Causal Inference: A Guide for Policymakers
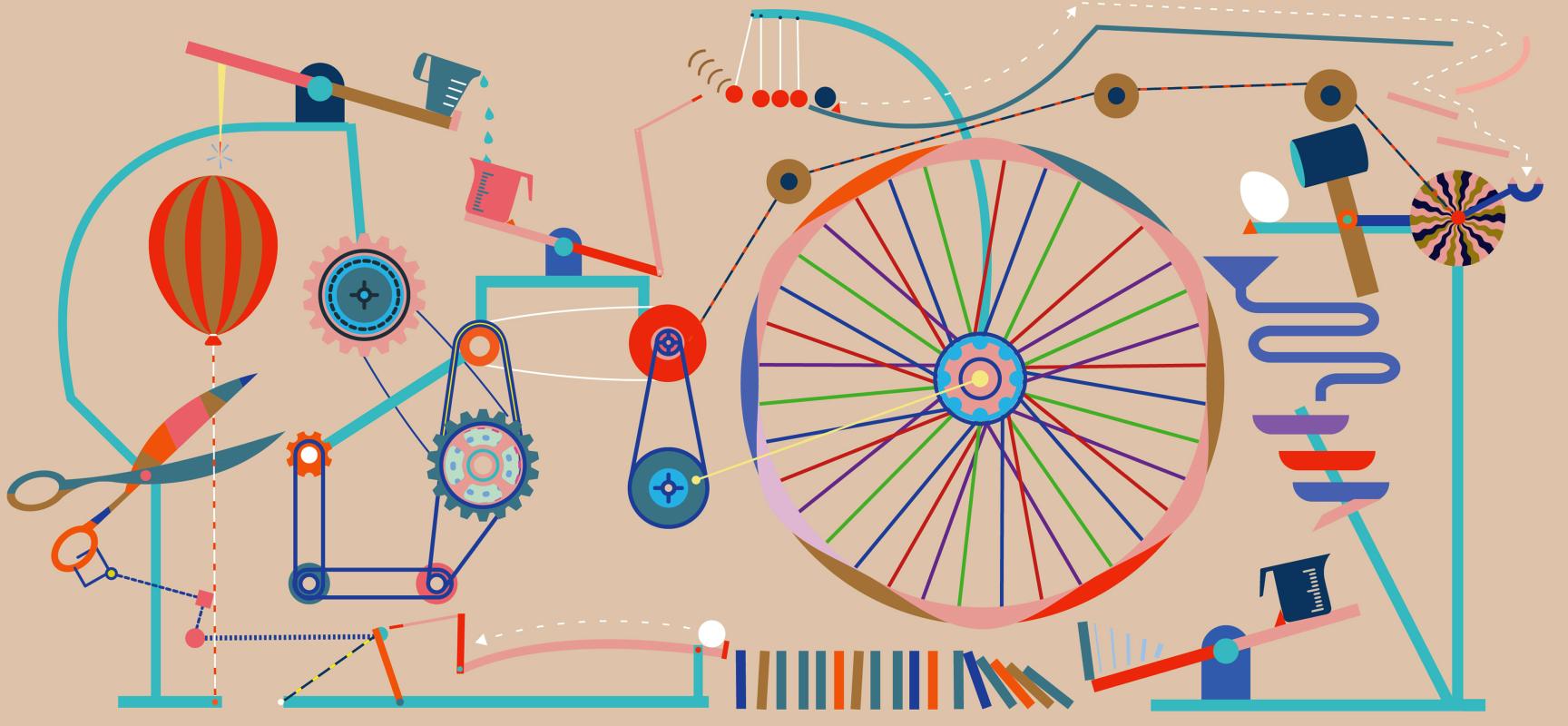
A Simons Institute white paper developed with support from a grant from the Alfred P. Sloan Foundation
by Nathaniel Popper (Science Communicator in Residence, Spring 2022)
Introduction
The reams of data being collected on human activity every minute of every day — from websites and sensors, from hospitals and government agencies — beg to be analyzed and explained. Was the rise in coronavirus infection rates visible in one data set caused by the falling temperatures in another data set, or a result of the mobility patterns apparent in a separate data collection, or was it some other less visible change in social patterns, or perhaps even just random chance, or actually some combination of all these factors?
