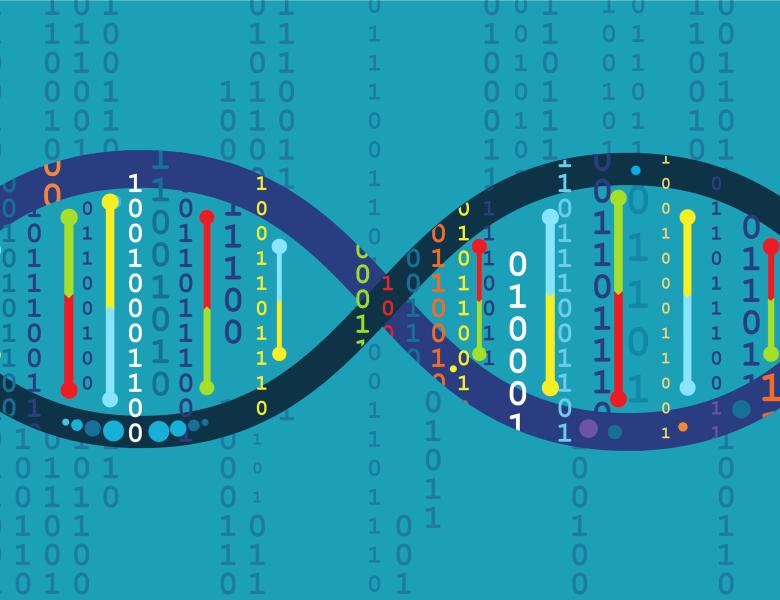
0:32:36
Priya Moorjani (University of California, Berkeley)
https://simons.berkeley.edu/talks/evolution-germline-mutation-spectrum-humans
Computational Challenges in Very Large-Scale 'Omics'
Germline mutations are the source of all heritable variation. Understanding the rate and mechanisms by which mutations occur is of paramount importance for studies of human genetics (to interpret heritable disease prevalence) and evolutionary biology (to date evolutionary events). Over the past decade, there has been a flood of data in genomics––within pedigrees, among populations and across species––that is fundamentally revising our understanding of the process of mutagenesis. In my talk, I will first briefly summarize the key findings from these different datasets and then discuss recent findings investigating differences in mutation rate and spectrum (i.e., proportions of different mutation types) across human populations. To investigate inter-population differences, we developed a framework to compare polymorphisms that arose in different time windows in the past while controlling for the effects of selection and biased gene conversion. Applying this approach to high-coverage, whole genome sequences from the 1000 Genomes Project, we detect significant changes in the mutation spectrum of alleles of different ages, notably two independent changes that arose after the split of the ancestors of African and non-African populations. We also find that the mutation spectrum differs significantly between populations sampled in and outside of Africa at old polymorphisms that predate the out-of-Africa migration; this seemingly contradictory observation is likely due to mutation rate differences in remote ancestors that contributed to varying degrees to the ancestry of contemporary human populations. Finally, by relating the mutation spectrum of polymorphisms to the parental age effects on de novo mutations, we show that plausible changes in the age of reproduction over time cannot explain the joint patterns observed for different mutation types. Thus, other factors--genetic modifiers or environmental exposures--must have had a non-negligible impact on the human mutation landscape.
