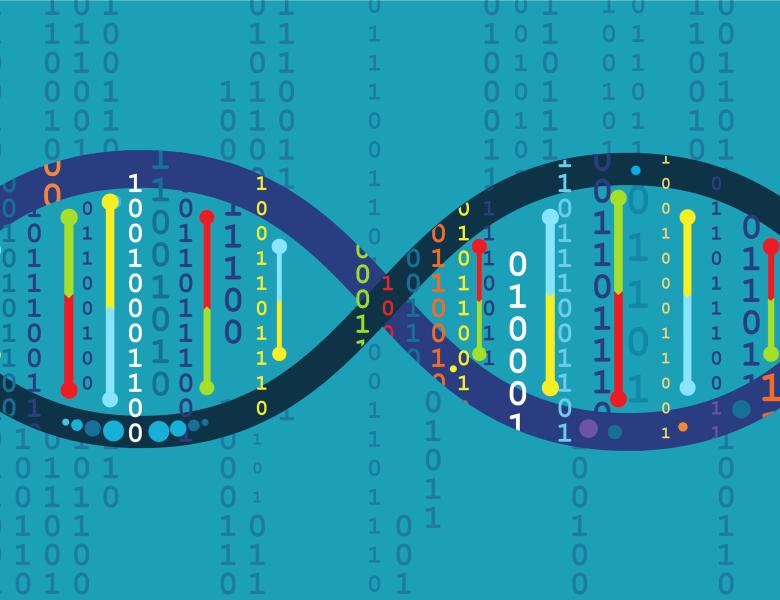
Abstract
We develop artificial intelligence (AI) methods for extracting new biomedical knowledge from the wiring patterns of systems-level, heterogeneous omics data. Our graphlet-based and other methods uncover the patterns in molecular (omics) networks and in the multi-scale organization of these networks indicative of biological function, translating the information hidden in the network topology into domain-specific knowledge. Also, we introduce a versatile data fusion (integration) machine learning (ML) framework to address key challenges in precision medicine from the wiring patterns of omics network data: better stratification of patients, prediction of driver genes in cancer, and re-purposing of approved drugs to particular patients and patient groups, including Covid-19 patients. Our new methods stem from novel network science algorithms coupled with graph-regularized non-negative matrix tri-factorization (NMTF), a machine learning technique for dimensionality reduction, inference and co-clustering of heterogeneous datasets. We utilize our new framework to develop methodologies for understanding the molecular organization of the omics data embedding space.