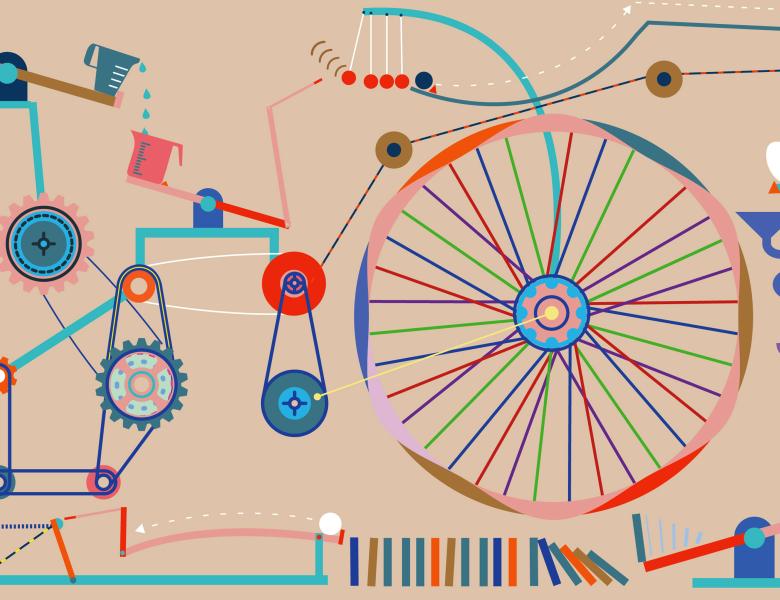
Abstract
The synthetic control method (SCM) is a popular approach for estimating the impact of a treatment on a single unit in panel data settings. The “synthetic control” is a weighted average of control units that balances the treated unit’s pre-treatment outcomes and other covariates as closely as possible. We extend SCM in two ways that expand its potential uses. First, analogous to bias correction for inexact matching, we propose an Augmented SCM estimator that uses an outcome model to estimate the bias in SCM due to imperfect pre-treatment fit and de-biases the SCM estimate. Second, we propose “partially pooled” SCM to extend SCM and ASCM to the staggered adoption setting, where multiple units are treated at different times. The resulting partially pooled SCM weights minimize a weighted combination of the pre-treatment imbalance of the treated group average and of the individual treated units. We connect these proposals to “balancing weights,” a form of inverse propensity score weighting that directly minimizes covariate imbalance, and discuss some other recent extensions. The “augsynth” R package implements the proposed methods. This is based on joint work with Eli Ben-Michael and Jesse Rothstein: https://arxiv.org/abs/1811.04170 (forthcoming at JASA) and https://arxiv.org/abs/1912.03290 (forthcoming at JRSSB).