Visit talk page
https://simons.berkeley.edu/talks/evolution-germline-mutation-spectrum-humans
Computational Challenges in Very Large-Scale 'Omics'
Germline mutations are the source of all heritable variation. Understanding the rate and mechanisms by which mutations occur is of paramount importance for studies of human genetics (to interpret heritable disease prevalence) and evolutionary biology (to date evolutionary events). Over the past decade, there has been a flood of data in genomics––within pedigrees, among populations and across species––that is fundamentally revising our understanding of the process of mutagenesis. In my talk, I will first briefly summarize the key findings from these different datasets and then discuss recent findings investigating differences in mutation rate and spectrum (i.e., proportions of different mutation types) across human populations. To investigate inter-population differences, we developed a framework to compare polymorphisms that arose in different time windows in the past while controlling for the effects of selection and biased gene conversion. Applying this approach to high-coverage, whole genome sequences from the 1000 Genomes Project, we detect significant changes in the mutation spectrum of alleles of different ages, notably two independent changes that arose after the split of the ancestors of African and non-African populations. We also find that the mutation spectrum differs significantly between populations sampled in and outside of Africa at old polymorphisms that predate the out-of-Africa migration; this seemingly contradictory observation is likely due to mutation rate differences in remote ancestors that contributed to varying degrees to the ancestry of contemporary human populations. Finally, by relating the mutation spectrum of polymorphisms to the parental age effects on de novo mutations, we show that plausible changes in the age of reproduction over time cannot explain the joint patterns observed for different mutation types. Thus, other factors--genetic modifiers or environmental exposures--must have had a non-negligible impact on the human mutation landscape.
0:28:45
Rayan Chikhi (Institut Pasteur)
https://simons.berkeley.edu/talks/sequence-bioinformatics-large-scale-petabase-scale-sequence-alignment-catalyses-viral
Computational Challenges in Very Large-Scale 'Omics'
Petabytes of valuable sequencing data reside in public repositories, doubling in size every two years. They contain a wealth of genetic information about viruses that would help us monitor spillovers and anticipate future pandemics. We recently developed a bioinformatics cloud infrastructure, named Serratus, to perform petabase-scale sequence alignment. With it we analyzed all available RNA-seq samples (5.7 million samples, 10 petabytes) and discovered 10x more RNA viruses than previously known, including a new family of coronaviruses (Edgar et al, Nature, 2022). In this talk, I will present the computational infrastructure and some of the biological analyses.
Visit talk page
https://simons.berkeley.edu/talks/sequence-bioinformatics-large-scale-petabase-scale-sequence-alignment-catalyses-viral
Computational Challenges in Very Large-Scale 'Omics'
Petabytes of valuable sequencing data reside in public repositories, doubling in size every two years. They contain a wealth of genetic information about viruses that would help us monitor spillovers and anticipate future pandemics. We recently developed a bioinformatics cloud infrastructure, named Serratus, to perform petabase-scale sequence alignment. With it we analyzed all available RNA-seq samples (5.7 million samples, 10 petabytes) and discovered 10x more RNA viruses than previously known, including a new family of coronaviruses (Edgar et al, Nature, 2022). In this talk, I will present the computational infrastructure and some of the biological analyses.
0:32:25
Ali Mortazavi (University of California, Irvine)
https://simons.berkeley.edu/talks/long-read-transcriptome-complexity-and-cell-type-regulatory-signatures-encode4
Computational Challenges in Very Large-Scale 'Omics'
A significant proportion of mammalian genes encode for multiple transcript isoforms that result from differential promoter usage, changes in internal splicing, and 3’ end choice. The comprehensive characterization of transcript diversity across tissues, cell types, and species has been challenging because transcripts are much longer than reads normally used for RNA-seq. Long-read RNA-seq (lrRNA-seq) allows for identification of the complete structure of each transcript. As part of the final phase of the ENCODE Consortium, we sequenced 216 lrRNA-seq libraries totaling 1 billion circular consensus reads (CCS) for 60 unique human and mouse samples. We detected and quantified 94.4% of GENCODE protein coding genes as well as 42.6% of known protein coding transcripts. Overall, we detected over 100,000 full-length transcripts, one third of which are novel. We then define a new reference set of transcription start sites (TSSs), transcription end sites (TESs), and intron chains that are used for each gene across diverse tissues and cell types. Finally, we develop new metrics to characterize the transcriptional diversity of each gene in terms of alternative TSS choice, TES choice, and internal splicing; and demonstrate that this diversity varies on a per-gene basis across tissues, cell lines, and species. Our results represent the first comprehensive survey of human and mouse transcriptomes using full-length long reads and will serve as a foundation for further transcript-centric analyses. Genomic regulation after birth contributes significantly to tissue and organ maturation, but is under-studied relative to existing genomic catalogues of prenatal development in mouse. As part of ENCODE4, we generated the first comprehensive bulk and single-cell atlas of postnatal regulatory events across a diverse set of mouse tissues. The collection encompassed seven postnatal time points spanning the human equivalent of childhood through adolescence and adulthood, and focused on adrenal glands, gastrocnemius muscle, heart, hippocampus, and cortex. To allow for allele-specific analyses, we used C57BL6J/Castaneus F1 hybrid mice. Our analysis revealed novel dynamics of cell type composition including identifying new sex-specific cell populations and new commonalities in cell types shared among tissues. We also identify genomic regulatory signatures associated with dynamics of cell type composition, specialization of sub-cell types, and switching between cell states during postnatal development across 21 different cell types broken down into 68 sub-cell types. We provide an organizational framework to describe TFs that are re-purposed in regulatory signatures of cell type identity in different tissues. Together, these analyses provide a foundation for understanding the postnatal development of diverse tissues.
