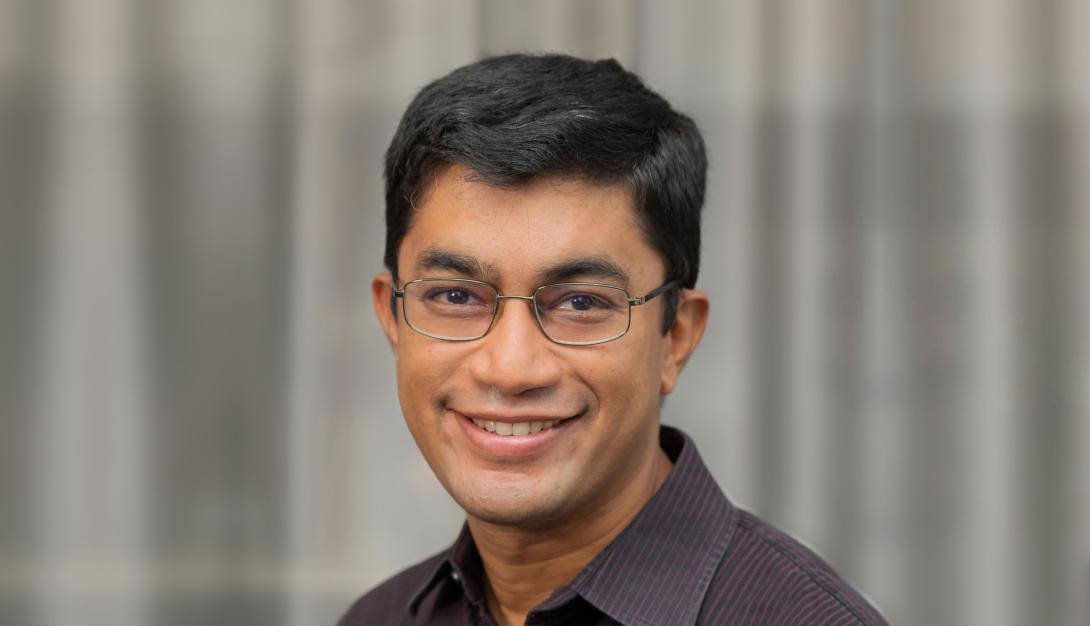
Greetings from Berkeley, where we’ve recently welcomed a merry band of cryptographers for what promises to be an outstanding summer program on...
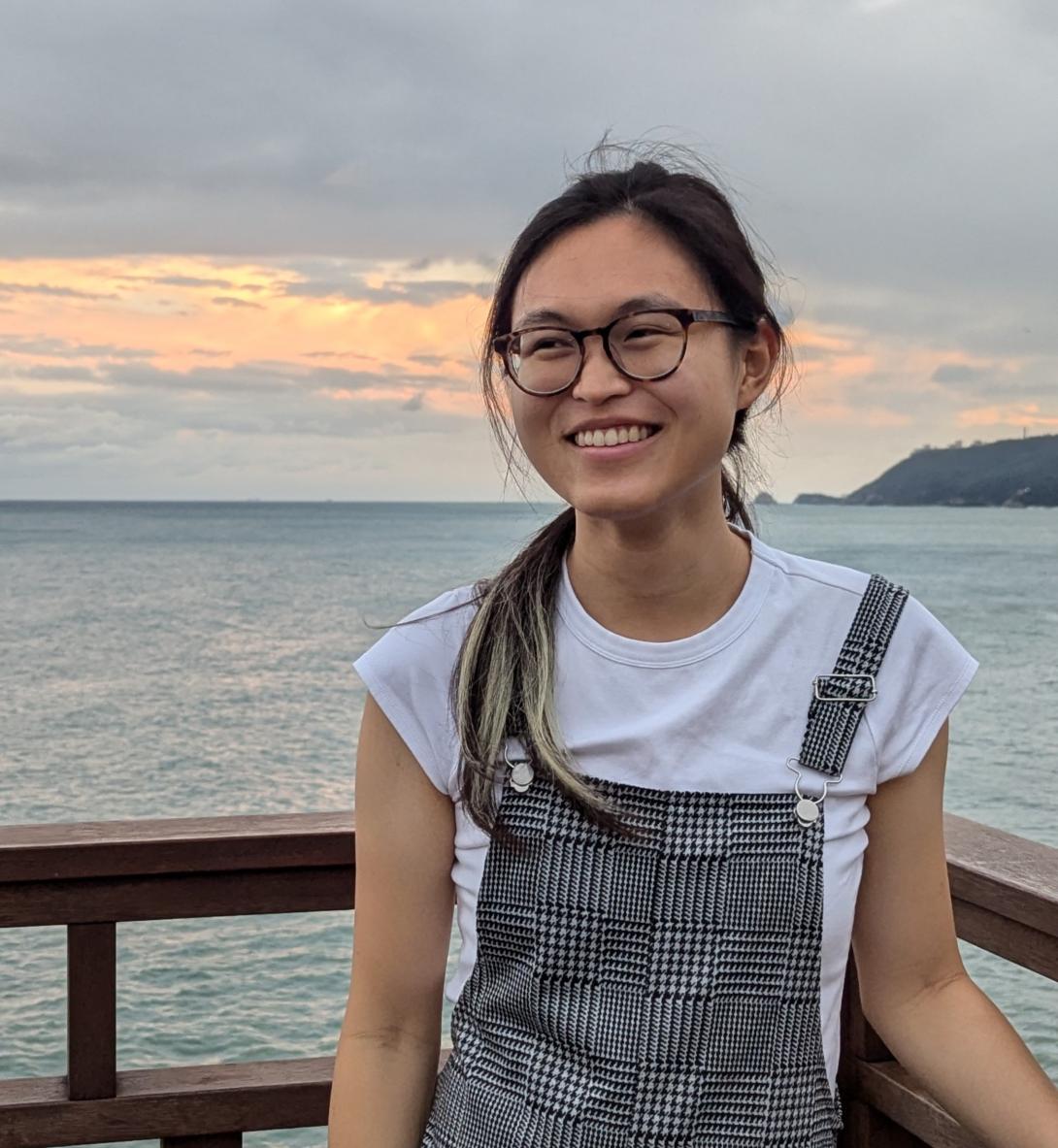
We’re delighted to share that Miller fellow and Simons