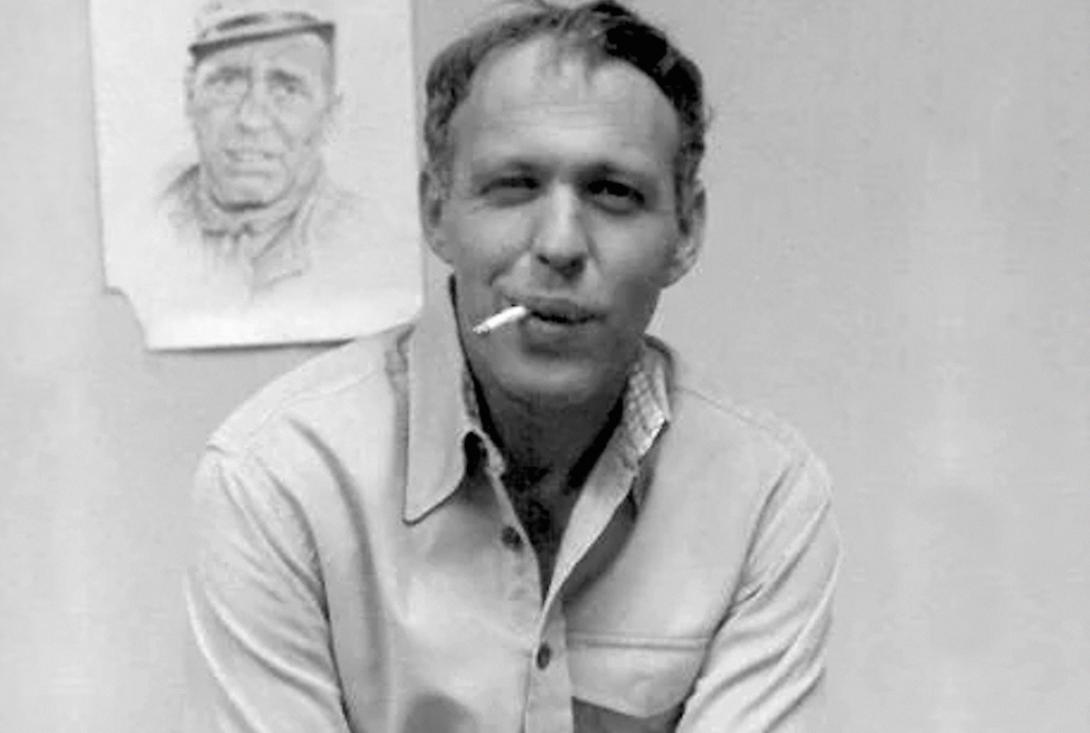
Appreciation and memories of Jim Simons (1938–2024), from the Simons Institute community. Featuring contributions from Avi Wigderson, Dick Karp, Shafi...
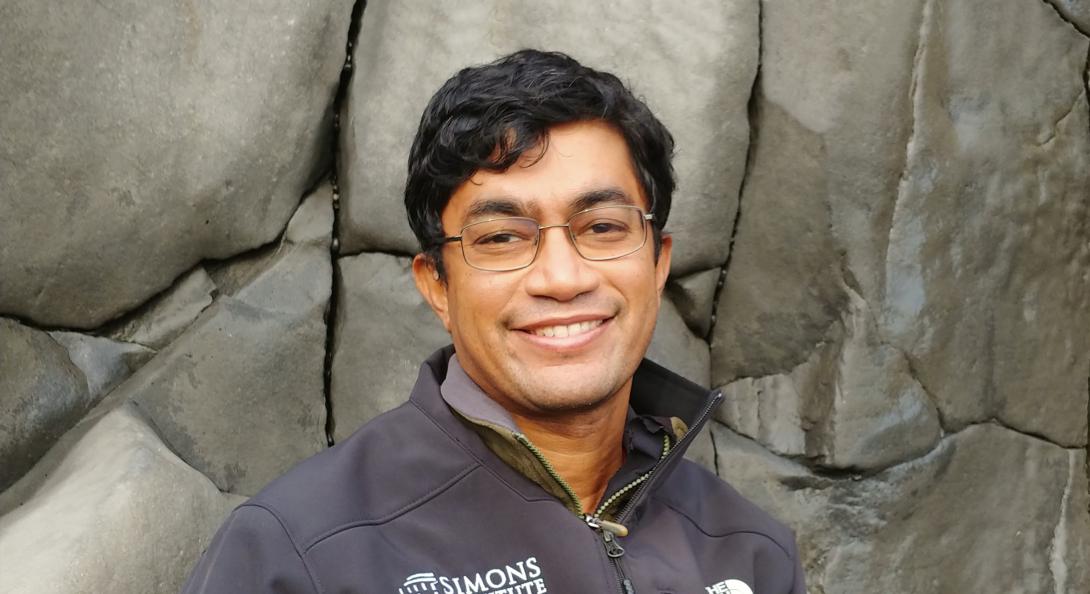
Simons Institute Senior Scientist Venkatesan Guruswami, along with Bingkai Lin, Yican Sun, and Berkeley theory graduate students Xuandi Ren and Kewen...
