-cell-brain-isoforms-space-and-time
Computational Challenges in Very Large-Scale 'Omics'
Most mammalian genes encode multiple distinct RNA isoforms and the brain harbors especially diverse isoforms. Complex tissue, including the brain, often include highly divergent cell types and these cell types employ distinct isoforms for many genes. To untangle the distinct cell-type specific isoform profiles of the brain, we developed Single-cell isoform RNA sequencing (ScISOr-Seq (ref1)) for fresh tissues as well Single-nuclei isoform RNA sequencing (SnISOr-Seq (ref2)) for frozen tissues. To add spatial resolution, we developed Slide-isoform sequencing (ref3). Collectively, these long-read approaches reveal a striking difference between coordinated pairs of exons with in-between exons (“Distant coordinated exons”) and without in-between exons (“Adjacent coordinated exons”): The former show strong enrichment for cell-type specific usage of exons, whereas the latter do not in mouse (ref1) and human brain (ref2). Of note, coordinated TSS-exon pairs and exon-polyA-site pairs follow the same trend as distant coordinated exon pairs (ref2). Simultaneously, autism-associated exons are among the most highly variably used exons across cell types (ref2). Differences in isoform expression between hippocampus and prefrontal cortex are most often explained by differences arising between the two regions in one specific cell type (e.g., excitatory neurons), but for a smaller program of genes brain regions can override cell-type identity3. Spatially barcoded isoform sequencing revealed that often region-specific isoform differences correlate with precise boundaries of brain structures (e.g., from the choroid plexus to the hippocampus). However, genes including Snap25 go against this trend, using a steady gradient of exon inclusion as one traverses the brain (ref3). Moreover, choroid plexus epithelial cells show a dramatically distinct isoform profile, which originates from distinct exon and poly(A) site usage, but most strongly from distinct TSS usage (ref3). Most recently, we have made advances in understanding the error sources of Pacific Biosciences and Oxford Nanopore long-read sequencing by sequencing cDNA representations of the same unique RNA on both platforms (ref4) and have implemented a highly accurate long-read interpretation pipeline (ref5). 1. Gupta*, Collier* et al, Nature Biotechnology, 2018 2. Hardwick*, Hu*, Joglekar* et al, Nature Biotechnology, 2022 3. Joglekar et al, Nature Communications, 2021 4. Mikheenko*, Prjibelski*, Genome Research, 2022 5. Prjibelski et al, https://assets.researchsquare.com/files/rs-1571850/v1_covered.pdf
Visit talk page
https://simons.berkeley.edu/talks/single-cell-brain-isoforms-space-and-time
Computational Challenges in Very Large-Scale 'Omics'
Most mammalian genes encode multiple distinct RNA isoforms and the brain harbors especially diverse isoforms. Complex tissue, including the brain, often include highly divergent cell types and these cell types employ distinct isoforms for many genes. To untangle the distinct cell-type specific isoform profiles of the brain, we developed Single-cell isoform RNA sequencing (ScISOr-Seq (ref1)) for fresh tissues as well Single-nuclei isoform RNA sequencing (SnISOr-Seq (ref2)) for frozen tissues. To add spatial resolution, we developed Slide-isoform sequencing (ref3). Collectively, these long-read approaches reveal a striking difference between coordinated pairs of exons with in-between exons (“Distant coordinated exons”) and without in-between exons (“Adjacent coordinated exons”): The former show strong enrichment for cell-type specific usage of exons, whereas the latter do not in mouse (ref1) and human brain (ref2). Of note, coordinated TSS-exon pairs and exon-polyA-site pairs follow the same trend as distant coordinated exon pairs (ref2). Simultaneously, autism-associated exons are among the most highly variably used exons across cell types (ref2). Differences in isoform expression between hippocampus and prefrontal cortex are most often explained by differences arising between the two regions in one specific cell type (e.g., excitatory neurons), but for a smaller program of genes brain regions can override cell-type identity3. Spatially barcoded isoform sequencing revealed that often region-specific isoform differences correlate with precise boundaries of brain structures (e.g., from the choroid plexus to the hippocampus). However, genes including Snap25 go against this trend, using a steady gradient of exon inclusion as one traverses the brain (ref3). Moreover, choroid plexus epithelial cells show a dramatically distinct isoform profile, which originates from distinct exon and poly(A) site usage, but most strongly from distinct TSS usage (ref3). Most recently, we have made advances in understanding the error sources of Pacific Biosciences and Oxford Nanopore long-read sequencing by sequencing cDNA representations of the same unique RNA on both platforms (ref4) and have implemented a highly accurate long-read interpretation pipeline (ref5). 