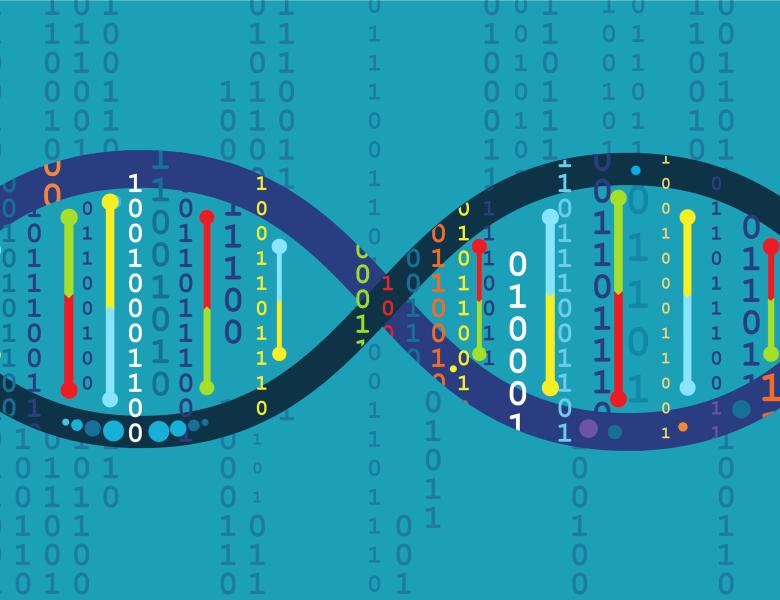
Playlist: 22 videos
From Algorithms to Discovery in Genome-Scale Biology and Medicine
0:46:56
Shyam Prabhakar (Genome Institute of Singapore)
https://simons.berkeley.edu/talks/tbd-443
From Algorithms to Discovery in Genome-Scale Biology and Medicine
Each cell type in a solid tissue has a characteristic transcriptome and spatial arrangement, both of which are observable using modern spatial omics assays. Surprisingly however, spatial information is frequently ignored when clustering cells to identify cell types and states. In fact, spatial location is typically considered only when solving the related, but distinct, problem of demarcating tissue domains (which could include multiple cell types). We present BANKSY, an algorithm that unifies cell type clustering and domain segmentation by constructing a product space of cell and neighborhood transcriptomes, representing cell state and microenvironment, respectively. BANKSY's spatial kernel-based feature augmentation strategy improves performance on both tasks when tested on diverse FISH- and sequencing-based spatial omics datasets. Uniquely, BANKSY identified hitherto undetected niche-dependent cell states in mouse brain. We also show that quality control of spatial omics data can be formulated as a domain identification problem and solved using BANKSY. Lastly, BANKSY is orders of magnitude faster and more scalable than existing spatial clustering methods, and thus capable of processing the large datasets generated by emerging spatial technologies. In summary, BANKSY represents an accurate, biologically motivated, scalable, and versatile framework for analyzing spatial omics data.
Visit talk page
https://simons.berkeley.edu/talks/tbd-443
From Algorithms to Discovery in Genome-Scale Biology and Medicine
Each cell type in a solid tissue has a characteristic transcriptome and spatial arrangement, both of which are observable using modern spatial omics assays. Surprisingly however, spatial information is frequently ignored when clustering cells to identify cell types and states. In fact, spatial location is typically considered only when solving the related, but distinct, problem of demarcating tissue domains (which could include multiple cell types). We present BANKSY, an algorithm that unifies cell type clustering and domain segmentation by constructing a product space of cell and neighborhood transcriptomes, representing cell state and microenvironment, respectively. BANKSY's spatial kernel-based feature augmentation strategy improves performance on both tasks when tested on diverse FISH- and sequencing-based spatial omics datasets. Uniquely, BANKSY identified hitherto undetected niche-dependent cell states in mouse brain. We also show that quality control of spatial omics data can be formulated as a domain identification problem and solved using BANKSY. Lastly, BANKSY is orders of magnitude faster and more scalable than existing spatial clustering methods, and thus capable of processing the large datasets generated by emerging spatial technologies. In summary, BANKSY represents an accurate, biologically motivated, scalable, and versatile framework for analyzing spatial omics data.
