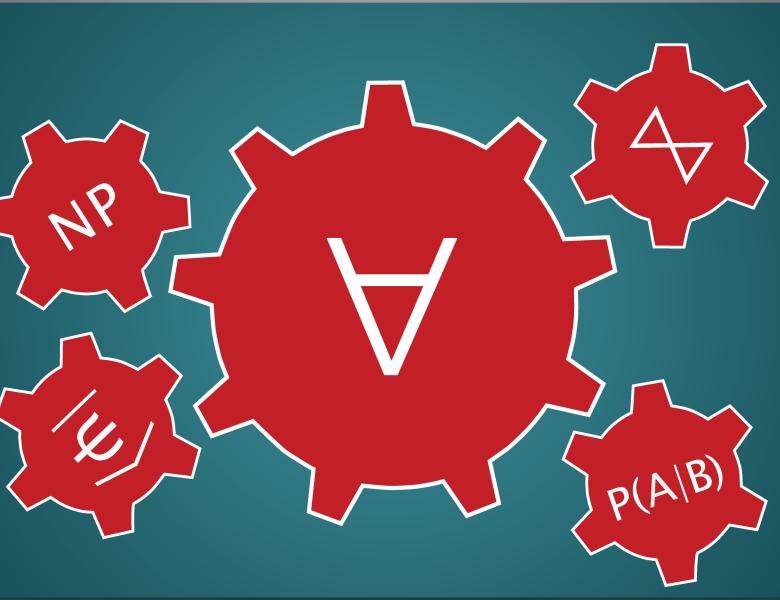
Abstract
Many modern machine learning applications involve sensitive correlated data, such private information on users connected together into a social network, and measurements of physical activity of a single user across time. However, the current gold standard of privacy in machine learning, differential privacy, cannot adequately address privacy issues in this kind of data. This work looks at a recent generalization of differential privacy, called Pufferfish, that can be used to address privacy in correlated data. The main challenge in applying Pufferfish to correlated data problems is the lack of suitable mechanisms. In this talk, I will present two such mechanisms -- a general mechanism, called the Wasserstein Mechanism, which applies to any Pufferfish framework, and a more computationally efficient one, called the Markov Quilt Mechanism, that exploits structural properties of the correlation model for computational efficiency.