Finding answers to these sorts of questions is an increasingly urgent task for policymakers and legislators. The answers can point to the most effective laws and interventions in every area from pandemic preparedness to criminal justice, curriculum development, health policy, and labor law, just for starters. It is hard to properly decide on a particular piece of legislation or budget line without knowing how different interventions are likely to lead to different outcomes. All the new data collected on human behavior often promises easy explanations for patterns like rising coronavirus infection rates and declining educational outcomes. But far too often, the explanations that end up being used by commentators and policymakers are based on spurious correlations between various data sets. What should be guiding policy is solid analysis establishing a real causal relationship between a particular intervention and the outcomes visible in the statistics. As Scott Cunningham put it in his approachable introduction to the subject, Causal Inference: The Mixtape:
When the rooster crows, the sun soon after rises, but we know the rooster didn’t cause the sun to rise. Had the rooster been eaten by the farmer’s cat, the sun still would have risen. Yet so often people make this kind of mistake when naively interpreting simple correlations.1
There are, though, a rapidly rising number of sophisticated tools and methods that can help establish the nature of the causal relations hiding in all the data sitting on company servers and government statistics bureaus around the world. Economists, statisticians, and computer scientists have all been honing the art and science of causal inference — the methods used to identify and quantify the elusive links between cause and effect. This has been the focus of decades of work in academia. But the advent of machine learning and big data has offered a world of new opportunities for innovation, and researchers have recently made progress on several fronts. Too often, though, this progress has remained hidden away in the academic realm. One of the top researchers in this area, Susan Athey of Stanford University, wrote in Science that as machine learning experts and scientists “continue to join together in pursuit of solutions to real-world policy problems using big data, we expect that there will be even greater opportunities for methodological advances, as well as successful implementations, of data-driven policy.”2
The newest methods and tools were put on display during the semester-long program on Causality at the Simons Institute for the Theory of Computing in the spring of 2022. One group that presented, led by Devavrat Shah of MIT, has found new ways to parse among the differential impacts that policies can have on subjects of diverse backgrounds and ages. The group has focused on important issues of public policy like the use of police body cameras and the question of how cameras change the work and outcomes of policing. This work has provided a window into how people in different cities or states might react differently to a policy like the introduction of body cameras.3
Avi Feller of UC Berkeley and other statisticians have made advances with a method known as synthetic control. This has been used to evaluate situations in which there is no obviously comparable control group or counterfactual of the sort that scientists have needed to do their analysis in the past.4 This allows policymakers to consider all possible counterfactual (or “unlived”) worlds to evaluate what’s the best course of action for the future.
Meanwhile, James Cussens of the University of Bristol,5 Frederick Eberhardt of Caltech, and other computer scientists have been improving methods for identifying causal relationships in large, complicated data sets about both the human body and brain, as well as the economy, by using causal graphs. The presentations at the Simons Institute made it evident that the surge of research happening around causality is more than can fit even in several workshops over the course of a semester. This work has been the source of a number of recent Nobel Prizes and will likely be the source of more in the years to come. For policymakers, the research has the potential to answer real-world questions about the specific interventions that are the most likely to get them the outcomes they and the public desire.
The Route That Causality Research Has Followed
The work of teasing out cause and effect was not something that traditional economics was particularly good at. That was due, at least in part, to the fact that data was rarely available to test different economic hypotheses 50 or 100 years ago. The founders of the modern social sciences generally came up with theories as to why things happened the way they did based on rough observation and high-level mathematical modeling. That theoretical bent has been changing for decades now as a result of the experimental and causal revolutions in economics and other social sciences. Economists along with sociologists and psychologists have shifted toward using more data to test their theories, supported by careful strategies to identify the parameters of interest through careful research design. This has allowed researchers to test long-held assumptions in their fields, as well as novel questions that the new forms of analysis have opened up for the first time.
