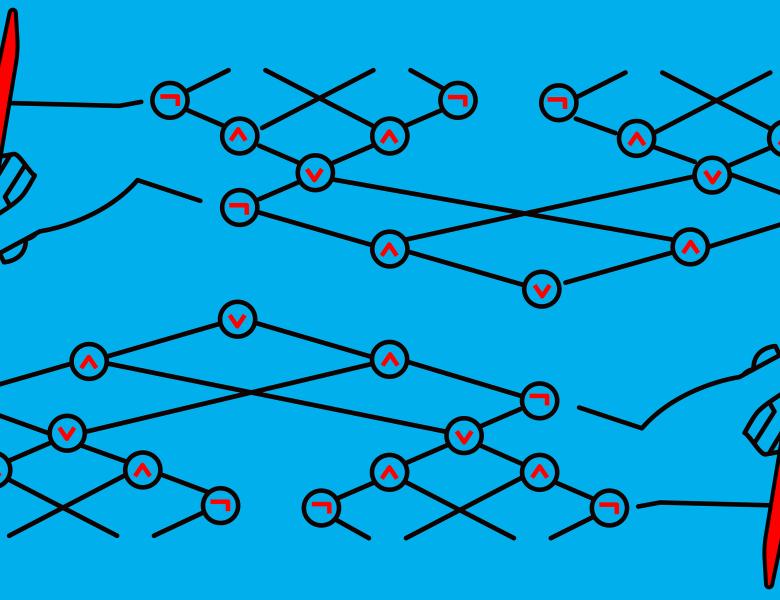
About
This workshop will build on recent insights about connections among complexity lower bounds, learning, and average-case complexity [Carmosino-Impagliazzo-Kabanets-Kolokolova16, Hirahara18]. Some of the major questions addressed will be: Are meta-complexity problems such as MCSP and Kpoly NP-hard, and what obstacles are there to showing hardness? Are there worst-case to average-case reductions for complexity classes such as NP and P? What are the weakest average-case hardness assumptions under which we can rule out PAC learning of DNFs? Are restricted circuit classes such as constant-depth circuits with mod m gates (for composite m) and depth-two threshold circuits learnable efficiently under the uniform distribution?
Chairs/Organizers