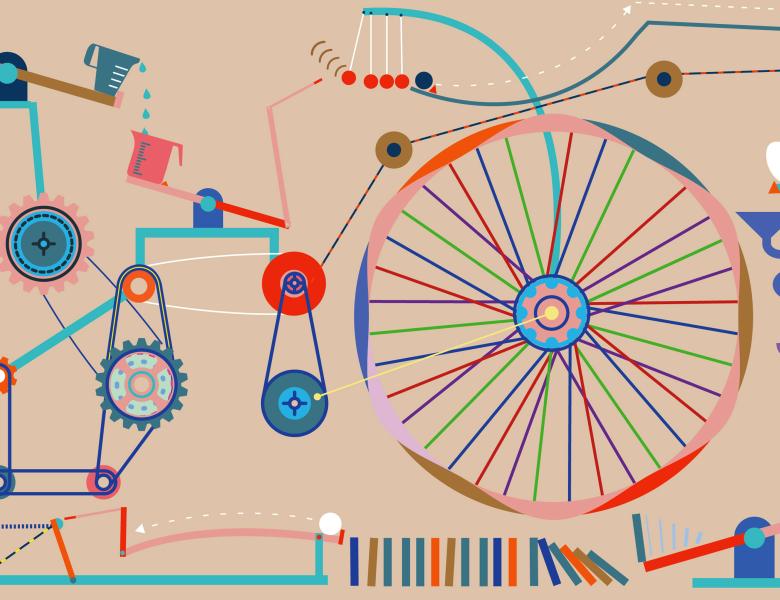
Abstract
Causal inference plays a key role in many scientific disciplines. Often the underlying causal graph is unknown and needs to be estimated from data. In this context, one fundamental difficulty is that causal models can generally not be fully identified based on observational data only. Interventional data, that is, data originating from different experimental environments, improves identifiability. By exploiting the invariance of the causal model the method “Invariant Causal Prediction” (Peters et al., 2016) exploits data from different environments to estimate the causal parents of a response variable of interest. However, the improvement in identifiability compared to the observational setting depends critically on the target and nature of the interventions carried out in each experiment. Since in real applications experiments tend to be costly, there is a need to perform the right interventions such that as few as possible are required. In this talk, I will present new active learning (i.e. experiment selection) strategies based on causal invariance, which select intervention targets that quickly reveal the direct causes of a response variable of interest in the causal graph.