Visit talk page
https://simons.berkeley.edu/talks/long-read-transcriptome-complexity-and-cell-type-regulatory-signatures-encode4
Computational Challenges in Very Large-Scale 'Omics'
A significant proportion of mammalian genes encode for multiple transcript isoforms that result from differential promoter usage, changes in internal splicing, and 3’ end choice. The comprehensive characterization of transcript diversity across tissues, cell types, and species has been challenging because transcripts are much longer than reads normally used for RNA-seq. Long-read RNA-seq (lrRNA-seq) allows for identification of the complete structure of each transcript. As part of the final phase of the ENCODE Consortium, we sequenced 216 lrRNA-seq libraries totaling 1 billion circular consensus reads (CCS) for 60 unique human and mouse samples. We detected and quantified 94.4% of GENCODE protein coding genes as well as 42.6% of known protein coding transcripts. Overall, we detected over 100,000 full-length transcripts, one third of which are novel. We then define a new reference set of transcription start sites (TSSs), transcription end sites (TESs), and intron chains that are used for each gene across diverse tissues and cell types. Finally, we develop new metrics to characterize the transcriptional diversity of each gene in terms of alternative TSS choice, TES choice, and internal splicing; and demonstrate that this diversity varies on a per-gene basis across tissues, cell lines, and species. Our results represent the first comprehensive survey of human and mouse transcriptomes using full-length long reads and will serve as a foundation for further transcript-centric analyses. Genomic regulation after birth contributes significantly to tissue and organ maturation, but is under-studied relative to existing genomic catalogues of prenatal development in mouse. As part of ENCODE4, we generated the first comprehensive bulk and single-cell atlas of postnatal regulatory events across a diverse set of mouse tissues. The collection encompassed seven postnatal time points spanning the human equivalent of childhood through adolescence and adulthood, and focused on adrenal glands, gastrocnemius muscle, heart, hippocampus, and cortex. To allow for allele-specific analyses, we used C57BL6J/Castaneus F1 hybrid mice. Our analysis revealed novel dynamics of cell type composition including identifying new sex-specific cell populations and new commonalities in cell types shared among tissues. We also identify genomic regulatory signatures associated with dynamics of cell type composition, specialization of sub-cell types, and switching between cell states during postnatal development across 21 different cell types broken down into 68 sub-cell types. We provide an organizational framework to describe TFs that are re-purposed in regulatory signatures of cell type identity in different tissues. Together, these analyses provide a foundation for understanding the postnatal development of diverse tissues.
