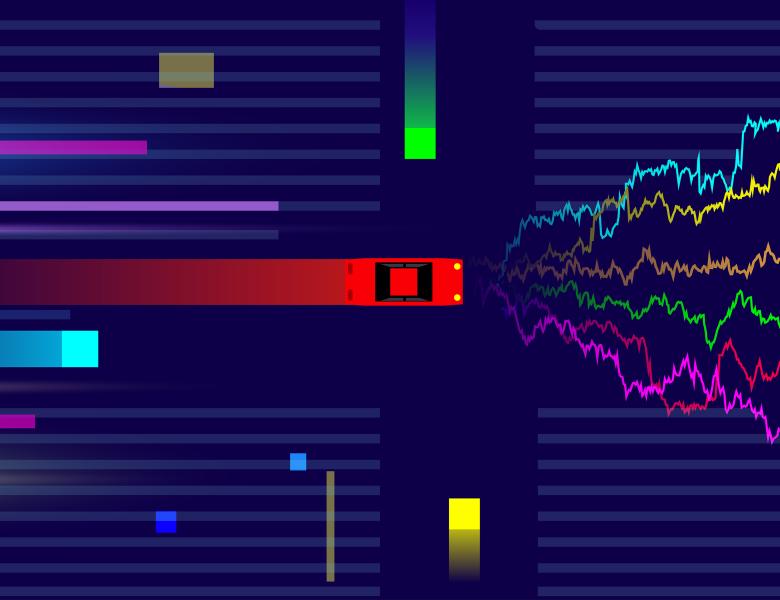
Abstract
Algorithmic decision-making in societal contexts, such as retail pricing, loan administration, recommendations on online platforms, etc., often involves experimentation with decisions for the sake of learning, which results in perceptions of unfairness among people impacted by these decisions. It is hence necessary to embed appropriate notions of fairness in such decision-making processes. The goal of this paper is to highlight the rich interface between temporal notions of fairness and online decision-making through a novel meta-objective of ensuring fairness at the time of decision. Given some arbitrary comparative fairness notion for static decision-making (e.g., students should pay at most 90% of the general adult price), a corresponding online decision-making algorithm satisfies fairness at the time of decision if the said notion of fairness is satisfied for any entity receiving a decision in comparison to all the past decisions. We show that this basic requirement introduces new methodological challenges in online decision-making. We illustrate the novel approaches necessary to address these challenges in the context of stochastic convex optimization with bandit feedback under a comparative fairness constraint that imposes lower bounds on the decisions received by entities depending on the decisions received by everyone in the past. The talk will showcase some novel research opportunities in online decision-making stemming from temporal fairness concerns. This is based on joint work with Vijay Kamble and Jad Salem.