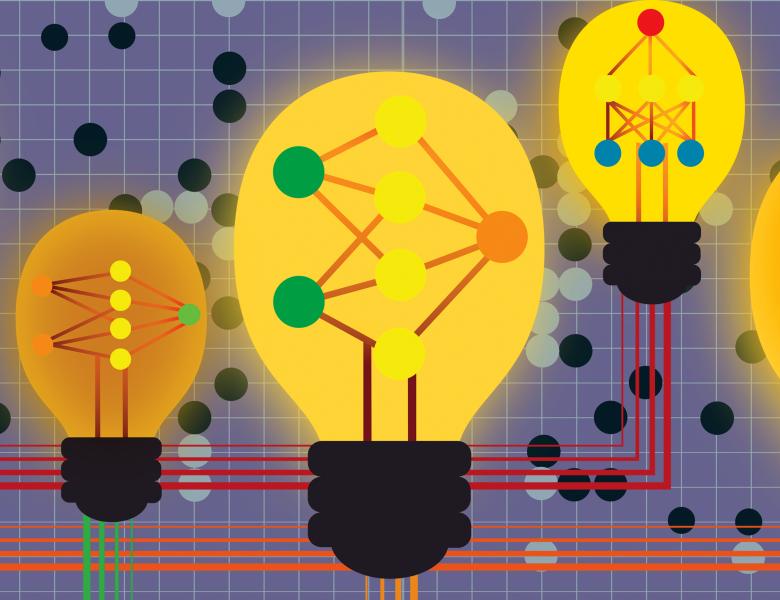
Many of the most exciting recent applications of reinforcement learning are game theoretic in nature. Agents must learn in the presence of other agents whose decisions influence the feedback they gather, and must explore and optimize their own decisions in anticipation of how they will affect the other agents and the state of the world. Such problems are naturally modeled through the framework of multi-agent reinforcement learning (MARL) — i.e., as problems of learning and optimization in multi-agent stochastic games.
While the basic (single-agent) reinforcement learning problem has been the subject of intense recent investigation — including development of efficient algorithms with provable, non-asymptotic theoretical guarantees — multi-agent reinforcement learning has been comparatively unexplored. This workshop will focus on developing strong theoretical foundations for multi-agent reinforcement learning, and on bridging gaps between theory and practice.
Tamer Başar (University of Illinois Urbana-Champaign), Kalesha Bullard (DeepMind), Simon Du (University of Washington), Abhimanyu Dubey (FAIR), Gabriele Farina (CMU), Drew Fudenberg (MIT), Noah Golowich (MIT & Google), Amy Greenwald (Brown University), Sergiu Hart (Hebrew University of Jerusalem), Elad Hazan (Princeton University), Katja Hofmann (Microsoft Research), Chi Jin (Princeton University), Sham Kakade (Harvard and MSR), Sham Kakade (University of Washington), Marc Lanctot (DeepMind), Marc Lanctot (DeepMind), Na Li (Harvard University), Haipeng Luo (University of Southern California), Eric Mazumdar (California Institute of Technology), Vidya Muthukumar (Georgia Institute of Technology), Ann Nowe (Vrije Universiteit Brussel (VUB)), Ioannis Panageas (UC Irvine), Alex Peysakhovich (Facebook), Georgios Piliouras (Singapore University of Technology and Design), Doina Precup (McGill University), Dorsa Sadigh (Stanford University), Mark Sellke (Stanford), Sylvain Sorin (Sorbonne Universite), Zhuoran Yang (Princeton University), Kaiqing Zhang (MIT)