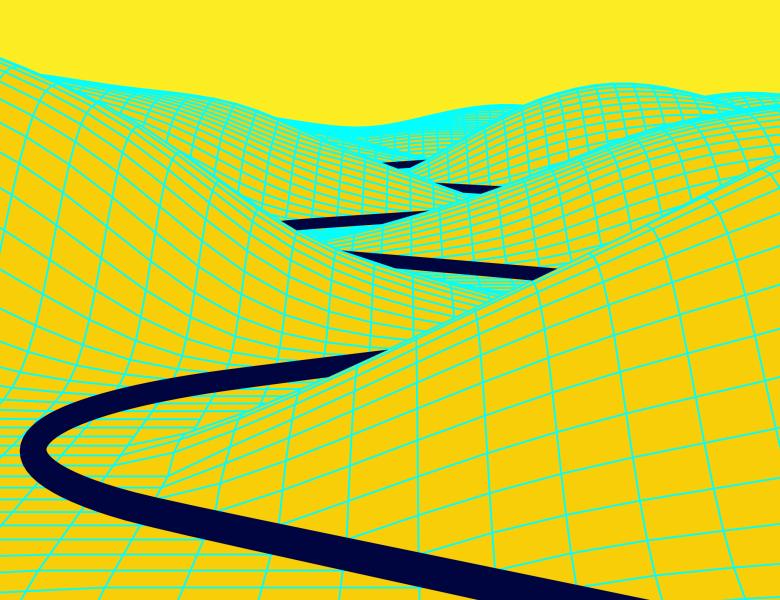
Playlist: 17 videos
Deep Learning Theory Workshop and Summer School
1:0:41
Chelsea Finn (Stanford)
https://simons.berkeley.edu/node/21935
Deep Learning Theory Workshop and Summer School
Visit talk page
https://simons.berkeley.edu/node/21935
Deep Learning Theory Workshop and Summer School
0:57:15
Pragya Sur (Harvard)
https://simons.berkeley.edu/node/21934
Deep Learning Theory Workshop and Summer School
Visit talk page
https://simons.berkeley.edu/node/21934
Deep Learning Theory Workshop and Summer School
1:7:31
Ahmed El Alaoui (Cornell)
https://simons.berkeley.edu/talks/methods-statistical-physics-iii
Deep Learning Theory Workshop and Summer School
Visit talk page
https://simons.berkeley.edu/talks/methods-statistical-physics-iii
Deep Learning Theory Workshop and Summer School
1:0:6
Song Mei (UC Berkeley)
https://simons.berkeley.edu/node/21939
Deep Learning Theory Workshop and Summer School
Recent empirical work has shown that hierarchical convolutional kernels inspired by convolutional neural networks (CNNs) significantly improve the performance of kernel methods in image classification tasks. A widely accepted explanation for the success of these architectures is that they encode hypothesis classes that are suitable for natural images. However, understanding the precise interplay between approximation and generalization in convolutional architectures remains a challenge.
In this talk, we consider the stylized setting of covariates (image pixels), and fully characterize the RKHS of kernels composed of single layers of convolution, pooling, and downsampling operations. We then study the gain in sample efficiency of kernel methods using these kernels over standard inner-product kernels. In particular, we show that 1) the convolution layer breaks the curse of dimensionality by restricting the RKHS to `local' functions; 2) global average pooling enforces the learned function to be translation invariant; 3) local pooling biases learning towards low-frequency functions. Notably, our results quantify how choosing an architecture adapted to the target function leads to a large improvement in the sample complexity.
Visit talk page
https://simons.berkeley.edu/node/21939
Deep Learning Theory Workshop and Summer School
Recent empirical work has shown that hierarchical convolutional kernels inspired by convolutional neural networks (CNNs) significantly improve the performance of kernel methods in image classification tasks. A widely accepted explanation for the success of these architectures is that they encode hypothesis classes that are suitable for natural images. However, understanding the precise interplay between approximation and generalization in convolutional architectures remains a challenge.
In this talk, we consider the stylized setting of covariates (image pixels), and fully characterize the RKHS of kernels composed of single layers of convolution, pooling, and downsampling operations. We then study the gain in sample efficiency of kernel methods using these kernels over standard inner-product kernels. In particular, we show that 1) the convolution layer breaks the curse of dimensionality by restricting the RKHS to `local' functions; 2) global average pooling enforces the learned function to be translation invariant; 3) local pooling biases learning towards low-frequency functions. Notably, our results quantify how choosing an architecture adapted to the target function leads to a large improvement in the sample complexity.
