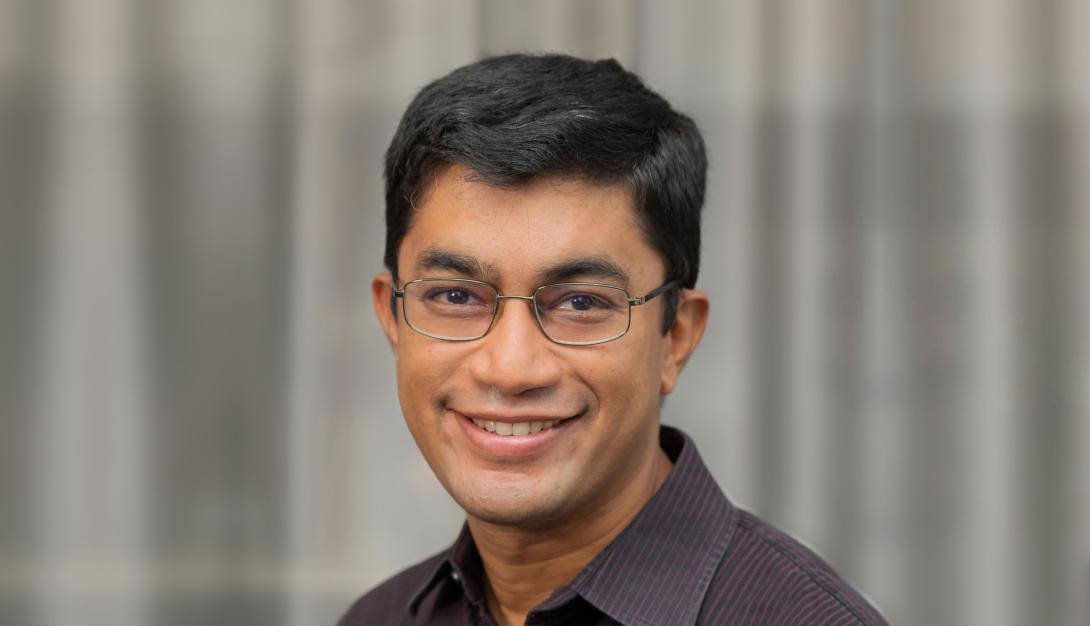
Greetings from Berkeley, where we’ve recently welcomed a merry band of cryptographers for what promises to be an outstanding summer program on...
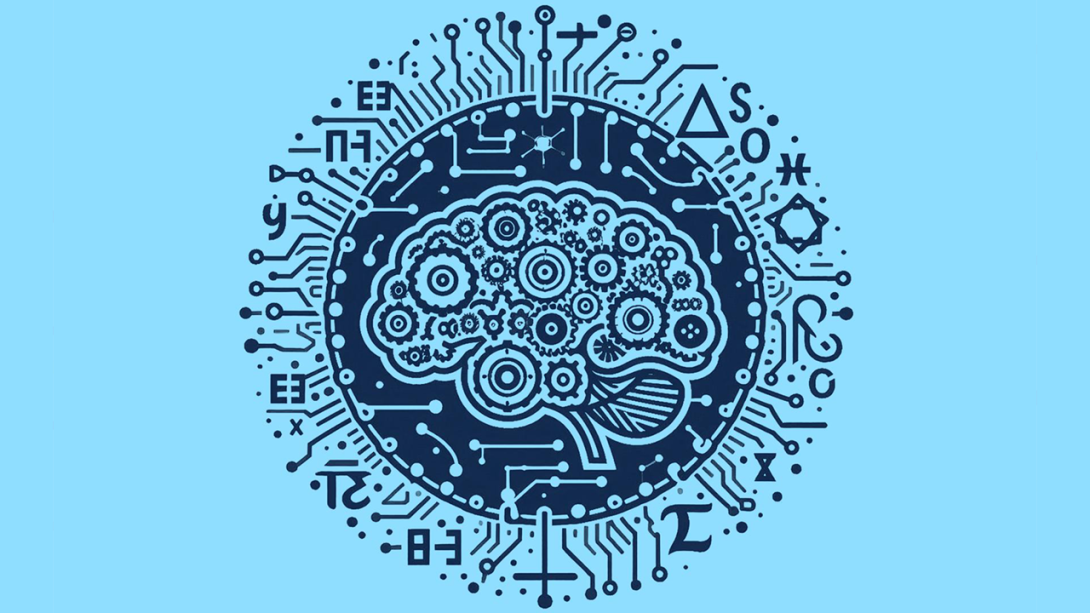
This month, we held a joint workshop with SLMath on AI for Mathematics and Theoretical Computer Science. It was unlike any other Simons Institute...
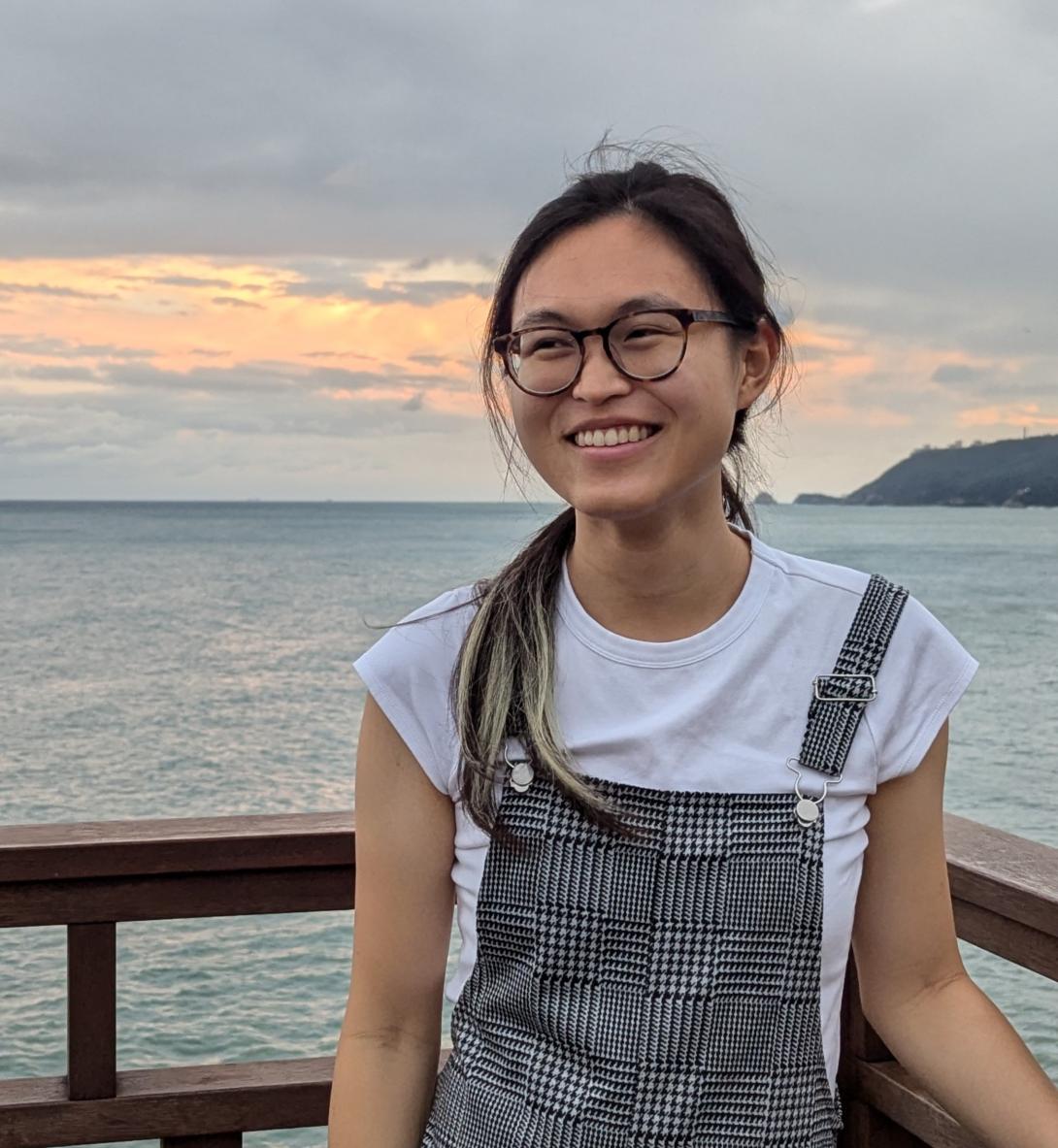
We’re delighted to share that Miller fellow and Simons Institute Quantum Pod postdoc Ewin Tang has been awarded the 2025 Maryam Mirzakhani New...

