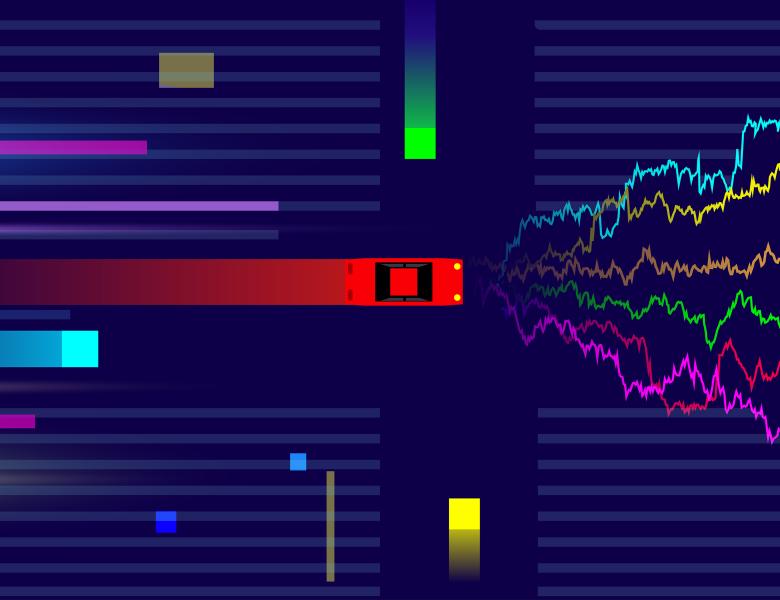
Playlist: 21 videos
Societal Considerations and Applications
0:58:26
Christina Ilvento (Google)
https://simons.berkeley.edu/talks/privacy-safe-measurement-web-open-questions-privacy-sandbox
Societal Considerations and Applications
The Privacy Sandbox aims "to create technologies that both protect people's privacy online and give companies and developers tools to build thriving digital businesses." This talk will describe some of the design, implementation and practical challenges in evolving measurement solutions away from persistent cross-site identifiers.
Visit talk page
https://simons.berkeley.edu/talks/privacy-safe-measurement-web-open-questions-privacy-sandbox
Societal Considerations and Applications
The Privacy Sandbox aims "to create technologies that both protect people's privacy online and give companies and developers tools to build thriving digital businesses." This talk will describe some of the design, implementation and practical challenges in evolving measurement solutions away from persistent cross-site identifiers.
1:7:31
Laura Brandimarte (University of Arizona)
https://simons.berkeley.edu/node/22918
Societal Considerations and Applications
In this talk I will review some of the psychological and economic factors influencing consumers’ desire and ability to manage their privacy effectively. Contrary to depictions of online sharing behaviors as careless, consumers fundamentally care about online privacy, but technological developments and economic forces have made it prohibitively difficult to attain desired, or even desirable, levels of privacy through individual action alone. The result does not have to be what some have called "digital resignation" though: a combination of individual and institutional efforts can change what seems to be the inevitability of the death of privacy into effective privacy protection.
Visit talk page
https://simons.berkeley.edu/node/22918
Societal Considerations and Applications
In this talk I will review some of the psychological and economic factors influencing consumers’ desire and ability to manage their privacy effectively. Contrary to depictions of online sharing behaviors as careless, consumers fundamentally care about online privacy, but technological developments and economic forces have made it prohibitively difficult to attain desired, or even desirable, levels of privacy through individual action alone. The result does not have to be what some have called "digital resignation" though: a combination of individual and institutional efforts can change what seems to be the inevitability of the death of privacy into effective privacy protection.
0:41:21
Wanrong Zhang (Harvard University)
https://simons.berkeley.edu/talks/concurrent-composition-theorems-all-standard-variants-differential-privacy
Societal Considerations and Applications
We study the concurrent composition properties of interactive differentially private mechanisms, whereby an adversary can arbitrarily interleave its queries to the different mechanisms. We prove that all composition theorems for non-interactive differentially private mechanisms extend to the concurrent composition of interactive differentially private mechanisms for all standard variants of differential privacy including $(\eps,\delta)$-DP with $\delta-0$, R\`enyi DP, and $f$-DP, thus answering the open question by \cite{vadhan2021concurrent}. For $f$-DP, which captures $(\eps,\delta)$-DP as a special case, we prove the concurrent composition theorems by showing that every interactive $f$-DP mechanism can be simulated by interactive post-processing of a non-interactive $f$-DP mechanism. For R\`enyi DP, we use a different approach by showing the optimal adversary against the concurrent composition can be decomposed as a product of the optimal adversaries against each interactive mechanism.
