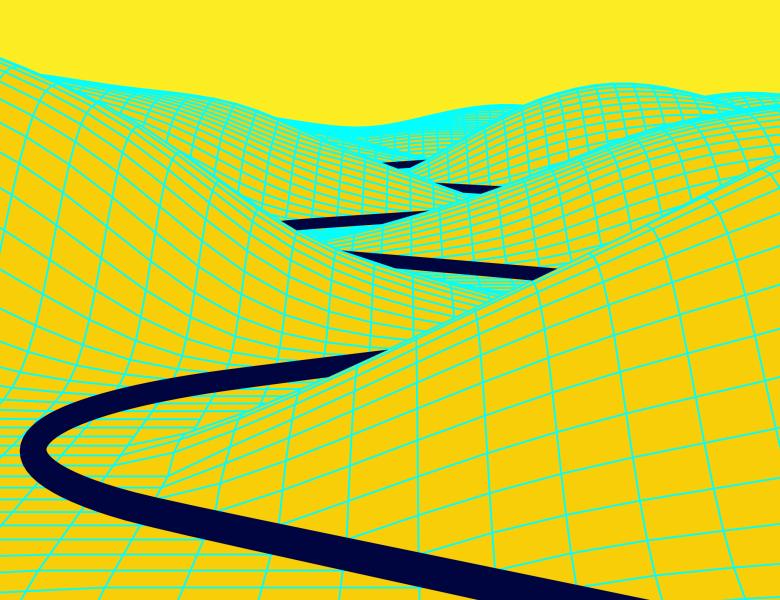
Abstract
Abstract: Causal inference from high-dimensional observational studies poses intriguing challenges. In this context, the augmented inverse probability weighting estimator is widely used for average treatment effect estimation. This estimator exhibits fascinating properties, such as double robustness. However, existing statistical guarantees rely on some form of sparsity in the underlying model, and may fail to apply in practical settings when these assumptions are violated.
In this talk, we present a new central limit theorem for this estimator, that applies in high dimensions, without sparsity-type assumptions on underlying signals. Specifically, we work in the proportional asymptotics regime, where the number of features and samples are both large and comparable. Our work uncovers novel high-dimensional phenomena that are strikingly different from their classical counterparts.
To conclude, we discuss opportunities that arise in our framework, when modern machine-learning-based estimators are used for learning the high-dimensional nuisance parameters. On the technical front, our work utilizes a novel interplay between three distinct tools---the theory of deterministic equivalents, approximate message passing theory, and the leave-one-out approach (alternately known as the cavity method in statistical physics).
This is based on joint work with Kuanhao Jiang, Rajarshi Mukherjee, and Subhabrata Sen (Harvard).