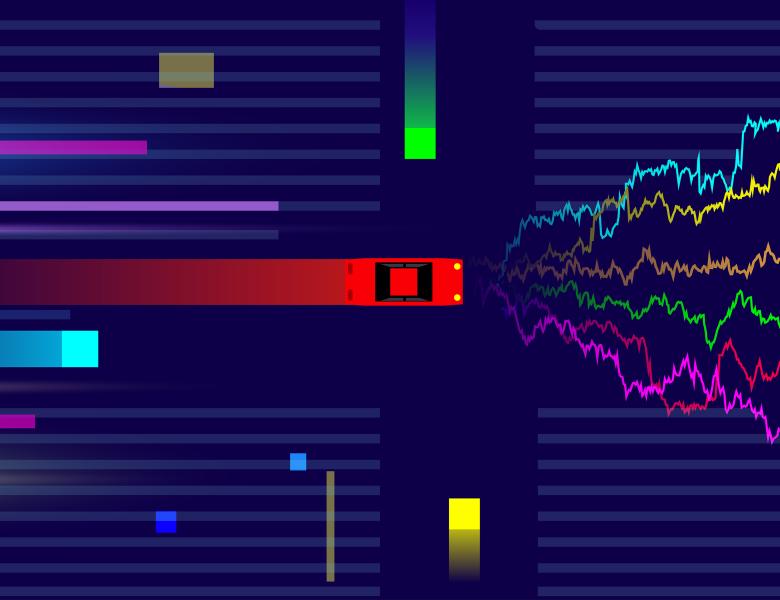
The workshop will explore online decision-making under different modeling assumptions on the reward structure. The two classical approaches for that consist of the setting where rewards are stochastic from a distribution and the one where they are adversarially selected. We will discuss different hybrid models to go between these extremes (data-dependent algorithms that adapt to “easy data”, model-predictive methods, ML-augmented algorithms, etc). We will also consider settings where the rewards come from agents with particular behavioral or choice models and how the algorithms need to change to adapt to that.
Anish Agarwal (MIT), Yossi Azar (Tel-Aviv University), Arjada Bardhi (Duke University), Steve Callander (Stanford University), Modibo Camara (Northwestern University), Victor Gabillon (Queensland University of Technology), Kyra (Jingyi) Gan (Harvard University), Ravi Kumar (Google), Hannah Li (Stanford), Ilan Lobel ((None)), Benjamin Moseley (Carnegie Mellon University), Vidya Muthukumar (Georgia Institute of Technology), Marco Ottaviani (Bocconi University), Chara Podimata (UC Berkeley), Aleksandrs Slivkins (Microsoft Research), Wen Sun (Cornell University), Csaba Szepesvári (University of Alberta, Google DeepMind), Panos Toulis (University of Chicago, Booth School of Business), Can Urgun (Princeton University), Haifeng Xu (University of Chicago), Julian Zimmert (Google Research)