1. Gupta*, Collier* et al, Nature Biotechnology, 2018 2. Hardwick*, Hu*, Joglekar* et al, Nature Biotechnology, 2022 3. Joglekar et al, Nature Communications, 2021 4. Mikheenko*, Prjibelski*, Genome Research, 2022 5. Prjibelski et al, https://assets.researchsquare.com/files/rs-1571850/v1_covered.pdf
0:31:45
Jingyi Jessica Li (University of California, Los Angeles)
https://simons.berkeley.edu/talks/benchmarking-inference-and-silico-controls-single-cell-and-spatial-omics-data-science
Computational Challenges in Very Large-Scale 'Omics'
Realistic synthetic data are essential for benchmarking the many computation tools developed for single-cell and spatial omics data. Here we propose a unified statistical framework scDesign3, which generates single-cell and spatial omics data from discrete cell types and continuous cell trajectories. Notably, scDesign3 uses a unified probabilistic model with an accessible likelihood. This probabilistic formulation is advantageous in that it enables the inference of the cell heterogeneity structure that fits a dataset, by leveraging the statistical model selection principle. Moreover, scDesign3 has interpretable parameters that can be adjusted to generate in silico negative and positive controls, providing the basis for false discovery rate control and power evaluation. In addition, scDesign3 coupled with scReadSim can generate sequence reads in addition to read counts, allowing the benchmarking of low-level computational tools.
Visit talk page
https://simons.berkeley.edu/talks/benchmarking-inference-and-silico-controls-single-cell-and-spatial-omics-data-science
Computational Challenges in Very Large-Scale 'Omics'
Realistic synthetic data are essential for benchmarking the many computation tools developed for single-cell and spatial omics data. Here we propose a unified statistical framework scDesign3, which generates single-cell and spatial omics data from discrete cell types and continuous cell trajectories. Notably, scDesign3 uses a unified probabilistic model with an accessible likelihood. This probabilistic formulation is advantageous in that it enables the inference of the cell heterogeneity structure that fits a dataset, by leveraging the statistical model selection principle. Moreover, scDesign3 has interpretable parameters that can be adjusted to generate in silico negative and positive controls, providing the basis for false discovery rate control and power evaluation. In addition, scDesign3 coupled with scReadSim can generate sequence reads in addition to read counts, allowing the benchmarking of low-level computational tools.
0:34:15
Davide Risso (University of Padova)
Learning Gene Association Networks Using Single-Cell RNA-Seq Data: A Graphical Model Approach
Computational Challenges in Very Large-Scale 'Omics'
I will present a general framework for learning the structure of a graph from single-cell RNA-seq data, based on a graphical model for count data. I will explore the use of different node-conditional distributions, including Poisson, negative binomial, and zero-inflated negative binomial, and discuss the advantages of each. I will show with simulations that our approach is able to retrieve the structure of a graph in a variety of settings and I will show the utility of the approach on two real datasets in the context of stem cell differentiation and response to treatment in cancer.
Visit talk page
Learning Gene Association Networks Using Single-Cell RNA-Seq Data: A Graphical Model Approach
Computational Challenges in Very Large-Scale 'Omics'
I will present a general framework for learning the structure of a graph from single-cell RNA-seq data, based on a graphical model for count data. I will explore the use of different node-conditional distributions, including Poisson, negative binomial, and zero-inflated negative binomial, and discuss the advantages of each. I will show with simulations that our approach is able to retrieve the structure of a graph in a variety of settings and I will show the utility of the approach on two real datasets in the context of stem cell differentiation and response to treatment in cancer.
