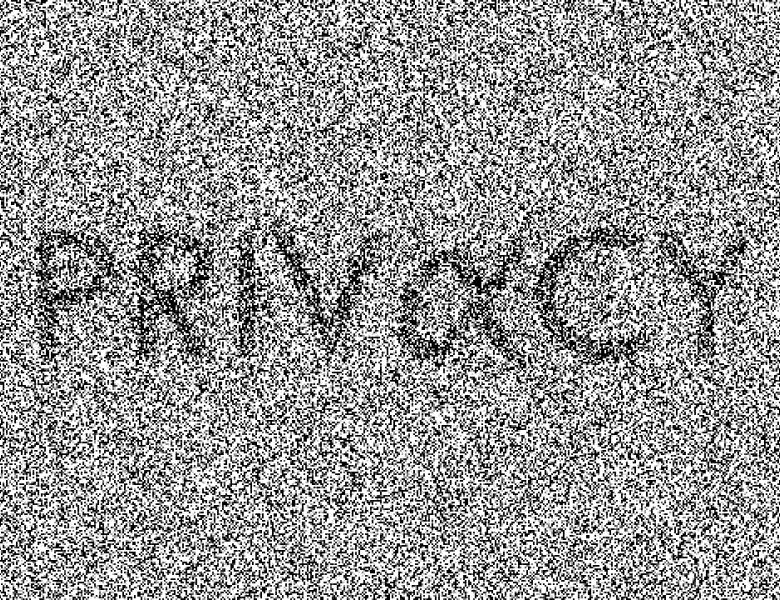
Differential privacy is at a turning point. Implementations in private industry, the public sector, and academia present the opportunity to measure and compare the total privacy loss incurred by data-intensive activities. Appropriately leveraged, this could be a watershed moment for privacy. However, like other technologies and techniques that allow for a range of instantiations, the details of differentially private implementations matter for such assessments. When meaningfully implemented, differential privacy supports deep data-driven insights with minimal worst-case privacy loss. When not meaningfully implemented, differential privacy delivers privacy mostly in name. Using differential privacy to maximize learning while providing a meaningful degree of privacy requires judicious choices with respect to the privacy parameter epsilon and careful selection of other factors. Today there is little understanding of what is the optimal value of epsilon for a given system or class of systems, purposes, data etc., or how to go about figuring it out. To understand current differential privacy implementations and how organizations currently make these key choices in practice, we conducted interviews with differential privacy practitioners to learn from their experiences. We found no clear consensus on how to choose epsilon, nor agreement on how to approach this and other key implementation decisions. Given the importance of these details there is a need for shared learning among the differential privacy community. To serve these purposes, and foster competition, we propose the creation of the Epsilon Registry, a publicly available communal body of knowledge about differential privacy implementations that can be used by various stakeholders to drive the identification and adoption of judicious differentially private implementations.
Joint work with Cynthia Dwork and Nitin Kohli
All scheduled dates:
Upcoming
No Upcoming activities yet