0:35:55
Ana Conesa (Spanish National Research Council)
https://simons.berkeley.edu/talks/leveraging-long-reads-sequencing-develop-functional-iso-transcriptomics-analysis-framework
Computational Challenges in Very Large-Scale 'Omics'
Post-transcriptional mechanisms such as Alternative Splicing (AS) and Alternative PolyAdenylation (APA) regulate the maturation of pre-mRNAs and may result in different transcripts arising from the same gene, increasing the diversity and regulation capacity of transcriptomes and proteomes. AS and APA has been extensively characterized at the mechanistic levels but to a lesser extent in terms of functional impact. While functional profiling is widely used to characterize the functional relevance of gene expression at the genome-wide level, similar tools at isoform resolution are missing. In contrast to short reads, single molecular sequencing technologies allow for direct sequencing of full-length transcripts, and novel tools are needed to leverage the information potential of these platforms to study the functional consequences of alternative transcript processing. Particularly, RNA sequencing using long reads technologies results in a vast number of novel transcripts that are a mixture of representations of true molecules and technology artifacts. Additionally, functional annotation at isoform resolution has not been developed yet. Here we present a novel computational framework for Functional Iso-Transcriptomics analysis (FIT), specially designed to study isoform (differential) expression from a functional perspective. This framework consists of three bioinformatics developments. SQANTI is used to define and curate expressed transcriptomes obtained with long-read technologies. SQANTI categorizes full-length reads, evaluates their potential biases, and removes low-quality instances. The IsoAnnot pipeline combines multiple databases and function prediction algorithms to return a rich isoform-level annotation file of functional domains, motifs, and sites, both coding and non-coding. Finally, the tappAS software introduces novel analysis methods to interrogate the functional relevance of isoform complexity. I will show the application of the FIT framework to the analysis of differentiating mouse neural cells.
Visit talk page
https://simons.berkeley.edu/talks/leveraging-long-reads-sequencing-develop-functional-iso-transcriptomics-analysis-framework
Computational Challenges in Very Large-Scale 'Omics'
Post-transcriptional mechanisms such as Alternative Splicing (AS) and Alternative PolyAdenylation (APA) regulate the maturation of pre-mRNAs and may result in different transcripts arising from the same gene, increasing the diversity and regulation capacity of transcriptomes and proteomes. AS and APA has been extensively characterized at the mechanistic levels but to a lesser extent in terms of functional impact. While functional profiling is widely used to characterize the functional relevance of gene expression at the genome-wide level, similar tools at isoform resolution are missing. In contrast to short reads, single molecular sequencing technologies allow for direct sequencing of full-length transcripts, and novel tools are needed to leverage the information potential of these platforms to study the functional consequences of alternative transcript processing. Particularly, RNA sequencing using long reads technologies results in a vast number of novel transcripts that are a mixture of representations of true molecules and technology artifacts. Additionally, functional annotation at isoform resolution has not been developed yet. Here we present a novel computational framework for Functional Iso-Transcriptomics analysis (FIT), specially designed to study isoform (differential) expression from a functional perspective. This framework consists of three bioinformatics developments. SQANTI is used to define and curate expressed transcriptomes obtained with long-read technologies. SQANTI categorizes full-length reads, evaluates their potential biases, and removes low-quality instances. The IsoAnnot pipeline combines multiple databases and function prediction algorithms to return a rich isoform-level annotation file of functional domains, motifs, and sites, both coding and non-coding. Finally, the tappAS software introduces novel analysis methods to interrogate the functional relevance of isoform complexity. I will show the application of the FIT framework to the analysis of differentiating mouse neural cells.
0:32:6
Ron Shamir (Tel Aviv University)
https://simons.berkeley.edu/talks/multi-omic-integration-understanding-disease
Computational Challenges in Very Large-Scale 'Omics'
The availability of large multi-modal biological datasets invites researchers to deepen our understanding in basic science and medicine, with the goal of personalized analysis. While inquiry of each data type separately often provides insights, integrative analysis has the potential to reveal more holistic, systems-level findings. We demonstrate the power of integrated analysis in disease by developing algorithms on several levels, including subtyping based on multiple omics for the same cancer; predicting one omic based on another; and predicting a healthy individual’s future risk of developing cancer based on data from routine periodical checkups.