0:29:40
Martin Hemberg (Brigham and Women's Hospital)
https://simons.berkeley.edu/talks/spatial-transcriptomics-identifies-neighbourhoods-and-molecular-markers-alveolar-damage-lung
From Algorithms to Discovery in Genome-Scale Biology and Medicine
The integration of single cell and spatial transcriptomics provides a new approach to profile human disease pathology in situ. Here, I will introduce our work on dissecting lung alveolar damage in severe COVID-19 using a new single cell atlas and transcriptome wide spatial profiling of post-mortem lung tissue. First, we generated a comprehensive single-cell lung cell atlas through integration of multiple healthy and COVID-19 datasets. Second, we generated a spatially resolved transcriptomic dataset of diffuse alveolar damage (DAD) across different stages of pathology using the Nanostring WTA technology. To resolve changes in cell type abundance across progressive pathology, we integrated our single cell and spatial transcriptomic datasets. We identified dynamic sets of immune and stromal cells and tissue microenvironments that distinguish early (exudative) and late (organised) alveolar damage. Finally, we could re-map pathological phenotypes in our single-cell transcriptomic reference using pathology biomarkers identified from spatial data. Our work identifies candidate molecular and cellular targets of novel therapies for COVID-19 in the respiratory system.
Visit talk page
https://simons.berkeley.edu/talks/spatial-transcriptomics-identifies-neighbourhoods-and-molecular-markers-alveolar-damage-lung
From Algorithms to Discovery in Genome-Scale Biology and Medicine
The integration of single cell and spatial transcriptomics provides a new approach to profile human disease pathology in situ. Here, I will introduce our work on dissecting lung alveolar damage in severe COVID-19 using a new single cell atlas and transcriptome wide spatial profiling of post-mortem lung tissue. First, we generated a comprehensive single-cell lung cell atlas through integration of multiple healthy and COVID-19 datasets. Second, we generated a spatially resolved transcriptomic dataset of diffuse alveolar damage (DAD) across different stages of pathology using the Nanostring WTA technology. To resolve changes in cell type abundance across progressive pathology, we integrated our single cell and spatial transcriptomic datasets. We identified dynamic sets of immune and stromal cells and tissue microenvironments that distinguish early (exudative) and late (organised) alveolar damage. Finally, we could re-map pathological phenotypes in our single-cell transcriptomic reference using pathology biomarkers identified from spatial data. Our work identifies candidate molecular and cellular targets of novel therapies for COVID-19 in the respiratory system.
0:38:40
Zhiping Weng (UMass Chan Medical School)
https://simons.berkeley.edu/talks/landscapes-human-cis-regulatory-elements-and-transcription-factor-binding-sites-evolutionarily
From Algorithms to Discovery in Genome-Scale Biology and Medicine
Visit talk page
https://simons.berkeley.edu/talks/landscapes-human-cis-regulatory-elements-and-transcription-factor-binding-sites-evolutionarily
From Algorithms to Discovery in Genome-Scale Biology and Medicine
0:36:15
Patrick Aloy (Institute for Research in Biomedicine)
https://simons.berkeley.edu/talks/formatting-biological-big-data-enable-personalized-systems-pharmacology
From Algorithms to Discovery in Genome-Scale Biology and Medicine
Big Data analytical techniques and AI have the potential to transform drug discovery, as they are reshaping other areas of science and technology, but we need to blend biology and chemistry in a format that is amenable for modern machine learning. In this talk, I will present the Chemical Checker (CC), a resource that provides processed, harmonized and integrated bioactivity data on small molecules. The CC divides data into five levels of increasing complexity, ranging from the chemical properties of compounds to their clinical outcomes. In between, it considers targets, off-targets, perturbed biological networks and several cell-based assays such as gene expression, growth inhibition and morphological profiles. In the CC, bioactivity data are expressed in a vector format, which naturally extends the notion of chemical similarity between compounds to similarities between bioactivity signatures of different kinds. We show how CC signatures can boost the performance of drug discovery tasks that typically capitalize on chemical descriptors, including compound library optimization, target identification and anticipation of failures in clinical trials. Moreover, we demonstrate and experimentally validate that CC signatures can be used to reverse and mimic biological signatures of disease models and genetic perturbations, options that are otherwise impossible using chemical information alone. Indeed, using bioactivity signatures we have identified small molecules able to revert transcriptional signatures related to Alzheimer´s disease in vitro and in vivo, as well as compounds against Snail1, a transcription factor with an essential role in the epithelial-to-mesenchymal transition, showing that our approach might offer a new perspective to find small molecules able to modulate the activity of undruggable proteins.
