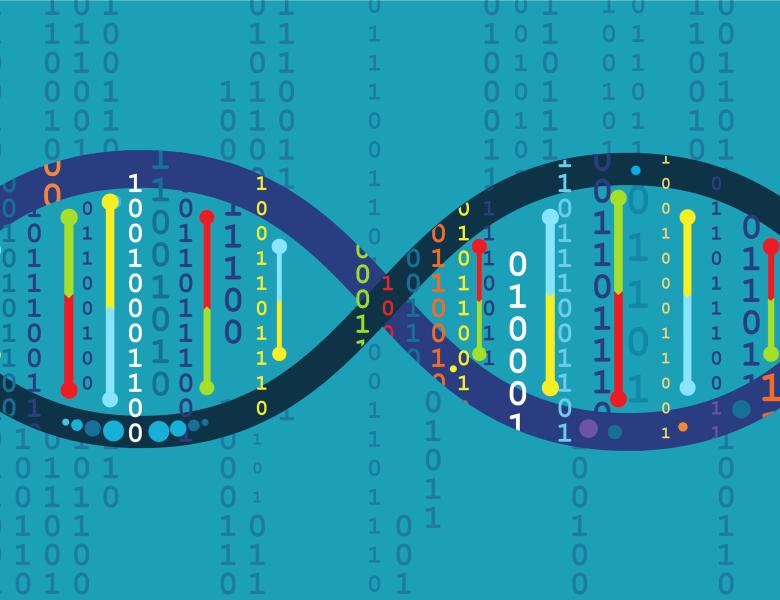
Abstract
Single-cell sequencing allows for quantifying molecular traits at the single-cell level. Comparison of different cellular features (or modalities) from cells from the same biological system gives the potential for a holistic understanding of the system. A growing number of multi-modality sequencing platforms jointly measure multiple modalities from the same cells but most single-cell sequencing datasets measure a single modality, resulting in disjoint measurements of different modalities on different cells. We present method, Cobolt, that not only allows for analyzing data from multi-modality platforms, but also provides a coherent framework for harnessing the multi-modality data to allow for the the integration of single-modality datasets. Cobolt estimates this joint representation of all cells irrespective of modalities via a novel application of Multimodal Variational Autoencoder (MVAE) to a hierarchical generative model. We demonstrate the performance of Cobolt in two systems -- cortical brain cells in mouse and PBMC cells from humans -- where Cobolt allows for the integration of multi-modality data with single-modality datasets of scRNA-seq and ATAC-seq.