Visit talk page
https://simons.berkeley.edu/talks/multi-omic-integration-understanding-disease
Computational Challenges in Very Large-Scale 'Omics'
The availability of large multi-modal biological datasets invites researchers to deepen our understanding in basic science and medicine, with the goal of personalized analysis. While inquiry of each data type separately often provides insights, integrative analysis has the potential to reveal more holistic, systems-level findings. We demonstrate the power of integrated analysis in disease by developing algorithms on several levels, including subtyping based on multiple omics for the same cancer; predicting one omic based on another; and predicting a healthy individual’s future risk of developing cancer based on data from routine periodical checkups.
0:36:11
Roderic Guigó (Center for Genomic Regulation)
https://simons.berkeley.edu/talks/multi-omic-executable-networks-mechanisms-clinical-applications-cancer
Computational Challenges in Very Large-Scale 'Omics'
Histone modifications are widely accepted to play a causal role in the regulation of gene expression. This role has been recently challenged, however, by reports showing that gene expression may occur in the absence of histone modifications. To address this controversy, we have generated densely-spaced transcriptomic and epigenomic maps in a time-course cell homogeneous system that occurs with massive transcriptional changes. We found that the relationship between histone modifications and gene expression is weaker than previously reported, and that it can even run contrary to established assumptions, such as in the case of H3K9me3. Our data suggest a model that reconciles the seemingly contradictory observations in the field. According to this model, histone modifications are associated with expression only at the time of initial gene activation, when they are deposited in a dominant order at promoter regions, generally preceding deposition at enhancers. Further changes in gene expression, even larger than those occurring at gene activation, are essentially uncoupled from changes in histone modifications. Genes are in a very limited number of major chromatin states which mostly remain stable over time. Data available during mouse development largely recapitulates this model. Our work provides a first sketch of the epigenetic logic underlying gene activation in eukaryotic cells.
Visit talk page
https://simons.berkeley.edu/talks/multi-omic-executable-networks-mechanisms-clinical-applications-cancer
Computational Challenges in Very Large-Scale 'Omics'
Histone modifications are widely accepted to play a causal role in the regulation of gene expression. This role has been recently challenged, however, by reports showing that gene expression may occur in the absence of histone modifications. To address this controversy, we have generated densely-spaced transcriptomic and epigenomic maps in a time-course cell homogeneous system that occurs with massive transcriptional changes. We found that the relationship between histone modifications and gene expression is weaker than previously reported, and that it can even run contrary to established assumptions, such as in the case of H3K9me3. Our data suggest a model that reconciles the seemingly contradictory observations in the field. According to this model, histone modifications are associated with expression only at the time of initial gene activation, when they are deposited in a dominant order at promoter regions, generally preceding deposition at enhancers. Further changes in gene expression, even larger than those occurring at gene activation, are essentially uncoupled from changes in histone modifications. Genes are in a very limited number of major chromatin states which mostly remain stable over time. Data available during mouse development largely recapitulates this model. Our work provides a first sketch of the epigenetic logic underlying gene activation in eukaryotic cells.
0:29:40
Yana Safonova (Johns Hopkins University)
https://simons.berkeley.edu/talks/profiling-antibody-repertoires-and-immunoglobulin-loci-enables-large-scale-analysis-adaptive
Computational Challenges in Very Large-Scale 'Omics'
Twelve years ago, biologists developed the repertoire sequencing technology (Rep-seq) that samples millions out of a billion constantly changing antibodies (or immunoglobulins) circulating in each of us. Repertoire sequencing represented a paradigm shift as compared to the previous “one-antibody-at-a-time” approaches, raised novel algorithmic, statistical, information theory, and machine learning challenges, and led to the emergence of computational immunogenomics. In addition to that, recent improvements of whole genome sequencing (WGS) technologies and assembly methods have resulted in nearly complete assemblies of germline immunoglobulin loci for various vertebrate species. Together, Rep-Seq and WGS opened new avenues for studying adaptive immune systems. In this talk, I will describe our recent immunogenomics work on finding disease-specific features of antibody repertoires in humans and non-human species using Rep-Seq and WGS data.