0:27:1
Oliver Stegle (German Cancer Research Center, EMBL Heidelberg)
https://simons.berkeley.edu/talks/mapping-gene-regulatory-dependencies-single-cell-resolution
Computational Challenges in Very Large-Scale 'Omics'
The study of genetic effects on gene expression and other molecular traits using bulk sequencing has allowed for the functional annotation of disease variants in diverse human tissues. Advances in single-cell RNA sequencing and multi-omics protocols provide for unprecedented opportunities to increase the resolution of such genetic analyses, allowing to assess gene regulatory effects at the resolution of cell types, cell states and even in individual cells in human tissues. In the first part of this talk, I will present computational strategies for analyzing and integrating population-scale single-cell dataset. A challenge I will discuss is to leverage these data to map genetic effects at the resolution of cell types but also subtle subtypes in a data-driven manner. I will describe applications of these strategies to population-scale single-cell sequencing dataset from genetically diverse human iPSCs across differentiation towards a neuronal fate, identifying dynamic changes of regulatory variants. In the second part I will discuss extensions to use genetic engineering to assay tissue-targeted perturbations using single-cell readouts.
Visit talk page
https://simons.berkeley.edu/talks/mapping-gene-regulatory-dependencies-single-cell-resolution
Computational Challenges in Very Large-Scale 'Omics'
The study of genetic effects on gene expression and other molecular traits using bulk sequencing has allowed for the functional annotation of disease variants in diverse human tissues. Advances in single-cell RNA sequencing and multi-omics protocols provide for unprecedented opportunities to increase the resolution of such genetic analyses, allowing to assess gene regulatory effects at the resolution of cell types, cell states and even in individual cells in human tissues. In the first part of this talk, I will present computational strategies for analyzing and integrating population-scale single-cell dataset. A challenge I will discuss is to leverage these data to map genetic effects at the resolution of cell types but also subtle subtypes in a data-driven manner. I will describe applications of these strategies to population-scale single-cell sequencing dataset from genetically diverse human iPSCs across differentiation towards a neuronal fate, identifying dynamic changes of regulatory variants. In the second part I will discuss extensions to use genetic engineering to assay tissue-targeted perturbations using single-cell readouts.
0:29:6
Elizabeth Purdom (UC Berkeley)
https://simons.berkeley.edu/talks/harnessing-multimodal-single-cell-sequencing-data-integrative-analysis
Computational Challenges in Very Large-Scale 'Omics'
Single-cell sequencing allows for quantifying molecular traits at the single-cell level. Comparison of different cellular features (or modalities) from cells from the same biological system gives the potential for a holistic understanding of the system. A growing number of multi-modality sequencing platforms jointly measure multiple modalities from the same cells but most single-cell sequencing datasets measure a single modality, resulting in disjoint measurements of different modalities on different cells. We present method, Cobolt, that not only allows for analyzing data from multi-modality platforms, but also provides a coherent framework for harnessing the multi-modality data to allow for the the integration of single-modality datasets. Cobolt estimates this joint representation of all cells irrespective of modalities via a novel application of Multimodal Variational Autoencoder (MVAE) to a hierarchical generative model. We demonstrate the performance of Cobolt in two systems -- cortical brain cells in mouse and PBMC cells from humans -- where Cobolt allows for the integration of multi-modality data with single-modality datasets of scRNA-seq and ATAC-seq.
Visit talk page
https://simons.berkeley.edu/talks/harnessing-multimodal-single-cell-sequencing-data-integrative-analysis
Computational Challenges in Very Large-Scale 'Omics'
Single-cell sequencing allows for quantifying molecular traits at the single-cell level. Comparison of different cellular features (or modalities) from cells from the same biological system gives the potential for a holistic understanding of the system. A growing number of multi-modality sequencing platforms jointly measure multiple modalities from the same cells but most single-cell sequencing datasets measure a single modality, resulting in disjoint measurements of different modalities on different cells. We present method, Cobolt, that not only allows for analyzing data from multi-modality platforms, but also provides a coherent framework for harnessing the multi-modality data to allow for the the integration of single-modality datasets. Cobolt estimates this joint representation of all cells irrespective of modalities via a novel application of Multimodal Variational Autoencoder (MVAE) to a hierarchical generative model. We demonstrate the performance of Cobolt in two systems -- cortical brain cells in mouse and PBMC cells from humans -- where Cobolt allows for the integration of multi-modality data with single-modality datasets of scRNA-seq and ATAC-seq.