The newest research in causality is broadly working with two different types of data that shape the methods being used: data from controlled experiments and data gathered from observations of the world. Some of the most prominent work has involved researchers creating their own data sets through various types of controlled experiments. The team of Esther Duflo and Abhijit Banerjee at MIT won a Nobel Prize in 2019 for their influential work devising experiments to test out different ways of addressing poverty in low-income countries. They used trials in India and Africa to home in on questions about schooling, policing, and financial products, among other topics. People previously had assumptions about how to help poor children get more out of schools, but Duflo and Banerjee tried out the various strategies that were assumed to be effective and found that long-standing assumptions were often wrong. They found, for instance, that improving teacher training and school quality gave children a bigger boost in the long run than adding more free textbooks or additional days in school.
To study these sorts of interventions, Duflo and Banerjee used the randomized controlled trial made famous in the medical sciences. The work of teasing out cause and effect is, at the most basic level, often a job of trying to understand the counterfactual — that is, what would have happened if the policy or intervention in question had not been put in place. A randomized controlled trial provides a tidy way to do this. One group of subjects is given the treatment in question, and another group is not. Because the people are placed in the groups randomly, any variations in outcome should be a result of the treatment rather than any other difference between the two groups.
But researchers have learned over time that even randomized controlled trials can fail to capture the full effect of a particular treatment if externalities are ignored. One of the clearest instances of this is the outcomes visible in COVID-19 vaccine research. When one person gets a COVID-19 booster, it does not just reduce that individual’s chance of getting COVID-19; it also lowers the chances that people in the near vicinity will contract the coronavirus, even if those people did not get the shot themselves. This sort of effect is referred to in causality research as interference, given the way some factor beyond the shot itself can interfere with the ability to get a clean look at the effect of the intervention.
Addressing the Complications with Randomized Trials
Economists have been finding sophisticated methods to identify interference so that it can be isolated in order to get a clearer picture of the pure effect of an intervention as well as the broader effect an intervention could have on group outcomes as a result of the interference. P. M. Aronow and Cyrus Samii did some of the key pioneering work in this area, experimenting on data from an anti-conflict program in a middle school.6 Even if one child did not receive the anti-conflict training, the fact that other children in the same class did receive the training made the child who didn’t get the treatment less likely to end up in a fight. Aronow and Samii used a method known as inverse probability weighting to measure the size of this effect. Since the original paper on this work in 2013, researchers have used similar methods to come up with ways to measure interference in complicated settings far beyond the closely controlled experiment. This work has become increasingly relevant as social scientists have tried to understand the behavioral changes caused by social media sites like Facebook and TikTok. These popular sites influence the thoughts and actions of even individuals who don’t have accounts. Measuring this sort of interference can sometimes be just as important as understanding the effect that social media has on those individuals who are visiting the sites.
The proliferation of randomized controlled trials has also confronted causality researchers with another complication — the fact that different people often respond to the same treatment in different and sometimes contradictory ways. This is yet another area in which the history of medical sciences has provided guidance. Doctors sometimes found that some medicines helped certain patients while actually hurting others. This was not visible when looking at the population as a whole, even in a randomized controlled trial. But after finding a way to identify the distinction between the two groups — the heterogeneity — the results looked entirely different.
Homing in on the sources of heterogeneity has become a central preoccupation for many researchers trying to understand social and economic policies. A change in minimum wage might lead to one sort of outcome for a certain segment of the labor market and a very different outcome for another segment. Spotting these differences has become easier with the high-powered computational resources available to researchers. Machine learning algorithms are able to quickly work through different slices of data to home in on which characteristics seem to be the most important in determining how individuals respond to a particular intervention. Susan Athey, an outspoken proponent of machine learning, has been using these sorts of methods to get more fine-grained results from field trials. In a study at a hospital in Cameroon with economists at the World Bank, Athey and her co-researchers tested out various strategies aimed at getting young women to purchase different types of contraceptives.7 They found that offering discounts helped, especially with younger women, but that certain types of behavioral nudges were even more effective in prompting women to choose long-acting contraceptives like intrauterine devices.