Visit talk page
https://simons.berkeley.edu/talks/formatting-biological-big-data-enable-personalized-systems-pharmacology
From Algorithms to Discovery in Genome-Scale Biology and Medicine
Big Data analytical techniques and AI have the potential to transform drug discovery, as they are reshaping other areas of science and technology, but we need to blend biology and chemistry in a format that is amenable for modern machine learning. In this talk, I will present the Chemical Checker (CC), a resource that provides processed, harmonized and integrated bioactivity data on small molecules. The CC divides data into five levels of increasing complexity, ranging from the chemical properties of compounds to their clinical outcomes. In between, it considers targets, off-targets, perturbed biological networks and several cell-based assays such as gene expression, growth inhibition and morphological profiles. In the CC, bioactivity data are expressed in a vector format, which naturally extends the notion of chemical similarity between compounds to similarities between bioactivity signatures of different kinds. We show how CC signatures can boost the performance of drug discovery tasks that typically capitalize on chemical descriptors, including compound library optimization, target identification and anticipation of failures in clinical trials. Moreover, we demonstrate and experimentally validate that CC signatures can be used to reverse and mimic biological signatures of disease models and genetic perturbations, options that are otherwise impossible using chemical information alone. Indeed, using bioactivity signatures we have identified small molecules able to revert transcriptional signatures related to Alzheimer´s disease in vitro and in vivo, as well as compounds against Snail1, a transcription factor with an essential role in the epithelial-to-mesenchymal transition, showing that our approach might offer a new perspective to find small molecules able to modulate the activity of undruggable proteins.
0:34:57
Jimmie Ye (UCSF)
https://simons.berkeley.edu/talks/tbd-444
From Algorithms to Discovery in Genome-Scale Biology and Medicine
The integration of single cell and spatial transcriptomics provides a new approach to profile human disease pathology in situ. Here, I will introduce our work on dissecting lung alveolar damage in severe COVID-19 using a new single cell atlas and transcriptome wide spatial profiling of post-mortem lung tissue. First, we generated a comprehensive single-cell lung cell atlas through integration of multiple healthy and COVID-19 datasets. Second, we generated a spatially resolved transcriptomic dataset of diffuse alveolar damage (DAD) across different stages of pathology using the Nanostring WTA technology. To resolve changes in cell type abundance across progressive pathology, we integrated our single cell and spatial transcriptomic datasets. We identified dynamic sets of immune and stromal cells and tissue microenvironments that distinguish early (exudative) and late (organised) alveolar damage. Finally, we could re-map pathological phenotypes in our single-cell transcriptomic reference using pathology biomarkers identified from spatial data. Our work identifies candidate molecular and cellular targets of novel therapies for COVID-19 in the respiratory system.
