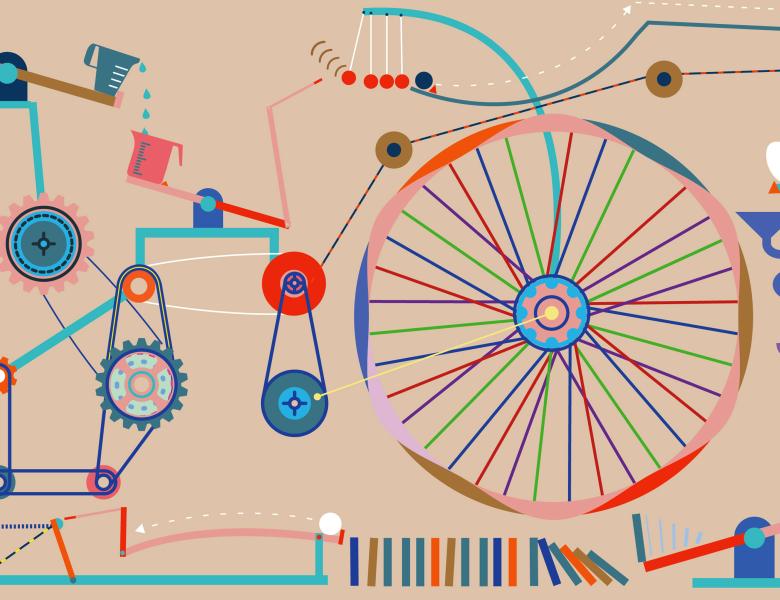
Abstract
In this talk, I'll discuss a couple of recent efforts that apply causal inference to study the effects of algorithmic interventions on digital platforms, specifically, the Twitter platform. The first work is an extensive audit of the algorithmic amplification of political content on Twitter based on a long-running, massive-scale randomized experiment. I'll focus on what the study design shows and what it cannot show. While some challenges, such as network effects and interference, are widely recognized, others are more subtle. The second work is about the difficulty of making causal inferences based on user choices on the platform. While user choices gives rise to a host of intriguing observational designs, I'll explain why these may be inherently difficult to deconfound. Based on these two studies, I'll discuss some shortcomings of causal inference in practice and avenues for future work. The first part is joint work with Ferenc Huszár, Sofia Ira Ktena, Conor O’Brien, Luca Belli, and Andrew Schlaikjer. The second is joint work with Smitha Milli and Luca Belli.