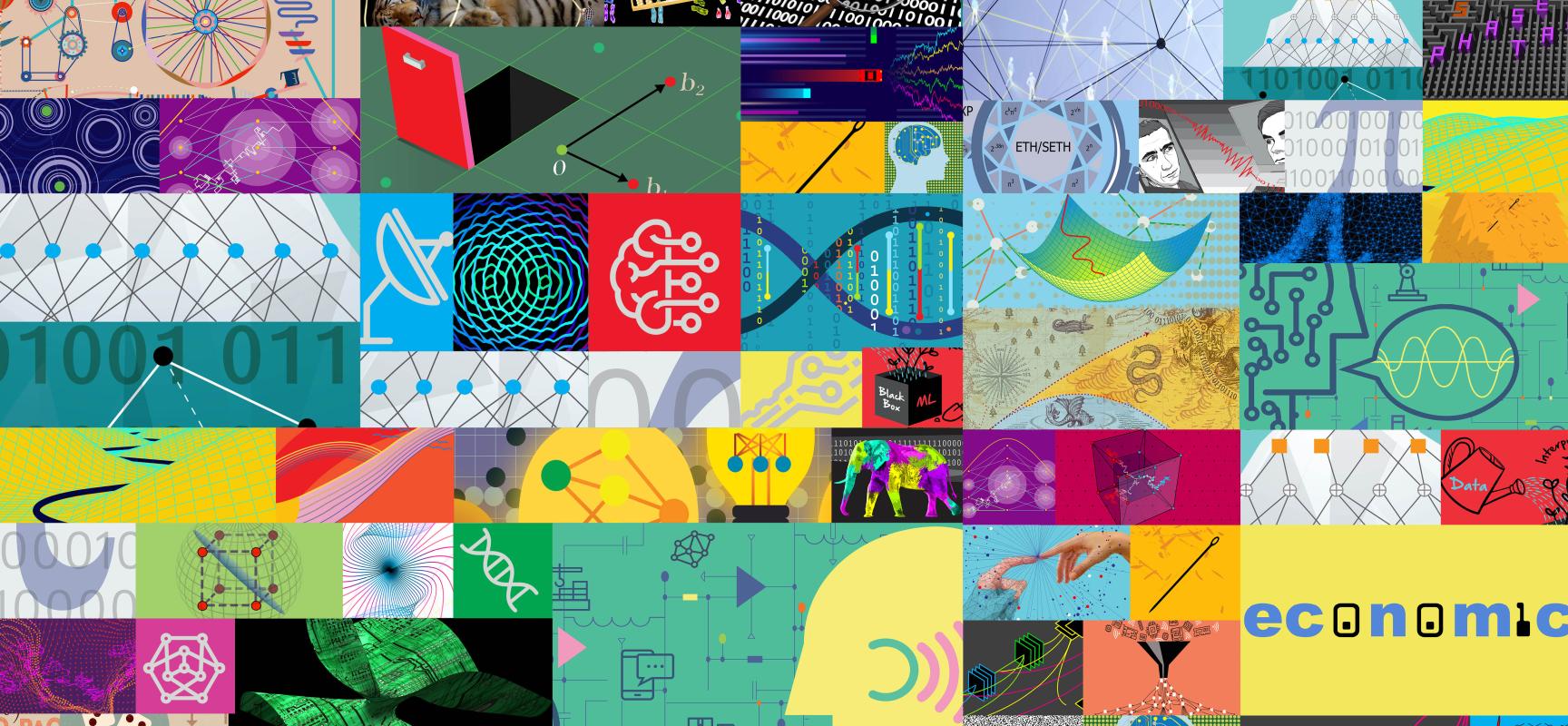
Internal Program Activities
No Internal Activities yet.
Charles Anthony Carlson (University of Colorado, Boulder)
Nisheeth Vishnoi (Yale University)
No Internal Activities yet.