Visit talk page
https://simons.berkeley.edu/talks/tbd-444
From Algorithms to Discovery in Genome-Scale Biology and Medicine
The integration of single cell and spatial transcriptomics provides a new approach to profile human disease pathology in situ. Here, I will introduce our work on dissecting lung alveolar damage in severe COVID-19 using a new single cell atlas and transcriptome wide spatial profiling of post-mortem lung tissue. First, we generated a comprehensive single-cell lung cell atlas through integration of multiple healthy and COVID-19 datasets. Second, we generated a spatially resolved transcriptomic dataset of diffuse alveolar damage (DAD) across different stages of pathology using the Nanostring WTA technology. To resolve changes in cell type abundance across progressive pathology, we integrated our single cell and spatial transcriptomic datasets. We identified dynamic sets of immune and stromal cells and tissue microenvironments that distinguish early (exudative) and late (organised) alveolar damage. Finally, we could re-map pathological phenotypes in our single-cell transcriptomic reference using pathology biomarkers identified from spatial data. Our work identifies candidate molecular and cellular targets of novel therapies for COVID-19 in the respiratory system.
0:29:1
Noam Shomron (TAU)
https://simons.berkeley.edu/talks/tbd-432
From Algorithms to Discovery in Genome-Scale Biology and Medicine
Metastasis is the primary cause for mortality in cancer. In our studies we show that breast cancer metastasis can be prevented by limiting cell movement using small RNA treatment. We compare experimental models that reveal the pathways involved in cancer aggressiveness. We pinpoint potential diagnostic markers that dictate the course of the disease. Overall, large data analysis and experimental studies assist in better understanding cancer genomics.
Visit talk page
https://simons.berkeley.edu/talks/tbd-432
From Algorithms to Discovery in Genome-Scale Biology and Medicine
Metastasis is the primary cause for mortality in cancer. In our studies we show that breast cancer metastasis can be prevented by limiting cell movement using small RNA treatment. We compare experimental models that reveal the pathways involved in cancer aggressiveness. We pinpoint potential diagnostic markers that dictate the course of the disease. Overall, large data analysis and experimental studies assist in better understanding cancer genomics.
0:32:30
Natasa Przulj (ICREA; Barcelona Supercomputing Center; University College London)
https://simons.berkeley.edu/talks/understanding-molecular-complexity-precision-medicine
From Algorithms to Discovery in Genome-Scale Biology and Medicine
We develop artificial intelligence (AI) methods for extracting new biomedical knowledge from the wiring patterns of systems-level, heterogeneous omics data. Our graphlet-based and other methods uncover the patterns in molecular (omics) networks and in the multi-scale organization of these networks indicative of biological function, translating the information hidden in the network topology into domain-specific knowledge. Also, we introduce a versatile data fusion (integration) machine learning (ML) framework to address key challenges in precision medicine from the wiring patterns of omics network data: better stratification of patients, prediction of driver genes in cancer, and re-purposing of approved drugs to particular patients and patient groups, including Covid-19 patients. Our new methods stem from novel network science algorithms coupled with graph-regularized non-negative matrix tri-factorization (NMTF), a machine learning technique for dimensionality reduction, inference and co-clustering of heterogeneous datasets. We utilize our new framework to develop methodologies for understanding the molecular organization of the omics data embedding space.
Visit talk page
https://simons.berkeley.edu/talks/understanding-molecular-complexity-precision-medicine
From Algorithms to Discovery in Genome-Scale Biology and Medicine
We develop artificial intelligence (AI) methods for extracting new biomedical knowledge from the wiring patterns of systems-level, heterogeneous omics data. Our graphlet-based and other methods uncover the patterns in molecular (omics) networks and in the multi-scale organization of these networks indicative of biological function, translating the information hidden in the network topology into domain-specific knowledge. Also, we introduce a versatile data fusion (integration) machine learning (ML) framework to address key challenges in precision medicine from the wiring patterns of omics network data: better stratification of patients, prediction of driver genes in cancer, and re-purposing of approved drugs to particular patients and patient groups, including Covid-19 patients. Our new methods stem from novel network science algorithms coupled with graph-regularized non-negative matrix tri-factorization (NMTF), a machine learning technique for dimensionality reduction, inference and co-clustering of heterogeneous datasets. We utilize our new framework to develop methodologies for understanding the molecular organization of the omics data embedding space.