The speed with which researchers can now identify sources of heterogeneity means that some teams have begun to adapt treatments in the middle of experiments in order to further zoom in on the particular populations that gain the most from a particular treatment. In some cases, doing so can make it possible to create “dynamic treatment regimes” that tailor an intervention to a particular individual based on the traits of that person. Molly Offer-Westort, a researcher at the University of Chicago, gave a presentation at the Simons Institute on the proper way to design these adaptive experiments and the potential pitfalls that need to be overcome.8 One place this was used was with a chatbot designed to confront people hesitant to get a COVID-19 vaccine. The adaptive methods were used to quickly determine what messaging was most likely to help individuals overcome their hesitation and to tailor that messaging based on the heterogeneous characteristics of each person. In the search for more adaptive interventions, there has been some — and there is the opportunity for more — cross-pollination with researchers from the tech industry, who have been honing their methods of A/B testing to refine the best ways to reach customers. Offer-Westort referred often in her presentation to the standards already established in the business world that could be brought into the academic realm.
When Controlled Experiments Aren’t Possible
Experiments have been a remarkable source of opportunity for economists. But many of the relationships that researchers want to understand do not lend themselves easily to controlled experiments. To understand the long-term consequences of various family environments — like single-parent households versus homes with grandparents in the mix — it is not possible to randomly assign children to a new family. This is also true of broad economic phenomena like shifts in minimum wages and interest rate policies from central banks. It would be hard to find central bankers willing to submit their policy decisions to randomized experimental design. This has led to an explosion of work on observational data gathered from the everyday occurrences and behaviors captured by sensors and computers around the world. Without the ability to control the people being studied, so as to randomize subjects and create different test groups, social scientists have had to look for other ways to establish the cause of outcomes generated by interventions carried out in the real world. Many researchers have looked for some sort of natural experiment that was carried out in a particular place or on a particular group of people.
In one of the most famous pieces of research in this vein, economists Andrew Card and Alan Krueger examined a policy that raised the minimum wage in New Jersey.9 To understand the effect of the new minimum wage on the labor market in New Jersey, it was not enough to simply compare the labor market in New Jersey after the minimum wage hike with what it had looked like before the law went into effect. That earlier version of New Jersey existed in a previous time with different macroeconomic conditions than the New Jersey that existed after the minimum wage went up. If rising wages coincided with higher unemployment, that might be due to the increased minimum wage, but it might also be due to other changes in the economy that occurred right before or after the minimum wage went up. Those other changes would be the sort of confounding factors that make studying the real economy so hard. But Card and Krueger realized that the New Jersey that existed after the change in policy was otherwise likely to be much more similar to Pennsylvania, the state just next door, both before and after the minimum wage was lifted, especially if the two areas near the border could be compared. The two areas are very likely to otherwise exist in the same economic environment, making any change in unemployment between the two states more likely to be only a result of the change in minimum wage, rather than other variations. Using this method, Card and Krueger found that the increase in minimum wage did not lead to an increase in unemployment among low-wage workers in New Jersey, as traditional economic theory had predicted. Instead, the rate of employment among low-wage workers actually increased. The careful design of the study made it much more convincing because it ruled out other factors that might have lifted employment levels in New Jersey without raising them next door in Pennsylvania.
The method Card and Krueger used is known as difference-in-difference, and it has been employed in countless pieces of economic research since the 1994 publication of the minimum wage paper. But over the years, scientists have come to understand just how messy the sort of observational data used by Card and Krueger can be. Even with randomized controlled trials, the data can easily be influenced by outside factors that make it hard to home in on the exact effect of an intervention. Numerous researchers have attempted to recognize this messiness and have come up with methods that contend with the complications of the real world. James Robins, a professor at Harvard University, has taught and collaborated with several influential followers who have studied how to contend with situations in which the exact size of a causal relationship cannot be ascertained. They have developed a method known as partial identification that allows researchers to put forward numerous possible causal relationships, with probabilities assigned to each one. Robins and one of his students, Thomas Richardson, attended the Simons Institute program on Causality and gave a number of talks in which they explored the wide-ranging implications of the work they have done, on everything from public health to climate modeling. The importance of the work pioneered by Robins was recognized right after the Simons Institute program ended, when Robins and several of his students were awarded in June the 2022 Rousseeuw Prize for Statistics, one of the top honors in the field. At the Simons Institute, even researchers who did not study with Robins demonstrated the influence of his methods. Ismael Mourifié of the University of Toronto presented research in which he used partial identification to test hypotheses for why women are underrepresented in STEM jobs. The work looked at a variety of regions around the world with naturally occurring differences in how women have been integrated into technical fields, in order to understand which of these differences were the most important. The research led by Mourifié found that early social expectations were much more decisive in determining a woman’s willingness to pursue a STEM field than were later efforts to increase female representation and pay.10
The Rise of Synthetic Controls
More recently, a particular approach to difference-in-difference has become the most celebrated method for identifying the causes for outcomes in the real world. In the New Jersey study, Card and Krueger picked Pennsylvania as the natural point of comparison. But Alberto Abadie of MIT argued that picking a single comparison, or even multiple similar cities, was too messy and introduced too much room for bias. Abadie argued for a new method known as synthetic control that uses a wide array of data to create what amounts to an imaginary point of comparison that is more similar to the area subjected to the intervention than any real-world comparison would be. This is particularly helpful in situations where the intervention in question represents a unique circumstance that would be unlikely to reoccur in another setting. For Abadie, the iconic example was a series of terrorist attacks that hit Spain’s Basque region. While other countries had experienced terrorism, those countries were all very different from the Basque region. Those differences introduced innumerable confounding factors that would make comparison difficult.
