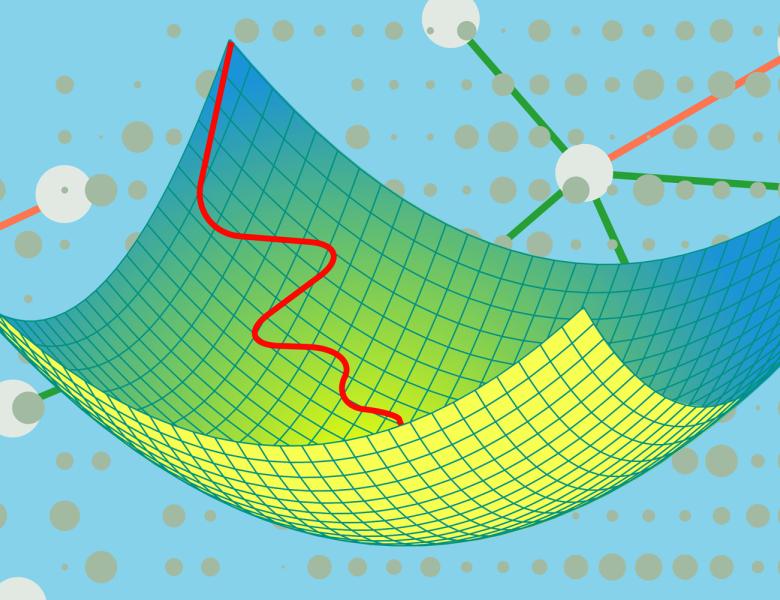
Recent advances in optimization, such as the development of fast iterative methods based on gradient descent, have enabled many breakthroughs in algorithm design. This workshop focuses on these recent advances in optimization and their implications for algorithm design. The workshop will explore both advances and open problems in the specific area of optimization as well as improvements in other areas of algorithm design that have leveraged optimization results as a key routine.
Specific topics to cover include gradient descent methods for convex and non-convex optimization problems; algorithms for solving structured linear systems; algorithms for graph problems such as maximum flows and cuts, connectivity, and graph sparsification; submodular optimization.
Deeksha Adil (ETH Zurich), Brian Bullins (Purdue University), Xiang Cheng (Massachusetts Institute of Technology), Jelena Diakonikolas (University of Wisconsin-Madison), Sally Dong (University of Washington), Petros Drineas (Purdue University), Maryam Fazel (University of Washington), Haotian Jiang (Microsoft Research, Redmond), Shunhua Jiang (Columbia University), Guanghui Lan (Georgia Institute of Technology), Yang Liu (Stanford University), Tengyu Ma (Stanford University), Cameron Musco (University of Massachusetts Amherst), Bento Natura (Georgia Institute of Technology), Debmalya Panigrahi (Duke University), Courtney Paquette (McGill University), Kent Quanrud (Purdue University), Mohit Singh (Georgia Institute of Technology), Suvrit Sra (TU Munich / MIT), Kevin Tian (UT Austin), Santosh Vempala (Georgia Tech), Adrian Vladu ((None)), László Végh (London School of Economics), Melanie Weber (Harvard University), Omri Weinstein (Hebrew University & Columbia University), David Woodruff (Carnegie Mellon University), Sorrachai Yingchareonthawornchai (Simons Institute, UC Berkeley)