Greetings from Berkeley, where the spring semester is just getting underway. This term, we are hosting the second half of the Special Year on Large Language Models and Transformers. This program explores LLMs from a broad perspective, asking questions about their power, scalability, trustworthiness, safety, implications for research in the sciences, and the legal frameworks in which LLMs operate, as well as their impacts on society at large.
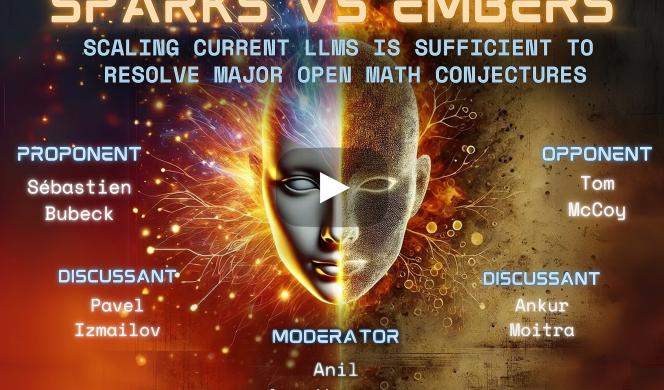
As part of the Simons Institute’s recent workshop on Unknown Futures of Generalization, we hosted a debate probing the unknown generalization limits of current LLMs. The debate was between Sébastien Bubeck (OpenAI), a coauthor of “Sparks of Artificial General Intelligence,” and Tom McCoy (Yale), a coauthor of “Embers of Autoregression,” and it addressed the motion “Current LLM scaling methodology is sufficient to generate new proof techniques needed to resolve major open mathematical conjectures such as P ≠ NP.”
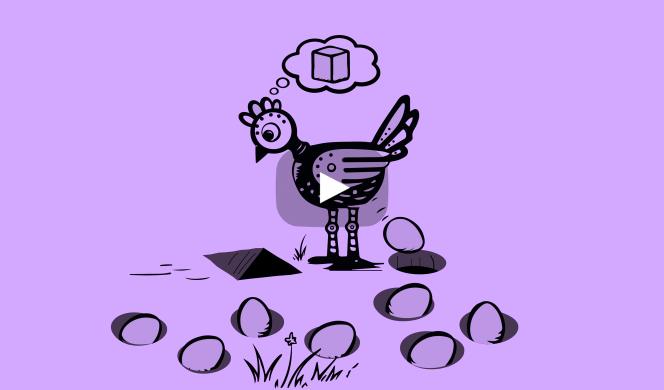
To survive in complex three-dimensional environments — and to behave effectively in unknown future settings — animals must possess the ability to represent and manipulate geometric representations of the world. Bruno Olshausen (UC Berkeley) and collaborators have been exploring the neural computations and representations that could underlie such abilities.
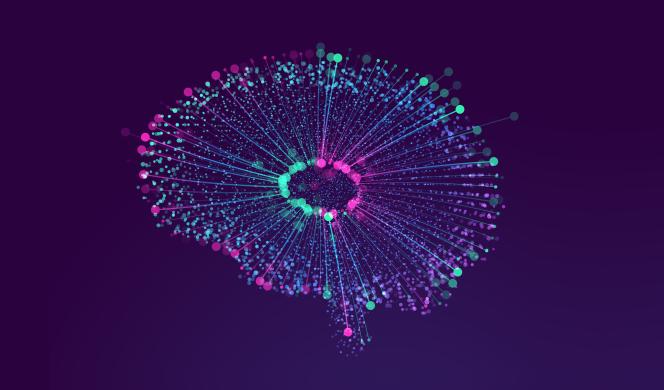
The New York Public Library sells a magnet printed with words by the American author Fran Lebowitz: “Think before you speak. Read before you think.” OpenAI’s latest offering — the o1 suite of large language models — seems to be taking this appeal to heart. The models, according to OpenAI, are “designed to spend more time thinking before they respond.” This allows the models to be more effective when trying to solve complex problems that require them to reason.