Visit talk page
https://simons.berkeley.edu/talks/concurrent-composition-theorems-all-standard-variants-differential-privacy
Societal Considerations and Applications
We study the concurrent composition properties of interactive differentially private mechanisms, whereby an adversary can arbitrarily interleave its queries to the different mechanisms. We prove that all composition theorems for non-interactive differentially private mechanisms extend to the concurrent composition of interactive differentially private mechanisms for all standard variants of differential privacy including $(\eps,\delta)$-DP with $\delta-0$, R\`enyi DP, and $f$-DP, thus answering the open question by \cite{vadhan2021concurrent}. For $f$-DP, which captures $(\eps,\delta)$-DP as a special case, we prove the concurrent composition theorems by showing that every interactive $f$-DP mechanism can be simulated by interactive post-processing of a non-interactive $f$-DP mechanism. For R\`enyi DP, we use a different approach by showing the optimal adversary against the concurrent composition can be decomposed as a product of the optimal adversaries against each interactive mechanism.
0:51:41
Vitaly Feldman (Apple ML Research)
https://simons.berkeley.edu/node/22921
Societal Considerations and Applications
Deep learning algorithms that achieve state-of-the-art results on image and text recognition tasks tend to fit the entire training dataset (nearly) perfectly including mislabeled examples and outliers. This propensity to memorize seemingly useless data and the resulting large generalization gap have puzzled many practitioners and is not explained by existing theories of machine learning. We provide a simple conceptual explanation and a theoretical model demonstrating that memorization of outliers and mislabeled examples is necessary for achieving close-to-optimal generalization error when learning from long-tailed data distributions. Image and text data are known to follow such distributions and therefore our results establish a formal link between these empirical phenomena. We then demonstrate the utility of memorization and support our explanation empirically. These results rely on a new technique for efficiently estimating memorization and influence of training data points. Our results allow us to quantify the cost of limiting memorization in learning and explain the disparate effects that privacy and model compression have on different subgroups.
Visit talk page
https://simons.berkeley.edu/node/22921
Societal Considerations and Applications
Deep learning algorithms that achieve state-of-the-art results on image and text recognition tasks tend to fit the entire training dataset (nearly) perfectly including mislabeled examples and outliers. This propensity to memorize seemingly useless data and the resulting large generalization gap have puzzled many practitioners and is not explained by existing theories of machine learning. We provide a simple conceptual explanation and a theoretical model demonstrating that memorization of outliers and mislabeled examples is necessary for achieving close-to-optimal generalization error when learning from long-tailed data distributions. Image and text data are known to follow such distributions and therefore our results establish a formal link between these empirical phenomena. We then demonstrate the utility of memorization and support our explanation empirically. These results rely on a new technique for efficiently estimating memorization and influence of training data points. Our results allow us to quantify the cost of limiting memorization in learning and explain the disparate effects that privacy and model compression have on different subgroups.
0:55:36
Aloni Cohen (Boston University)
https://simons.berkeley.edu/node/22924
Societal Considerations and Applications
Kerfuffle (/kərˈfəfəl/): a commotion or fuss, especially one caused by conflicting views. "There was a kerfuffle over the use of differential privacy for the 2020 Census." This talk will give a too-brief introduction to some of the issues that played out in tweets, court proceedings, and academic preprints. We'll also discuss approaches and challenges to understanding the effect of differential privacy on downstream policy.
Visit talk page
https://simons.berkeley.edu/node/22924
Societal Considerations and Applications
Kerfuffle (/kərˈfəfəl/): a commotion or fuss, especially one caused by conflicting views. "There was a kerfuffle over the use of differential privacy for the 2020 Census." This talk will give a too-brief introduction to some of the issues that played out in tweets, court proceedings, and academic preprints. We'll also discuss approaches and challenges to understanding the effect of differential privacy on downstream policy.
0:46:34
Alex Teytelboym (University of Oxford)
https://simons.berkeley.edu/talks/improving-refugee-resettlement
Societal Considerations and Applications
The current refugee resettlement system is inefficient because there are too few resettlement places and because refugees are resettled to locations where they might not thrive. I will overview some recent efforts to improve employment outcomes of refugees arriving to the United States. I will then describe some recent efforts to incorporate refugees' preferences in processes that match them to locations.
Visit talk page
https://simons.berkeley.edu/talks/improving-refugee-resettlement
Societal Considerations and Applications
The current refugee resettlement system is inefficient because there are too few resettlement places and because refugees are resettled to locations where they might not thrive. I will overview some recent efforts to improve employment outcomes of refugees arriving to the United States. I will then describe some recent efforts to incorporate refugees' preferences in processes that match them to locations.
