Image
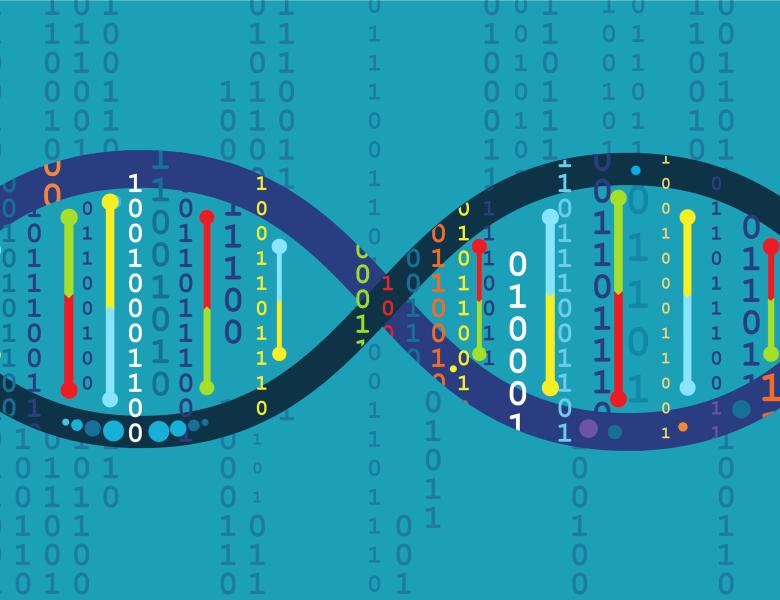
I will present a general framework for learning the structure of a graph from single-cell RNA-seq data, based on a graphical model for count data. I will explore the use of different node-conditional distributions, including Poisson, negative binomial, and zero-inflated negative binomial, and discuss the advantages of each. I will show with simulations that our approach is able to retrieve the structure of a graph in a variety of settings and I will show the utility of the approach on two real datasets in the context of stem cell differentiation and response to treatment in cancer.