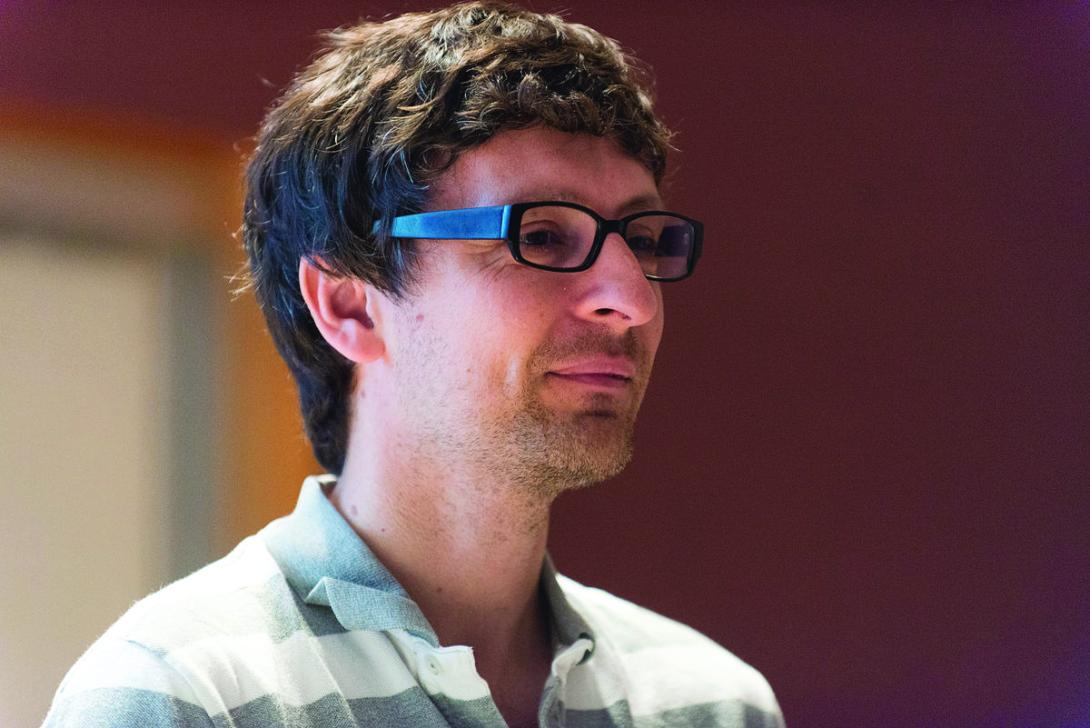
We are heartbroken by the loss of Luca Trevisan, who served as senior scientist at the Institute from 2014 to 2019.
