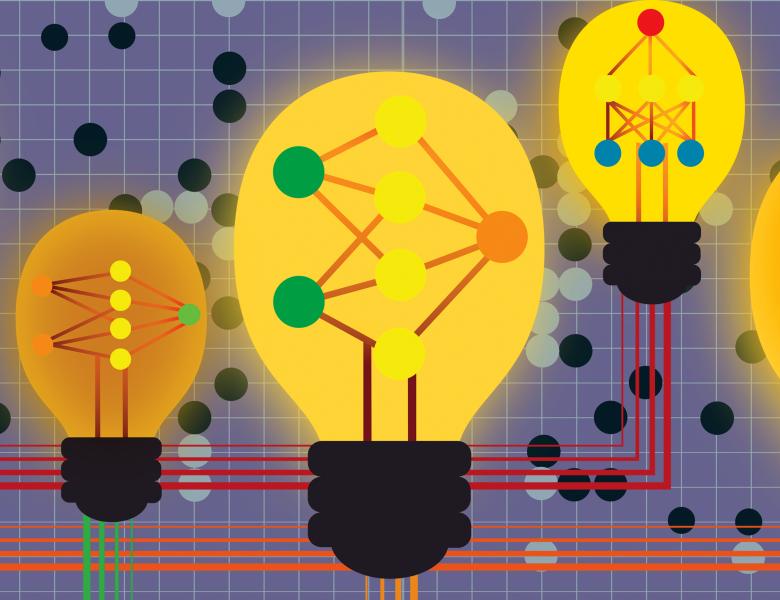
Abstract
In the stochastic multi-player bandit problem, m>1 players cooperate to maximize their total reward on a set of K>m bandit arms. However the players cannot communicate online and are penalized (e.g. receive no reward) if they collide by pulling the same arm at the same time. This problem was introduced in the context of wireless radio communication and serves as a natural model for decentralized online decision-making. There have been many results for different versions of this model. Most rely on a small number of collisions in order to implicitly communicate. However it was later realized that nearly-optimal T^{1/2} regret is possible with no collisions (and hence no communication) at all. I will discuss this construction, as well as our recent characterization of the Pareto optimal trade-offs for gap dependent regret without communication. Based on collaborations with Sebastien Bubeck, Thomas Budzinski, and Allen Liu.