Image
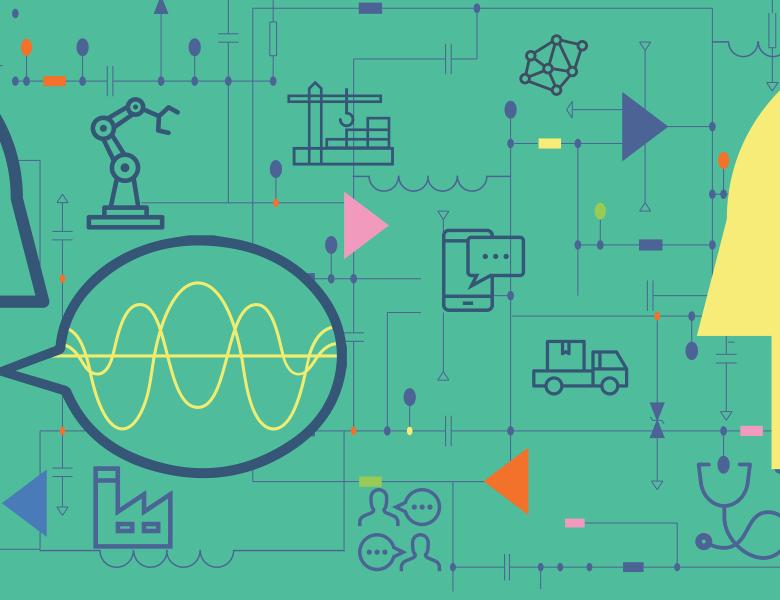
In this talk we discuss computational approaches based on Monte Carlo sampling techniques to solving multistage stochastic programming problems. In some applications the considered programs have a periodical behavior. We demonstrate that in such cases it is possible to drastically reduce the number of stages by introducing a periodical analog of the so-called Bellman equations, used in Markov Decision Processes and Stochastic Optimal Control. Furthermore, we describe a primal - dual variant of
the Stochastic Dual Dynamic Programming algorithm, applied to the constructed periodical Bellman equations. We consider risk neutral and risk averse settings.