Institute Quantum Pod postdoc Ewin Tang has been awarded the 2025 Maryam Mirzakhani New...
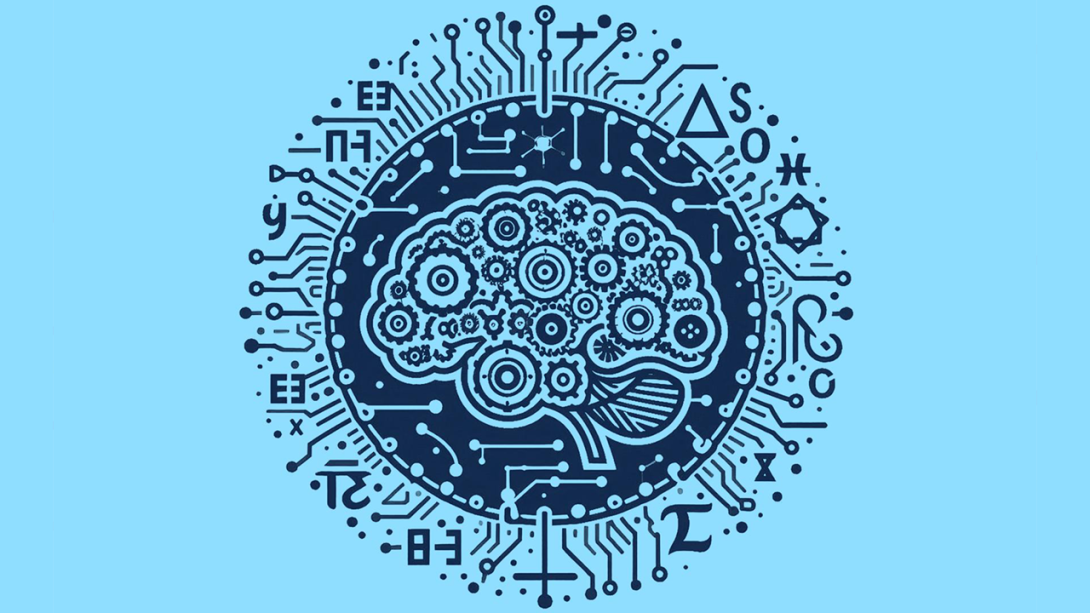
This month, we held a joint workshop with SLMath on AI for Mathematics and Theoretical Computer Science. It was unlike any other Simons Institute...
