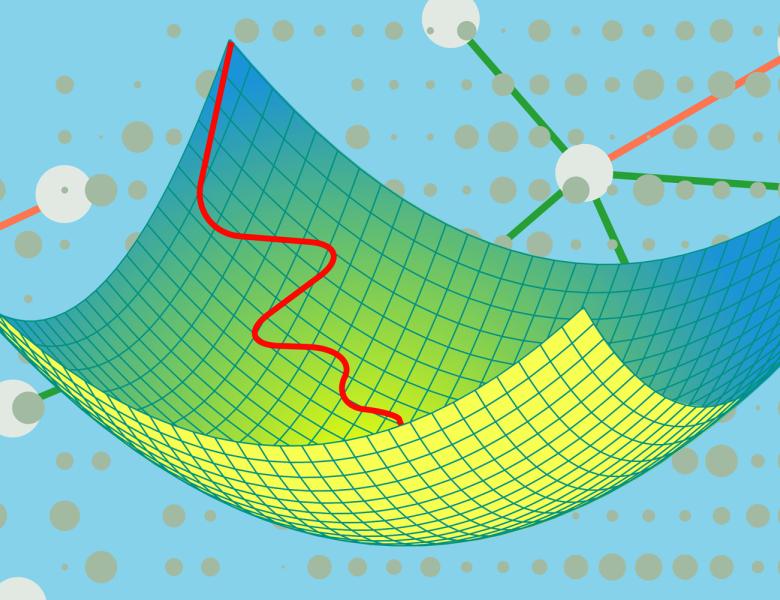
Abstract
I will survey algorithmic applications of a recent structural tool, called short-flat decompositions, developed in the context of solving undercomplete linear inverse problems, e.g. sparse recovery and low-rank matrix completion. Using this structural tool, we develop novel principled approaches for designing iterative methods solving these linear inverse problems, as well as their robust generalizations. We demonstrate that our sparse recovery algorithm extends to solve undercomplete sparse linear systems in RIP design matrices, perturbed by a natural semi-random adversary previously considered in the literature, in nearly-linear time. We also show that our matrix completion algorithm obtains improved noise tolerance (measured in Frobenius norm error) compared to the prior state-of-the-art. Based on joint works at COLT 2023 and FOCS 2023 with Jonathan A. Kelner, Jerry Li, Allen Liu, and Aaron Sidford.