0:34:51
Jian Ma (Carnegie Mellon University)
https://simons.berkeley.edu/talks/tbd-446
From Algorithms to Discovery in Genome-Scale Biology and Medicine
The chromosomes of the human genome are organized in three-dimensions by compartmentalizing the cell nucleus and different genomic loci also interact with each other. However, the principles underlying such 3D genome organization and its functional impact remain poorly understood. In this talk, I will introduce some of our recent work in developing representation learning methods to study single-cell 3D genome organization. Our methods reveal the single-cell chromatin interactome patterns in different cellular conditions and at different scales. We hope that these algorithms will provide new insights into the structure and function of nuclear organization in health and disease.
Visit talk page
https://simons.berkeley.edu/talks/tbd-446
From Algorithms to Discovery in Genome-Scale Biology and Medicine
The chromosomes of the human genome are organized in three-dimensions by compartmentalizing the cell nucleus and different genomic loci also interact with each other. However, the principles underlying such 3D genome organization and its functional impact remain poorly understood. In this talk, I will introduce some of our recent work in developing representation learning methods to study single-cell 3D genome organization. Our methods reveal the single-cell chromatin interactome patterns in different cellular conditions and at different scales. We hope that these algorithms will provide new insights into the structure and function of nuclear organization in health and disease.
0:26:51
Julia Salzman (Stanford)
https://simons.berkeley.edu/talks/new-approaches-direct-statistical-inference-genomics
From Algorithms to Discovery in Genome-Scale Biology and Medicine
I will discuss a unifying statistical formulation for many fundamental problems in genome science and develop a reference-free, highly efficient algorithm that solves it. This formulation allows us to construct an algorithm that performs inference on raw reads, avoiding references completely. We illustrate the power of our approach for new data-driven biological discovery with examples of novel single-cell resolved, cell-type-specific isoform expression, including splicing, expression in the major histocompatibility complex, and de novo prediction of viral protein adaptation including in SARS-CoV-2.
Visit talk page
https://simons.berkeley.edu/talks/new-approaches-direct-statistical-inference-genomics
From Algorithms to Discovery in Genome-Scale Biology and Medicine
I will discuss a unifying statistical formulation for many fundamental problems in genome science and develop a reference-free, highly efficient algorithm that solves it. This formulation allows us to construct an algorithm that performs inference on raw reads, avoiding references completely. We illustrate the power of our approach for new data-driven biological discovery with examples of novel single-cell resolved, cell-type-specific isoform expression, including splicing, expression in the major histocompatibility complex, and de novo prediction of viral protein adaptation including in SARS-CoV-2.
0:34:40
James Zou (Stanford University)
https://simons.berkeley.edu/talks/title-geometric-deep-learning-spatial-omics
From Algorithms to Discovery in Genome-Scale Biology and Medicine
I will discuss how to leverage large-scale EHR data linked with tumor mutation profiles to emulate cancer clinical trials in silico. This approach helps us to design real trials, making them more incluse and efficient (Liu et al. Nature 2021). It also helps to identify a large number of predictive biomarkers, i.e. mutations that impact the survival of patients who are taking specific chemo, targeted or immuno therapies (Liu et al. Nature Medicine 2022). These mutation-drug interactions are critical for treatment planning and precision oncology.
Visit talk page
https://simons.berkeley.edu/talks/title-geometric-deep-learning-spatial-omics
From Algorithms to Discovery in Genome-Scale Biology and Medicine
I will discuss how to leverage large-scale EHR data linked with tumor mutation profiles to emulate cancer clinical trials in silico. This approach helps us to design real trials, making them more incluse and efficient (Liu et al. Nature 2021). It also helps to identify a large number of predictive biomarkers, i.e. mutations that impact the survival of patients who are taking specific chemo, targeted or immuno therapies (Liu et al. Nature Medicine 2022). These mutation-drug interactions are critical for treatment planning and precision oncology.