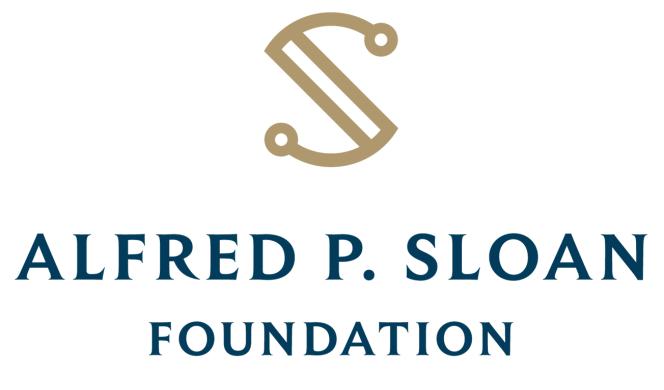
“Every traditional statistics text includes a warning not to mistake correlation for causality. But until very recently, there has been almost nothing else to say about causality,” observes Daniel Goroff, director of the Sloan Foundation’s economics program. “Research on the theory and practice of causal inference, as facilitated by the Simons Institute, will be critical to progress on everything from machine learning to evidence-based policymaking.”
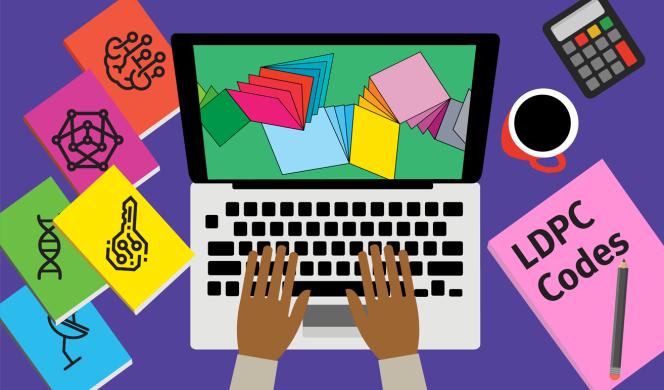
It has been only a few months since the last time this column appeared. Yet there is so much to write about that it feels like a year has passed. For one area in particular, a decade’s worth of developments seems to have emerged in a few months’ time. I am talking of course about the phenomenal developments on error-correcting codes that we witnessed in the past few months.
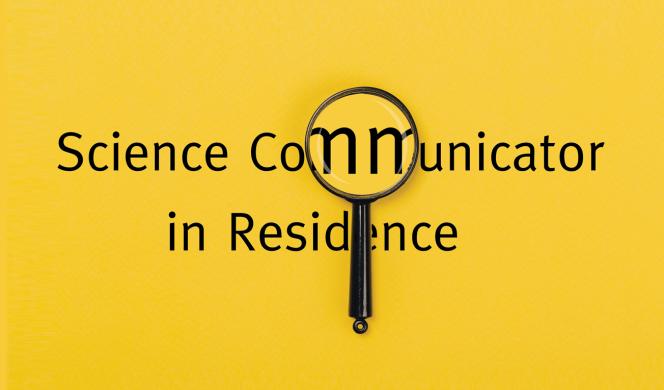
The Simons Institute is currently welcoming applications for Science Communicator in Residence slots for Fall 2022 and Spring 2023. The application deadline has been extended to March 31.
