Image
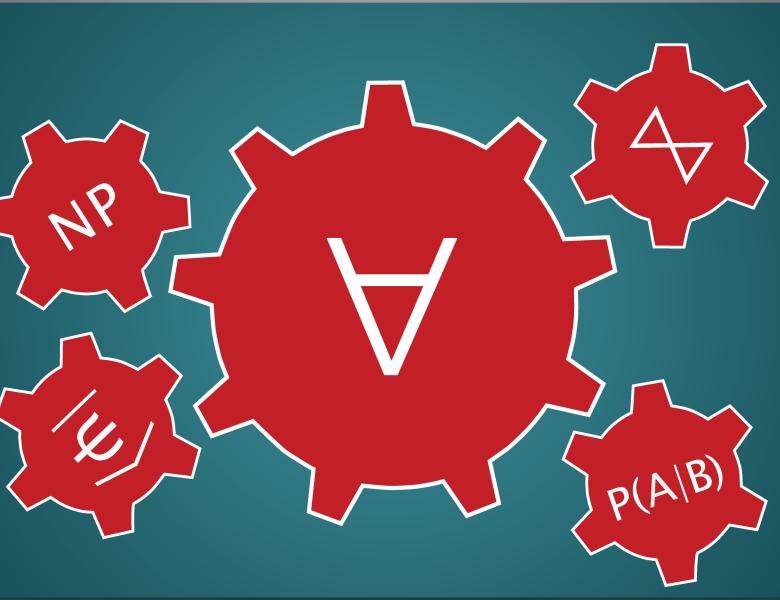
A desired closure property in Bayesian probability is that an updated posterior distribution is in the same class of distributions --- say Gaussians --- as the prior distribution. When the updating takes place via a likelihood, one then calls the class of prior distributions the `conjugate prior' of this likelihood. This talk gives (1) an abstract formulation of this notion of conjugate prior, using channels, in a graphical language, and (2) a simple abstract proof that such conjugate priors yield Bayesian inversions.