After presenting his findings on the economic impact of the terrorist attacks in the Basque region, Abadie and a handful of collaborators created standardized methods for generating a synthetic control. This made it possible for the tools to be applied to a wide array of real-world situations. The practice of using synthetic controls has since become one of the most widely employed methods for quantifying the degree to which some real-world event led to a particular set of outcomes. Now, though, the use of synthetic controls is often combined with other innovations in causality research — such as the estimation of various types of interference and the identification of important sources of heterogeneity — to unpack even the most complicated real-world circumstances.
One of the most interesting innovations on the synthetic controls method is known as synthetic interventions. While synthetic controls provide a new way to compare some intervention with a synthetic counterfactual, synthetic intervention uses many of the same mathematical tools to provide a way to predict what would have happened if a different intervention had occurred. This method has been developed in the lab of Devavrat Shah at MIT and been led by his former graduate students Anish Agarwal, who will be joining Columbia University in 2023, and Dennis Shen, a fellow at Berkeley. The synthetic intervention method relies on filling out a three-dimensional matrix known as a tensor, where each of the entries in the matrix represents a different set of background conditions in some real-world situation. As more entries are put into the matrix — using the estimation tools of synthetic controls — more of the other entries can be filled in, providing predictions on how some intervention will play out in numerous different background conditions. At the Simons Institute, Agarwal presented several cases in which the method has already been used to predict outcomes in real-world situations. In one case, the collaborators worked with Uber to determine “passenger wait time if U.S. cities adopted routing policies used in Brazil.” Data from MIT’s Poverty Action Lab was used to figure out the increase in “childhood immunization rates if personalized policies” were used in different locations in India. The method provided useful predictions that showed the promise of this method for helping policymakers predict the outcomes of other interventions.
Aiming for Methods with Fewer Assumptions
Almost all the causal inference work that is of interest to policymakers has involved finding some counterfactual that allows an intervention to be understood by comparing it with a version of the world in which the intervention didn’t occur. The “potential outcomes” school of causal inference has dominated the field. But the potential outcomes framework generally requires researchers to make assumptions about the type of effect a given intervention will have. In the case of the New Jersey minimum wage study, Card and Krueger knew they wanted to look at the outcome on employment levels after the minimum wage went up, and that determined what sort of characteristics they wanted to hold steady in their counterfactual comparison. Now, though, as social scientists want to understand more and more complicated real-world situations, it has been harder to know how to pick the ideal counterfactual. At the same time, some researchers have wanted to overcome the assumptions that have to be made in choosing counterfactuals, with all the subjective biases those assumptions can bring.
A group of theoretically minded researchers has been doing cutting-edge research to develop tools that rely less on the assumptions of the people doing the analysis. Many of these researchers come from the “graphical modeling” school of causal inference, which has used visual graphs to represent the different elements in a causal relationship. The directed acyclic graph (DAG) uses nodes and arrows to represent what interventions cause particular outcomes and what sorts of confounding factors and interference can muddy the picture.