In his Richard M. Karp Distinguished Lecture last month, Sasha Rush (Cornell Tech) introduced the literature related to test-time compute and model self-improvement, and discussed the expected implications of test-time scaling. This talk also briefly connected these research directions to current open-source efforts to build effective reasoning models.

In this recent talk from the workshop on Transformers as a Computational Model, Noam Brown (OpenAI) describes OpenAI’s new o1 model, an LLM trained via reinforcement learning to generate a hidden chain of thought before its response.

Greetings from the land of the Ohlone. As the year draws to a close, I am grateful for all of you who make up our brilliant, innovative, open-minded, and collaborative community, and for my wonderful colleagues at the Simons Institute who have made Calvin Lab a global home for our field.

Greetings from Berkeley, where we have a busy week ahead, including our flagship Industry Day on Thursday, November 7. And we’re pleased to announce three exciting new initiatives.

In this talk from the recent workshop on Alignment, Trust, Watermarking, and Copyright Issues in LLMs, Nicholas Carlini (Google DeepMind) introduces two attacks that cause ChatGPT to emit megabytes of data it was trained on from the public internet. In the first attack, they ask ChatGPT to emit the same word over and over ("Say 'poem poem poem...' forever") and find that this causes it to diverge, and that when it diverges, it frequently outputs text copied directly from the pretraining data. The second attack is much stronger, and shows how to break the model's alignment by exploiting a fine-tuning API, allowing the researchers to "undo" the safety fine-tuning.

We are delighted to announce an opportunity for researchers to propose workshops to be held at the Simons Institute, as part of two newly established workshop series: Goldwasser Exploratory Workshops and Breakthroughs Workshops.