Visit talk page
https://simons.berkeley.edu/talks/profiling-antibody-repertoires-and-immunoglobulin-loci-enables-large-scale-analysis-adaptive
Computational Challenges in Very Large-Scale 'Omics'
Twelve years ago, biologists developed the repertoire sequencing technology (Rep-seq) that samples millions out of a billion constantly changing antibodies (or immunoglobulins) circulating in each of us. Repertoire sequencing represented a paradigm shift as compared to the previous “one-antibody-at-a-time” approaches, raised novel algorithmic, statistical, information theory, and machine learning challenges, and led to the emergence of computational immunogenomics. In addition to that, recent improvements of whole genome sequencing (WGS) technologies and assembly methods have resulted in nearly complete assemblies of germline immunoglobulin loci for various vertebrate species. Together, Rep-Seq and WGS opened new avenues for studying adaptive immune systems. In this talk, I will describe our recent immunogenomics work on finding disease-specific features of antibody repertoires in humans and non-human species using Rep-Seq and WGS data.
0:29:51
Haiyan Huang (UC Berkeley)
https://simons.berkeley.edu/talks/leveraging-molecular-data-drug-discovery
Computational Challenges in Very Large-Scale 'Omics'
As the cost of genomic technologies continues to decrease and large scale profiling of molecular features in diseases and their changing expression after exposure to drugs becomes more readily available, leveraging molecular data for drug discovery becomes increasingly more important. Existing studies largely rely on the belief that drugs which reverse the expression of disease associated genes have potential to be efficacious for treating the disease in question, and thus statistics that can effectively summarize this reversal relationship are in high demand. We propose a rank based count statistic for detecting such reversal relationships. This statistic is robust to outliers and we have derived results concerning its asymptotic behavior. We also propose a gene-level statistic for detecting potential drug-target genes. In simulation studies and real data we see that our statistics are comparable to or outperform other measures in these scenarios.
Visit talk page
https://simons.berkeley.edu/talks/leveraging-molecular-data-drug-discovery
Computational Challenges in Very Large-Scale 'Omics'
As the cost of genomic technologies continues to decrease and large scale profiling of molecular features in diseases and their changing expression after exposure to drugs becomes more readily available, leveraging molecular data for drug discovery becomes increasingly more important. Existing studies largely rely on the belief that drugs which reverse the expression of disease associated genes have potential to be efficacious for treating the disease in question, and thus statistics that can effectively summarize this reversal relationship are in high demand. We propose a rank based count statistic for detecting such reversal relationships. This statistic is robust to outliers and we have derived results concerning its asymptotic behavior. We also propose a gene-level statistic for detecting potential drug-target genes. In simulation studies and real data we see that our statistics are comparable to or outperform other measures in these scenarios.
0:28:26
Alejandra Medina-Rivera (Universidad Nacional Autónoma de México)
https://simons.berkeley.edu/talks/rewards-and-challenges-constructing-patient-registries-mexico
Computational Challenges in Very Large-Scale 'Omics'
Electronic Health Record Information Systems set the mechanisms to record, exchange and consolidate information, however, the diversity of electronic clinical records and database storage systems in Mexico greatly hinders data sharing for research. This is one of the main challenges for Large Scale Omics projects in the country, as each hospital, insurance service (private, public, military), and region will have their own electronic system that can also change across administrations. Patient registries are an essential tool in public health research, by providing population-based information for understanding disease etiology, course, incidence and to evaluate treatments, life quality and assess patients needs, among others. In past years, patient registries independent of the government health systems have been established in Mexico with valuable results. In particular, we launched three nationwide registries in order to retrieve epidemiological and genetic information of twins (TwinsMX), people with Systemic Lupus Erythematosus (Lupus RGMX) and patients with Parkinson Disease (MEX-PD). These are designed to obtain data from electronic questionnaires and genetic testing and aim to expand our knowledge of the phenotypic and genetics of these traits in admixed populations. In the case of TwinsMX and LupusRGMX participants are mainly recruited through social media, while MEX-PD requires patients to be registered by their neurologists. Surveys are available on web-based application Research Electronic Data Capture (REDCap), which allows secure capture and storage of data, and most surveys are shared across registries to facilitate data integration for future projects. For all registries the participation of patient communities is key for the advancement of the registries, we strongly believe that the participants are not subjects of study, but active citizens with a major role in biomedical research. TwinsMX, Lupus RGMX and MEX-PD will allow us to characterize the genetic and environmental contribution over different traits and diseases with data directly collected in the Mexican population (which is heavily underrepresented in epidemiological studies). This characterization will provide relevant information that could in the future be useful to guide research to improve treatments.