0:41:25
Swati Gupta (Georgia Institute of Technology)
https://simons.berkeley.edu/talks/algorithmic-challenges-ensuring-fairness-time-decision
Societal Considerations and Applications
Algorithmic decision-making in societal contexts, such as retail pricing, loan administration, recommendations on online platforms, etc., often involves experimentation with decisions for the sake of learning, which results in perceptions of unfairness among people impacted by these decisions. It is hence necessary to embed appropriate notions of fairness in such decision-making processes. The goal of this paper is to highlight the rich interface between temporal notions of fairness and online decision-making through a novel meta-objective of ensuring fairness at the time of decision. Given some arbitrary comparative fairness notion for static decision-making (e.g., students should pay at most 90% of the general adult price), a corresponding online decision-making algorithm satisfies fairness at the time of decision if the said notion of fairness is satisfied for any entity receiving a decision in comparison to all the past decisions. We show that this basic requirement introduces new methodological challenges in online decision-making. We illustrate the novel approaches necessary to address these challenges in the context of stochastic convex optimization with bandit feedback under a comparative fairness constraint that imposes lower bounds on the decisions received by entities depending on the decisions received by everyone in the past. The talk will showcase some novel research opportunities in online decision-making stemming from temporal fairness concerns. This is based on joint work with Vijay Kamble and Jad Salem.
Visit talk page
https://simons.berkeley.edu/talks/algorithmic-challenges-ensuring-fairness-time-decision
Societal Considerations and Applications
Algorithmic decision-making in societal contexts, such as retail pricing, loan administration, recommendations on online platforms, etc., often involves experimentation with decisions for the sake of learning, which results in perceptions of unfairness among people impacted by these decisions. It is hence necessary to embed appropriate notions of fairness in such decision-making processes. The goal of this paper is to highlight the rich interface between temporal notions of fairness and online decision-making through a novel meta-objective of ensuring fairness at the time of decision. Given some arbitrary comparative fairness notion for static decision-making (e.g., students should pay at most 90% of the general adult price), a corresponding online decision-making algorithm satisfies fairness at the time of decision if the said notion of fairness is satisfied for any entity receiving a decision in comparison to all the past decisions. We show that this basic requirement introduces new methodological challenges in online decision-making. We illustrate the novel approaches necessary to address these challenges in the context of stochastic convex optimization with bandit feedback under a comparative fairness constraint that imposes lower bounds on the decisions received by entities depending on the decisions received by everyone in the past. The talk will showcase some novel research opportunities in online decision-making stemming from temporal fairness concerns. This is based on joint work with Vijay Kamble and Jad Salem.
0:41:16
Juba Ziani (Georgia Tech)
https://simons.berkeley.edu/talks/pipeline-interventions
Societal Considerations and Applications
We introduce the pipeline intervention problem, defined by a layered directed acyclic graph and a set of stochastic matrices governing transitions between successive layers. The graph is a stylized model for how people from different populations are presented opportunities, eventually leading to some reward. In our model, individuals are born into an initial position (i.e. some node in the first layer of the graph) according to a fixed probability distribution, and then stochastically progress through the graph according to the transition matrices, until they reach a node in the final layer of the graph; each node in the final layer has a reward associated with it. The pipeline intervention problem asks how to best make costly changes to the transition matrices governing people's stochastic transitions through the graph, subject to a budget constraint. We consider two objectives: social welfare maximization, and a fairness-motivated maximin objective that seeks to maximize the value to the population (starting node) with the least expected value. We consider two variants of the maximin objective that turn out to be distinct, depending on whether we demand a deterministic solution or allow randomization. For each objective, we give an efficient approximation algorithm (an additive FPTAS) for constant width networks. We also tightly characterize the "price of fairness" in our setting: the ratio between the highest achievable social welfare and the highest social welfare consistent with a maximin optimal solution. Finally we show that for polynomial width networks, even approximating the maximin objective to any constant factor is NP hard, even for networks with constant depth. This shows that the restriction on the width in our positive results is essential.
Visit talk page
https://simons.berkeley.edu/talks/pipeline-interventions
Societal Considerations and Applications
We introduce the pipeline intervention problem, defined by a layered directed acyclic graph and a set of stochastic matrices governing transitions between successive layers. The graph is a stylized model for how people from different populations are presented opportunities, eventually leading to some reward. In our model, individuals are born into an initial position (i.e. some node in the first layer of the graph) according to a fixed probability distribution, and then stochastically progress through the graph according to the transition matrices, until they reach a node in the final layer of the graph; each node in the final layer has a reward associated with it. The pipeline intervention problem asks how to best make costly changes to the transition matrices governing people's stochastic transitions through the graph, subject to a budget constraint. We consider two objectives: social welfare maximization, and a fairness-motivated maximin objective that seeks to maximize the value to the population (starting node) with the least expected value. We consider two variants of the maximin objective that turn out to be distinct, depending on whether we demand a deterministic solution or allow randomization. For each objective, we give an efficient approximation algorithm (an additive FPTAS) for constant width networks. We also tightly characterize the "price of fairness" in our setting: the ratio between the highest achievable social welfare and the highest social welfare consistent with a maximin optimal solution. Finally we show that for polynomial width networks, even approximating the maximin objective to any constant factor is NP hard, even for networks with constant depth. This shows that the restriction on the width in our positive results is essential.