0:33:36
Lior Pachter (Caltech)
https://simons.berkeley.edu/talks/tbd-457
Computational Challenges in Very Large-Scale 'Omics'
I will discuss several computational challenges that must be addressed in order to learn biophysically meaningful representations of cells from large-scale single-cell ‘omics’ data. I will discuss some progress we have made in attempting to address the challenges by way of developing a commons cell atlas framework for large-scale (isoform) resolved single-cell analysis, and explain how analysis of large-scale data can inform biophysical models of cells.
Visit talk page
https://simons.berkeley.edu/talks/tbd-457
Computational Challenges in Very Large-Scale 'Omics'
I will discuss several computational challenges that must be addressed in order to learn biophysically meaningful representations of cells from large-scale single-cell ‘omics’ data. I will discuss some progress we have made in attempting to address the challenges by way of developing a commons cell atlas framework for large-scale (isoform) resolved single-cell analysis, and explain how analysis of large-scale data can inform biophysical models of cells.
1:24:0
https://simons.berkeley.edu/talks/building-regulatory-atlas-based-5-single-cell-rna-seq
Computational Challenges in Very Large-Scale 'Omics'
Visit talk page
Computational Challenges in Very Large-Scale 'Omics'
0:34:36
Sunduz Keles (University of Wisconsin, Madison)
https://simons.berkeley.edu/talks/exploratory-and-model-based-analysis-schi-c-data
Computational Challenges in Very Large-Scale 'Omics'
Single-cell high-throughput chromatin conformation capture methodologies (scHi-C) enable profiling long-range genomic interactions; however, data from these technologies are prone to technical noise and biases that hinder downstream analysis. I will discuss normalization and denoising issues of scHi-C data by introducing a simple normalization approach named BandNorm and a deep generative modeling framework, scVI-3D, to account for scHi-C specific biases. I will also introduce single-cell gene associating domain (scGAD) scores as a dimension reduction and exploratory analysis tool for scHi-C data and illustrate how this approach enables integration of scHi-C data with other single cell data modalities.
Visit talk page
https://simons.berkeley.edu/talks/exploratory-and-model-based-analysis-schi-c-data
Computational Challenges in Very Large-Scale 'Omics'
Single-cell high-throughput chromatin conformation capture methodologies (scHi-C) enable profiling long-range genomic interactions; however, data from these technologies are prone to technical noise and biases that hinder downstream analysis. I will discuss normalization and denoising issues of scHi-C data by introducing a simple normalization approach named BandNorm and a deep generative modeling framework, scVI-3D, to account for scHi-C specific biases. I will also introduce single-cell gene associating domain (scGAD) scores as a dimension reduction and exploratory analysis tool for scHi-C data and illustrate how this approach enables integration of scHi-C data with other single cell data modalities.
0:35:41
Harris Lewin (University of California, Davis)
https://simons.berkeley.edu/talks/earth-biogenome-project-progress-and-challenges-ahead
Computational Challenges in Very Large-Scale 'Omics'
The Earth BioGenome Project (EBP) aims to sequence, catalog, and characterize the genomes of all of Earth’s eukaryotic biodiversity. The ultimate aim is to use these genomes as a foundation for revealing the “rules of life,” i.e., how biological complexity arose, the relationship between genotype and phenotype, and how biological systems evolve under changing environmental conditions. EBP affiliated projects and sequencing nodes are now producing annotated reference-quality genomes at a pace to complete the Phase I goal of sequencing at least one representative species of all ~9,400 eukaryotic taxonomic families in the next three years. Despite this early progress, the project still faces many challenges in achieving Phase I, Phase II (all genera) and Phase III (all remaining species) in a 10-year timeframe. In my talk, I will review progress made by EBP-affiliated projects and will focus on the technical and computational challenges in producing and analyzing reference-quality genomes at scale, across the eukaryotic tree of life and at ecosystem level. Meeting these challenges is imperative and will lead to advances that will address significant global problems, such as mitigating the effects of climate change on biodiversity and agriculture, conserving endangered species, achieving pandemic preparedness, and identifying new sources of food, medicine and biomaterials to drive a sustainable bioeconomy.