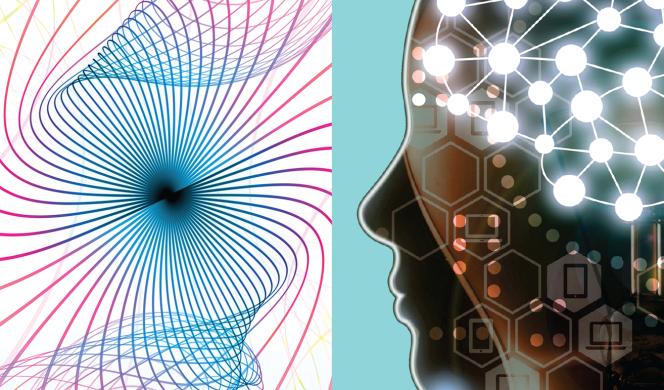
The Simons Institute’s Research Pod in Quantum Computing invites applications for postdoctoral fellows and visiting research scientists for the 2022–23 academic year.

Venkatesan Guruswami joined the Simons Institute as our newest senior scientist on January 1. We hope you enjoy our conversation with Venkat about his visions for the field and for the Simons Institute.
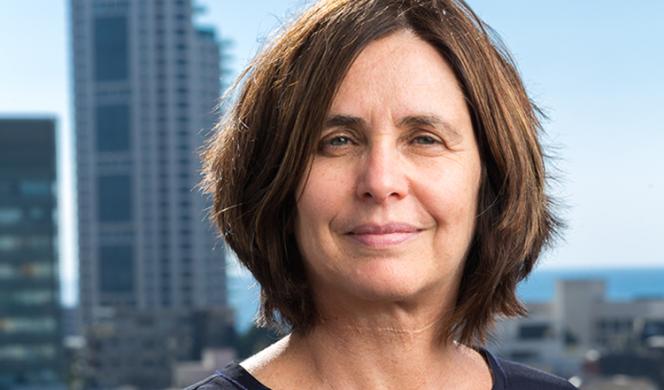
Collaborative research is proceeding apace in Calvin Lab, amid the various health and safety regulations. This semester we’re hosting 136 long-term visitors in our Causality and Learning and Games programs, and we look forward to a vibrant semester with them. Omicron willing, we are hoping for blue skies, and many workshops and results in the months to come.

Guruswami's research interests span many areas of theoretical computer science and related mathematics, including coding theory, approximate optimization, randomness in computing, and computational complexity.
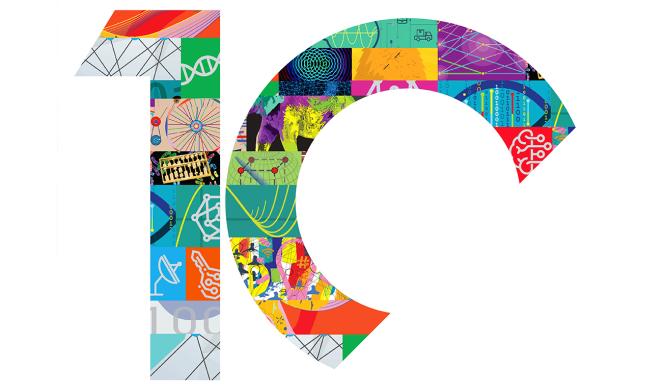
This fall, we embarked on the 10th year since the founding of the Simons Institute on July 1, 2012. Throughout the year, we will be celebrating the vision of Jim and Marilyn Simons, our founding benefactors, as well as the lasting friendships, breakthrough research, and interdisciplinary synergies that have been created over the years at the Institute that bears their name.