Visit talk page
https://simons.berkeley.edu/talks/rewards-and-challenges-constructing-patient-registries-mexico
Computational Challenges in Very Large-Scale 'Omics'
Electronic Health Record Information Systems set the mechanisms to record, exchange and consolidate information, however, the diversity of electronic clinical records and database storage systems in Mexico greatly hinders data sharing for research. This is one of the main challenges for Large Scale Omics projects in the country, as each hospital, insurance service (private, public, military), and region will have their own electronic system that can also change across administrations. Patient registries are an essential tool in public health research, by providing population-based information for understanding disease etiology, course, incidence and to evaluate treatments, life quality and assess patients needs, among others. In past years, patient registries independent of the government health systems have been established in Mexico with valuable results. In particular, we launched three nationwide registries in order to retrieve epidemiological and genetic information of twins (TwinsMX), people with Systemic Lupus Erythematosus (Lupus RGMX) and patients with Parkinson Disease (MEX-PD). These are designed to obtain data from electronic questionnaires and genetic testing and aim to expand our knowledge of the phenotypic and genetics of these traits in admixed populations. In the case of TwinsMX and LupusRGMX participants are mainly recruited through social media, while MEX-PD requires patients to be registered by their neurologists. Surveys are available on web-based application Research Electronic Data Capture (REDCap), which allows secure capture and storage of data, and most surveys are shared across registries to facilitate data integration for future projects. For all registries the participation of patient communities is key for the advancement of the registries, we strongly believe that the participants are not subjects of study, but active citizens with a major role in biomedical research. TwinsMX, Lupus RGMX and MEX-PD will allow us to characterize the genetic and environmental contribution over different traits and diseases with data directly collected in the Mexican population (which is heavily underrepresented in epidemiological studies). This characterization will provide relevant information that could in the future be useful to guide research to improve treatments.
0:31:26
Eran Halperin (Optum Labs and UCLA)
https://simons.berkeley.edu/talks/whole-genome-methylation-patterns-biomarkers-ehr-imputation
Computational Challenges in Very Large-Scale 'Omics'
Diagnosis and prediction of health outcomes using machine learning has shown major advances over the last few years. One of the major challenges remaining is the sparsity of electronic health records data, which often requires an imputation step. Genomic data can potentially be used to improve our ability for imputation; indeed, polygenic risk scores using genetic data have been heavily studied in the past few years. In this talk, I will present approaches for imputation using DNA methylation, and compare those predictions to polygenic risk scores and to traditional EHR imputation approaches.
Visit talk page
https://simons.berkeley.edu/talks/whole-genome-methylation-patterns-biomarkers-ehr-imputation
Computational Challenges in Very Large-Scale 'Omics'
Diagnosis and prediction of health outcomes using machine learning has shown major advances over the last few years. One of the major challenges remaining is the sparsity of electronic health records data, which often requires an imputation step. Genomic data can potentially be used to improve our ability for imputation; indeed, polygenic risk scores using genetic data have been heavily studied in the past few years. In this talk, I will present approaches for imputation using DNA methylation, and compare those predictions to polygenic risk scores and to traditional EHR imputation approaches.