0:57:45
Subhabrata Sen (Harvard)
https://simons.berkeley.edu/node/21940
Deep Learning Theory Workshop and Summer School
Approximate Message Passing (AMP) is a class of efficient iterative algorithms that have been extensively utilized for signal recovery in high-dimensional inference problems. At each iteration, the algorithm involves a vector-matrix product, followed by an application of a non-linear map coordinate-wise to the vector obtained. The main attraction of AMP arises from the fact that the limiting empirical distributions of AMP iterates are gaussian, with means and variances that can be characterized in terms of a low-dimensional recursion known as \emph{state evolution}. These guarantees are usually derived under very specific distributional assumptions on the matrix e.g. iid gaussian entries, or orthogonally invariant matrices. However, numerical investigations indicate that AMP algorithms have a remarkable degree of universality to the data distribution. We will discuss universality of AMP algorithms on a class of \emph{semi-random} matrices, which can be significantly less random than matrices with iid entries. Time permitting, I will discuss the implications for statistical learning problems.
This is based on joint work with Rishabh Dudeja and Yue Lu (Harvard).
Visit talk page
https://simons.berkeley.edu/node/21940
Deep Learning Theory Workshop and Summer School
Approximate Message Passing (AMP) is a class of efficient iterative algorithms that have been extensively utilized for signal recovery in high-dimensional inference problems. At each iteration, the algorithm involves a vector-matrix product, followed by an application of a non-linear map coordinate-wise to the vector obtained. The main attraction of AMP arises from the fact that the limiting empirical distributions of AMP iterates are gaussian, with means and variances that can be characterized in terms of a low-dimensional recursion known as \emph{state evolution}. These guarantees are usually derived under very specific distributional assumptions on the matrix e.g. iid gaussian entries, or orthogonally invariant matrices. However, numerical investigations indicate that AMP algorithms have a remarkable degree of universality to the data distribution. We will discuss universality of AMP algorithms on a class of \emph{semi-random} matrices, which can be significantly less random than matrices with iid entries. Time permitting, I will discuss the implications for statistical learning problems.
This is based on joint work with Rishabh Dudeja and Yue Lu (Harvard).
1:12:25
Ethan Dyer (Google Research, Blueshift Team)
https://simons.berkeley.edu/talks/tutorial-emergent-behaviors-deep-learning
Deep Learning Theory Workshop and Summer School
Abstract: Deep learning continues its march of performance progress as models and datasets are scaled up. This talk will discuss work investigating performance predictability with model, dataset, and compute scale for deep learning in general and large language models in particular. I will review scaling in linear models -- a simple analytic system exhibiting many of the phenomena characteristic of realistic networks. I will also discuss empirical work attempting to investigate what types of problems can practically be solved by scale alone and what types cannot.
Visit talk page
https://simons.berkeley.edu/talks/tutorial-emergent-behaviors-deep-learning
Deep Learning Theory Workshop and Summer School
Abstract: Deep learning continues its march of performance progress as models and datasets are scaled up. This talk will discuss work investigating performance predictability with model, dataset, and compute scale for deep learning in general and large language models in particular. I will review scaling in linear models -- a simple analytic system exhibiting many of the phenomena characteristic of realistic networks. I will also discuss empirical work attempting to investigate what types of problems can practically be solved by scale alone and what types cannot.
1:13:16
Chloe Hsu (UC Berkeley)
https://simons.berkeley.edu/talks/tutorial-deep-learning-applications-structural-biology-and-protein-engineering
Deep Learning Theory Workshop and Summer School
Tutorial: Deep Learning Applications in Structural Biology and Protein Engineering
Abstract: There are about 20,000 different proteins in each one of us, humans. These proteins carry out a diverse set of functions to keep us all alive and healthy. Recently, deep learning has been increasingly used to both 1) help us visualize and gain insights into naturally existing proteins and 2) design novel proteins for therapeutic and environmental applications. In this talk, we will take a deep dive into the inner workings of AlphaFold2 and other emerging deep learning methods in structural biology and protein design. We will also examine the assumptions on biological data distributions and discuss hypotheses for the crucial ingredients of successful deep learning applications.
Visit talk page
https://simons.berkeley.edu/talks/tutorial-deep-learning-applications-structural-biology-and-protein-engineering
Deep Learning Theory Workshop and Summer School
Tutorial: Deep Learning Applications in Structural Biology and Protein Engineering
Abstract: There are about 20,000 different proteins in each one of us, humans. These proteins carry out a diverse set of functions to keep us all alive and healthy. Recently, deep learning has been increasingly used to both 1) help us visualize and gain insights into naturally existing proteins and 2) design novel proteins for therapeutic and environmental applications. In this talk, we will take a deep dive into the inner workings of AlphaFold2 and other emerging deep learning methods in structural biology and protein design. We will also examine the assumptions on biological data distributions and discuss hypotheses for the crucial ingredients of successful deep learning applications.