These DAGs are a useful tool for representing and teaching causal relationships. In one instance, a study showed that countries with higher chocolate consumption had more Nobel Prizes. If this type of correlative analysis were used, it would be easy to conclude that eating more chocolate increases the chance of getting a Nobel Prize. But instead, what actually happened here was that there was the confounding factor of a country’s wealth: countries with higher wealth tend to have both higher chocolate consumption and Nobel Prizes. This example can be nicely captured by a DAG with three nodes — wealth, chocolate consumption, and number of Nobel Prizes — and some arrows between them.
DAGs have been harnessed by computer scientists looking to identify the causal relationships — and the DAG that represents them — in real-world situations. In some cases, researchers have started with an understanding of the causal relationships between various nodes. In these cases, the graphs have been used to estimate the size of the effect. DAGs use a mathematical method known as do-calculus to calculate these figures. In some of the most speculative but far-reaching research, scientists have started with observational data and tried to work backward to understand the causal relationships in a system and the DAG that can express those relationships. With the rise of machine learning, there is hope that computers can be taught to find the correct DAG by sifting through troves of data.
This is a controversial area of research, and it has been criticized for moving the assumptions from the human to the computer, given that even machine learning algorithms need to be programmed with assumptions about what they should be trying to identify. Robins and Aronow, leaders in other areas of causal inference research, have questioned how useful DAGs can be on their own, without related experiments.11 But DAG researchers have already provided promising results for scientists studying more complicated natural systems like genetics and the brain. Caroline Uhler, one of the organizers of the Simons Institute’s program on Causality, has used graphic models to understand how the sequence and structure of genes influence the way those genes express themselves. She collaborated with other scientists to investigate the genetic reasons that COVID-19 has been so much more dangerous for older patients than younger ones.12
In similar work being done on the brain, Eberhardt — one of the co-chairs of the Causality program — and his colleagues have helped explain what sorts of human interactions stimulate different parts of the brain.13 Because we understand so little of the brain, researchers have been willing to go in with fewer assumptions and have allowed computers to comb through MRI data to determine what parts of the brain are interacting in response to different stimulation. Recently, though, econometricians and computer scientists have attempted to use these methods to understand similarly complicated economic and financial interactions without relying on as many assumptions. The goal is to learn the causal graph from the data rather than setting out with the graph already in mind.
This work in the economic field is still in its infancy, and many economists are skeptical of whether it will yield useful results. But the opportunity visible here is the possibility that scientists might be able to identify fine-grained causal relationships in complex real-world settings that have eluded human explanation and analysis until now. Elias Bareinboim, a Columbia University professor who studied under the grandfather of DAG research, Judea Pearl, has been a leader in the push to use DAGs in econometric work. At the Simons Institute, Bareinboim presented some of the most promising methods,14 including those used in a paper he co-authored that explained how structured learning could be employed to understand relationships between economic actors. In the paper, Bareinboim concluded:
Until today, the possibilities to completely automatize the identification task, which is a necessary ingredient for causal machine learning, still remain largely unexplored in econometric practice. The applications of do-calculus we have discussed only require the analyst to provide a model of the economic context under study and a description of the available data, the rest can be handled automatically by an algorithm.
Conclusion
Several of these strands of research were put on display during the Simons Institute’s semester-long program on Causality, which provided a window into most of the new methods and approaches to causality discussed in this paper. The seminars and conversations that went on around the bigger talks are already leading to new work that will expand the frontier of how we identify and understand causal inference.
What is remarkable about the broad spectrum of new work on causal inference — especially for policymakers — is the wide variety of circumstances in which researchers have learned how to identify what interventions will lead to particular outcomes. It is no longer necessary to rely on a carefully controlled study or a comparison between two similar situations. Even without the furthest-reaching advances from machine learning, there are already established methods for successfully predicting outcomes from even the knottiest of data sets. The technical methods used in this work can make it seem unapproachable to untutored policymakers. But the underlying principles are often relatively straightforward. Understanding how these methods work, as well as the explanatory power they have, has the potential to lead to better decision-making and better outcomes for the public.
Acknowledgments
I wish to thank Frederick Eberhardt, Ismael Mourifié, Molly Offer-Westort, Sarah Cen, Kristin Kane, and Sandy Irani for their feedback on previous versions of this paper. I also wish to thank the other participants in the Causality program at the Simons Institute for extended conversations and exchange.
