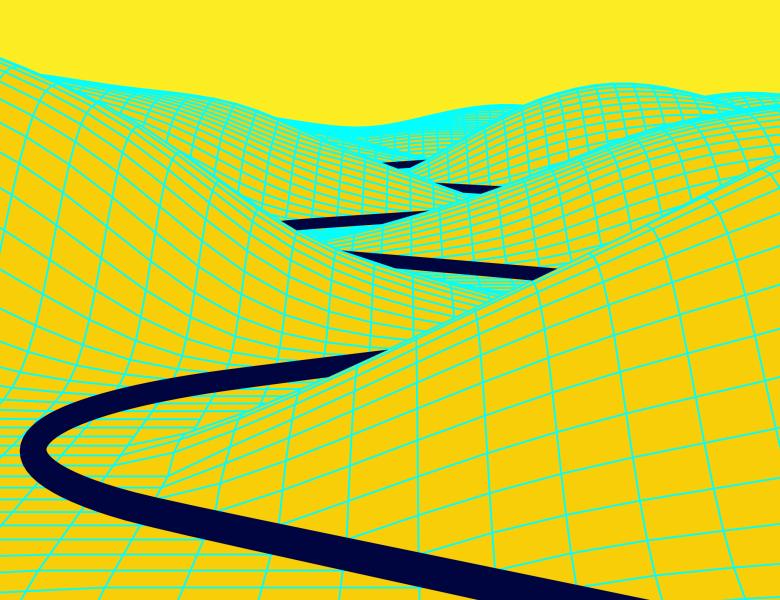
Abstract
Abstract: Approximate Message Passing (AMP) is a class of efficient iterative algorithms that have been extensively utilized for signal recovery in high-dimensional inference problems. At each iteration, the algorithm involves a vector-matrix product, followed by an application of a non-linear map coordinate-wise to the vector obtained. The main attraction of AMP arises from the fact that the limiting empirical distributions of AMP iterates are gaussian, with means and variances that can be characterized in terms of a low-dimensional recursion known as \emph{state evolution}. These guarantees are usually derived under very specific distributional assumptions on the matrix e.g. iid gaussian entries, or orthogonally invariant matrices. However, numerical investigations indicate that AMP algorithms have a remarkable degree of universality to the data distribution. We will discuss universality of AMP algorithms on a class of \emph{semi-random} matrices, which can be significantly less random than matrices with iid entries. Time permitting, I will discuss the implications for statistical learning problems.
This is based on joint work with Rishabh Dudeja and Yue Lu (Harvard).