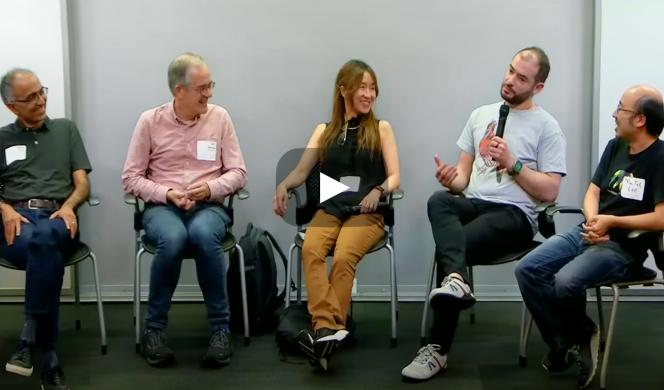
On the first day of the workshop on Large Language Models and Transformers, Alexei Efros (UC Berkeley) moderated a panel that addressed a range of topics, including the future of LLMs, memorization vs. generalization, and novelty and creativity. Featuring Sanjeev Arora (Princeton University), Chris Manning (Stanford), Yejin Choi (University of Washington), Ilya Sutskever (OpenAI), and Yin Tat Lee (University of Washington and Microsoft Research).
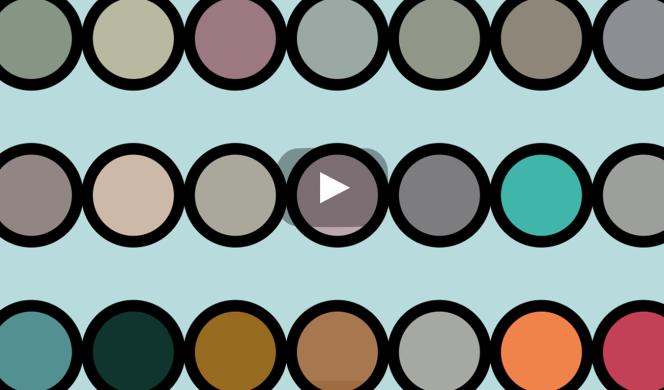
This month, we are highlighting three presentations from our August 2023 workshop on Large Language Models and Transformers. In this talk, OpenAI cofounder and chief scientist Ilya Sutskever presents a theory of unsupervised learning.
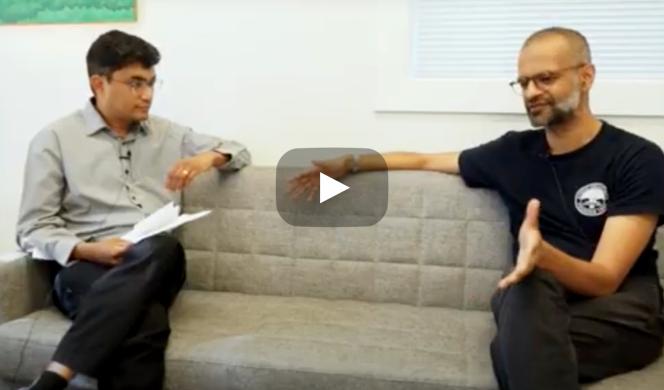
In July 2023, Nikhil Srivastava joined the Simons Institute as interim senior scientist. And with Shafi Goldwasser on sabbatical for the fall semester, Venkatesan Guruswami is serving as acting interim director through December 2023. The pair sat down last month to discuss their new roles, Nikhil’s research, and the intersections of math and TCS.
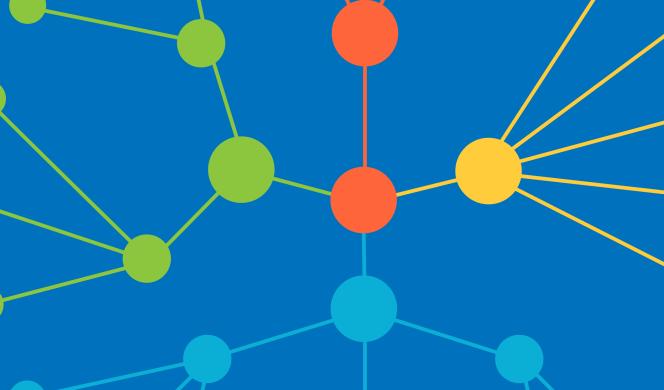
“The workshop atmosphere was thick with expectation and excitement,” said UC Berkeley’s Alexei Efros, comparing it to what might have been the mood at another epochal moment in scientific history — the development of quantum physics in the early 1900s. “I imagine that a gathering of physicists at the dawn of the 20th century might have felt similar — everyone sensed that something big was coming, but it wasn’t quite clear what.”