0:47:25
Hima Lakkaraju (Harvard University)
https://simons.berkeley.edu/node/22930
Societal Considerations and Applications
As various post hoc explanation methods are increasingly being leveraged to explain complex models in high-stakes settings, it becomes critical to develop a deeper understanding of if and when the explanations output by these methods disagree with each other, why these disagreements occur, and how to address these disagreements in a rigorous fashion. However, there is little to no research that provides answers to these critical questions. In this talk, I will present some of our recent research which addresses the aforementioned questions. More specifically, I will discuss i) a novel quantitative framework to formalize the disagreement between state-of-the-art feature attribution based explanation methods (e.g., LIME, SHAP, Gradient based methods). I will also touch upon on how this framework was constructed by leveraging inputs from interviews and user studies with data scientists who utilize explanation methods in their day-to-day work; ii) an online user study to understand how data scientists resolve disagreements in explanations output by the aforementioned methods; iii) a novel function approximation framework to explain why explanation methods often disagree with each other. I will demonstrate that all the key feature attribution based explanation methods are essentially performing local function approximations albeit, with different loss functions and notions of neighborhood. (iv) a set of guiding principles on how to choose explanation methods and resulting explanations when they disagree in real-world settings. I will conclude this talk by presenting a brief overview of an open source framework that we recently developed called Open-XAI which enables researchers and practitioners to seamlessly evaluate and benchmark both existing and new explanation methods based on various characteristics such as faithfulness, stability, and fairness.
Visit talk page
https://simons.berkeley.edu/node/22930
Societal Considerations and Applications
As various post hoc explanation methods are increasingly being leveraged to explain complex models in high-stakes settings, it becomes critical to develop a deeper understanding of if and when the explanations output by these methods disagree with each other, why these disagreements occur, and how to address these disagreements in a rigorous fashion. However, there is little to no research that provides answers to these critical questions. In this talk, I will present some of our recent research which addresses the aforementioned questions. More specifically, I will discuss i) a novel quantitative framework to formalize the disagreement between state-of-the-art feature attribution based explanation methods (e.g., LIME, SHAP, Gradient based methods). I will also touch upon on how this framework was constructed by leveraging inputs from interviews and user studies with data scientists who utilize explanation methods in their day-to-day work; ii) an online user study to understand how data scientists resolve disagreements in explanations output by the aforementioned methods; iii) a novel function approximation framework to explain why explanation methods often disagree with each other. I will demonstrate that all the key feature attribution based explanation methods are essentially performing local function approximations albeit, with different loss functions and notions of neighborhood. (iv) a set of guiding principles on how to choose explanation methods and resulting explanations when they disagree in real-world settings. I will conclude this talk by presenting a brief overview of an open source framework that we recently developed called Open-XAI which enables researchers and practitioners to seamlessly evaluate and benchmark both existing and new explanation methods based on various characteristics such as faithfulness, stability, and fairness.
1:4:24
Noa Dagan (Clalit Health Services)
https://simons.berkeley.edu/talks/predictive-modeling-healthcare-ai-special-considerations
Societal Considerations and Applications
Prediction models in healthcare are being utilized for many tasks. However, the use of these models for medical decision-making warrants special considerations that are less critical when prediction models are used in other domains. Two of these considerations, which we will discuss in the talk, are fairness and explainability. We will discuss these considerations from the viewpoint of a large healthcare organization that uses prediction models ubiquity on a daily basis. We will also describe how academic collaborations can expand our toolbox for handling these issues in practice.
Visit talk page
https://simons.berkeley.edu/talks/predictive-modeling-healthcare-ai-special-considerations
Societal Considerations and Applications
Prediction models in healthcare are being utilized for many tasks. However, the use of these models for medical decision-making warrants special considerations that are less critical when prediction models are used in other domains. Two of these considerations, which we will discuss in the talk, are fairness and explainability. We will discuss these considerations from the viewpoint of a large healthcare organization that uses prediction models ubiquity on a daily basis. We will also describe how academic collaborations can expand our toolbox for handling these issues in practice.