Visit talk page
https://simons.berkeley.edu/talks/earth-biogenome-project-progress-and-challenges-ahead
Computational Challenges in Very Large-Scale 'Omics'
The Earth BioGenome Project (EBP) aims to sequence, catalog, and characterize the genomes of all of Earth’s eukaryotic biodiversity. The ultimate aim is to use these genomes as a foundation for revealing the “rules of life,” i.e., how biological complexity arose, the relationship between genotype and phenotype, and how biological systems evolve under changing environmental conditions. EBP affiliated projects and sequencing nodes are now producing annotated reference-quality genomes at a pace to complete the Phase I goal of sequencing at least one representative species of all ~9,400 eukaryotic taxonomic families in the next three years. Despite this early progress, the project still faces many challenges in achieving Phase I, Phase II (all genera) and Phase III (all remaining species) in a 10-year timeframe. In my talk, I will review progress made by EBP-affiliated projects and will focus on the technical and computational challenges in producing and analyzing reference-quality genomes at scale, across the eukaryotic tree of life and at ecosystem level. Meeting these challenges is imperative and will lead to advances that will address significant global problems, such as mitigating the effects of climate change on biodiversity and agriculture, conserving endangered species, achieving pandemic preparedness, and identifying new sources of food, medicine and biomaterials to drive a sustainable bioeconomy.
0:32:36
Kazutaka Katoh (Osaka University)
https://simons.berkeley.edu/talks/multiple-sequence-alignment-predicting-antigen-antibody-interactions
Computational Challenges in Very Large-Scale 'Omics'
A possible direction of development and application of the MAFFT multiple sequence alignment (MSA) program will be discussed. An application we are considering is the prediction of protein-protein interactions. This area has been studied well and recently AlphaFold and AlphaFold-Multimer achieved highly accurate predictions using MSAs as features. However, their well-known weak point is in prediction of antigen-antibody interactions. Our hypothesis is that one of the reasons for the difficulty is that the sequences in an MSA of antibodies have evolutionary relationships greatly different from those of other proteins. Antibodies share a common ancestry, and typically a common framework region. However, they exhibit high diversity in the complementarity-determining regions (CDRs) through recombination and hypermutations in individual cells in an individual organism. Thus, if the sequences are collected by a standard database search, an MSA of antibodies is a mixture of a variety of homologous sequences that bind different binding sites (epitopes) of various antigens. Such an MSA can be noisy for predicting the interaction between an antibody and an antigen. A solution can be to use the InterClone database, being developed in our lab. InterClone clusters antibodies by their CDR sequences and can be used to prepare a set of antibody sequences that are more likely to share an antigen and epitope than sequences gathered by a standard sequence similarity search. We are also planning to experimentally determine the positions of epitopes in several protein antigens to obtain an informative set of antibody-antigen pairs and to expand the training data for AlphaFold or other structure prediction methods.
Visit talk page
https://simons.berkeley.edu/talks/multiple-sequence-alignment-predicting-antigen-antibody-interactions
Computational Challenges in Very Large-Scale 'Omics'
A possible direction of development and application of the MAFFT multiple sequence alignment (MSA) program will be discussed. An application we are considering is the prediction of protein-protein interactions. This area has been studied well and recently AlphaFold and AlphaFold-Multimer achieved highly accurate predictions using MSAs as features. However, their well-known weak point is in prediction of antigen-antibody interactions. Our hypothesis is that one of the reasons for the difficulty is that the sequences in an MSA of antibodies have evolutionary relationships greatly different from those of other proteins. Antibodies share a common ancestry, and typically a common framework region. However, they exhibit high diversity in the complementarity-determining regions (CDRs) through recombination and hypermutations in individual cells in an individual organism. Thus, if the sequences are collected by a standard database search, an MSA of antibodies is a mixture of a variety of homologous sequences that bind different binding sites (epitopes) of various antigens. Such an MSA can be noisy for predicting the interaction between an antibody and an antigen. A solution can be to use the InterClone database, being developed in our lab. InterClone clusters antibodies by their CDR sequences and can be used to prepare a set of antibody sequences that are more likely to share an antigen and epitope than sequences gathered by a standard sequence similarity search. We are also planning to experimentally determine the positions of epitopes in several protein antigens to obtain an informative set of antibody-antigen pairs and to expand the training data for AlphaFold or other structure prediction methods.