Peter Bartlett has received the UC Berkeley Chancellor’s Distinguished Service Award, in recognition of his exceptional contributions to the Simons Institute for the Theory of Computing at UC Berkeley, where he served as associate director from 2017 to 2022.
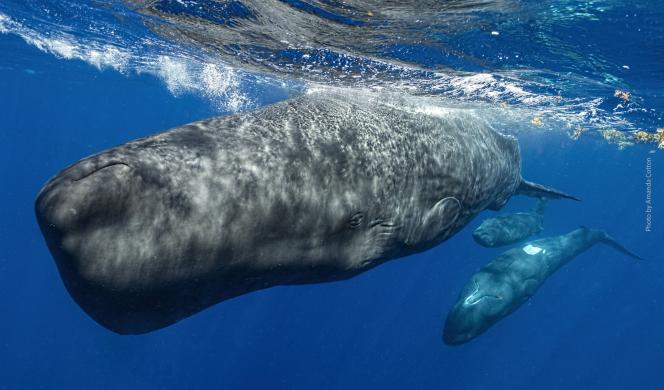
Three years ago, in August 2020, the Simons Institute co-hosted a workshop on Decoding Communication in Nonhuman Species. Looking back at the titles of the talks, none used the AI buzzwords that have become household terms in the course of the last several months: ChatGPT, large language models, generative AI, chatbots. Yet the technology these terms refer to is central to the task at hand. The idea of the workshop was to apply cutting-edge methods from the field of natural language processing, especially large language models, to animal communication. This past June, the Institute co-hosted a follow-up workshop, Decoding Communication in Nonhuman Species II. Now that the technology is advancing at a breathtaking pace, this second workshop afforded participants an opportunity to take a look at how these efforts have advanced in the last three years — and whether the field has produced new tools that can help researchers understand what animals are talking about.
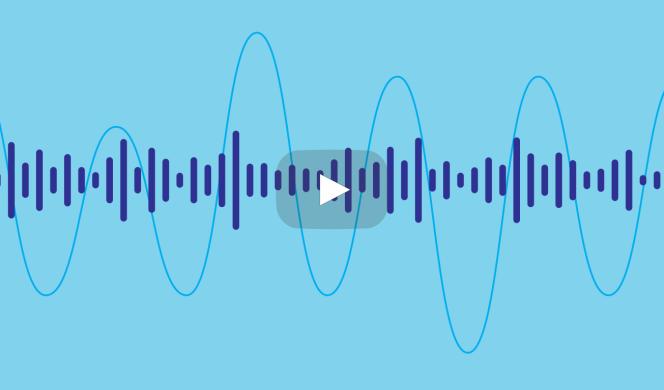
In this talk from the recent workshop on Decoding Communication in Nonhuman Species, Bryan Pardo (Northwestern) presents work applying iterative decoding and acoustic token modeling to music audio synthesis. The outputs of this procedure can range from a high-quality audio compression technique to variations on the original input music that match the original input music in terms of style, genre, beat and instrumentation, while varying specifics of timbre and rhythm.

Nima Anari (Stanford) and collaborators obtain the first polylogarithmic-time sampling algorithms for determinantal point processes, directed Eulerian tours, and more.

In a series of talks in the boot camp for this summer's program on Analysis and TCS, Dor Minzer (MIT) surveyed recent developments in PCPs fueled by hyper-contractive estimates for global functions that are not significantly affected by a restriction of a small set of coordinates.