-
Cunningham, Scott. Causal Inference: The Mixtape. Accessed November 27, 2022. https://mixtape.scunning.com/01-introduction.↩︎
-
Athey, Susan. “Beyond prediction: Using big data for policy problems.” Science 355, no. 6324 (February 3, 2017): 483–85. https://doi.org/10.1126/science.aal4321.↩︎
-
Shah, Devavrat. “Causalsim: Trace-Driven Simulation for Network Protocols.” Simons Institute for the Theory of Computing, March 22, 2022. https://simons.berkeley.edu/talks/causalsim-trace-driven-simulation-network-protocols.↩︎
-
Feller, Avi. “Balancing Weights for Causal Effects with Panel Data: Some Recent Extensions to the Synthetic Control Method.” Simons Institute for the Theory of Computing, February 16, 2022. https://simons.berkeley.edu/talks/balancing-weights-causal-effects-panel-data-some-recent-extensions-synthetic-control-method.↩︎
-
Cussens, James. “Causality Program Visitor Speaker Series — Encoding Graphs as Vectors for Causal Discovery.” Simons Institute for the Theory of Computing, February 4, 2022. https://simons.berkeley.edu/events/causality-program-visitor-speaker-series-encoding-graphs-vectors-causal-discovery.↩︎
-
Aronow, Peter M., and Cyrus Samii. “Estimating average causal effects under general interference, with application to a social network experiment.” The Annals of Applied Statistics 11, no. 4 (December 2017). https://doi.org/10.1214/16-aoas1005.↩︎
-
Athey, Susan, Katy Bergstrom, Vitor Hadad, Julian C. Jamison, Berk Özler, Luca Parisotto, and Julius Dohbit Sama. “Shared Decision-Making: Can Improved Counseling Increase Willingness to Pay for Modern Contraceptives?,” 2021. https://documents1.worldbank.org/curated/en/454221632144123878/pdf/Shared-Decision-Making-Can-Improved-Counseling-Increase-Willingness-to-Pay-for-Modern-Contraceptives.pdf.↩︎
-
Offer-Westort, Molly. “Designing Adaptive Experiments for Policy Learning and Inference.” Simons Institute for the Theory of Computing, February 16, 2022. https://simons.berkeley.edu/talks/designing-adaptive-experiments-policy-learning-and-inference.↩︎
-
Card, David & Alan B. Krueger. "Minimum Wages and Employment: A Case Study of the Fast-Food Industry in New Jersey and Pennsylvania," American Economic Review, American Economic Association, vol. 84(4), pages 772-793, September 1994.↩︎
-
Mourifié, Ismaël, Marc Henry, and Romuald Méango. “Sharp Bounds and Testability of a Roy Model of STEM Major Choices.” Journal of Political Economy 128, no. 8 (August 2020): 3220–83. https://doi.org/10.1086/708724.↩︎
-
Aronow, P. M., James M. Robins, Theo Saarinen, Fredrik Sävje, and Jasjeet Sekhon. “Nonparametric identification is not enough, but randomized controlled trials are.” ArXiv:2108.11342 [Stat], September 27, 2021. https://arxiv.org/abs/2108.11342.↩︎
-
Anastasiya Belyaeva, Adityanarayanan Radhakrishnan, Chandler Squires, Karren Dai Yang, Caroline Uhler, Louis Cammarata, and G. V. Shivashankar. “Causal network models of SARS-CoV-2 expression and aging to identify candidates for drug repurposing.” Nature Communications 12 (February 15, 2021). https://doi.org/10.1038/s41467-021-21056-z.↩︎
-
Dubois, Julien, Hiroyuki Oya, J. Michael Tyszka, Matthew Howard III, Frederick Eberhardt, and Ralph Adolphs. “Causal mapping of emotion networks in the human brain: Framework and initial findings.” Neuropsychologia 145 (August 2020): 106571. https://doi.org/10.1016/j.neuropsychologia.2017.11.015.↩︎
-
Bareinboim, Elias. “Learning & Reasoning With Soft Interventions.” Simons Institute for the Theory of Computing, February 14, 2022. https://simons.berkeley.edu/talks/tbd-360.↩︎