Care-Coordination Programs and Algorithmic Bias: An Interview With Sendhil Mullainathan
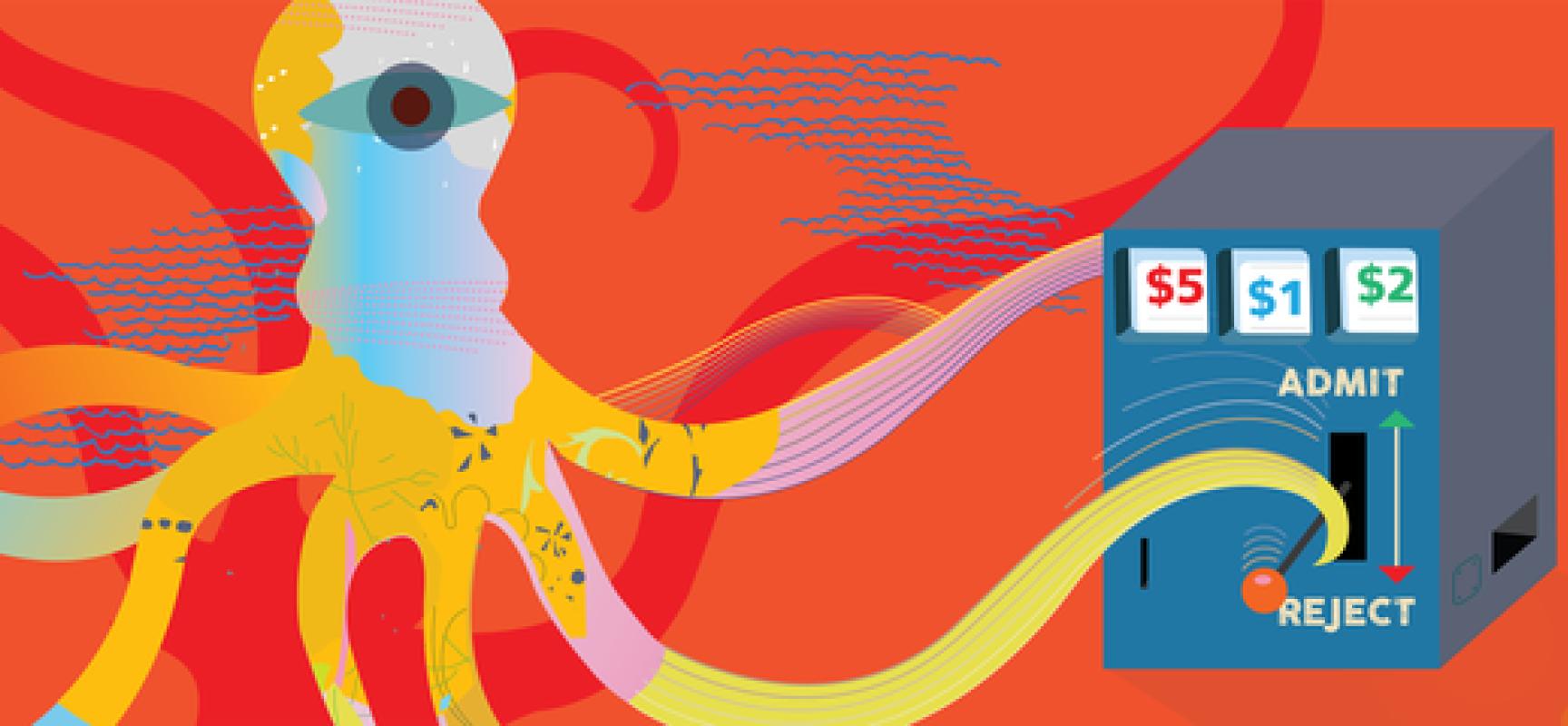
by Shera Avi-Yonah and Ella Rosenthal (Simons Institute Science Journalism Interns)
Sendhil Mullainathan, a professor of computation and behavioral science at the University of Chicago, participated in Wrong at the Root, a workshop on racial bias and algorithms held at the Simons Institute in June. He is one among a growing group of economists interested in algorithmic fairness. We spoke with him after the workshop.
How did you become interested in fairness in health care and fairness and algorithms?
I used to do a lot of work in behavioral science and, in particular, on social issues. So I’ve been working on discrimination for a long time. Typically the problem was trying to understand and quantify the extent or magnitude of discrimination, asking questions like: how much discrimination do African Americans face in the labor market? So that’s the kind of work that I spent a long time doing a while back. When there were no algorithms, that wasn’t the issue. There was one algorithm: the human mind.
Then, in more recent times, we started working on machine learning algorithms applied to social policy. Then it became kind of a natural question. Given that the biggest problem in these areas is discrimination by humans, what do algorithms do? Do they make that problem worse? What do they do to that landscape? It was kind of a natural melding of old and new.
Could you walk us through an example of algorithmic discrimination in greater detail?
We rarely are able to look at algorithmic bias as it plays itself out in the world. Typically we don’t have access to algorithms like Google image search — we don’t know what Google’s optimizing or how Amazon prices things. I don’t know what they’re doing. I don’t even know if there’s a problem or why there’s a problem.
Part of what we were interested in is, can we actually look at things that are out there that we can get access to and actually see what’s happening? Algorithms that help drive people to care-coordination programs were an amazing target because they were driving big decisions. If you are rated as “high need” because of this program, you get access to all these extra health care resources, because we would’ve judged that you have complex health needs. So it really mattered. I didn’t realize this until I began working on this paper, but it’s being used already. The market for these algorithms is probably close to 100 million people.
Given the data we had, we had the ability to actually get access to the algorithm as it was playing itself out as this one big health system. So there were the right ingredients. I think that’s what drew me to the problem. It’s consequential. We can actually study it carefully. So then when we went in, we said, “OK, let’s just do detective exercises, just ask a set of questions and see what they need.” So the first question is: does the algorithm show any sort of disparity?
There’s a literature on how you measure the disparity. But here, I thought, there’s a kind of obvious way to measure it for practical purposes. You want to ask the question: at a similar risk score, are whites less healthy or more healthy?
If you aren’t ranking people well, then if you take everyone above a certain risk score and put them in the program, you’re going to have a lot more of one group than the other. When you look at that, you get kind of a surprise. You say, “Oh, wow, whites are significantly healthier than blacks at a given risk score.” And then the magnitude is big. We can talk about that. But then the question is: what happened? Why is this happening? So that was interesting, because that’s the part where, because we had access to the data and the innards, we could actually diagnose what the problem is.
If we didn’t have access, we would just at this point say, “Oh, look — this algorithm is biased.” Having access, we look deeper. The problem appears to be the objective function, which was given as equating costs with poor health. We measure sickness through the health system, which is monetized, so we tend to say sick people are the ones who cost more. But there’s a problem there, because at the same level of sickness, some groups, like African Americans, can cost less, because they have worse access to care. So then when we kind of disentangled health as a physiological state from dollars that we spend on health, you start to see mechanically what’s happening. At a given level of physiological health, African Americans cost less. Therefore, if you predict costs, the algorithm ends up thinking of African Americans as less sick, whereas in the physiological sense, they’re actually more sick. So that then gives you a very clean answer.
It was helpful for me, because I think if you went into this just absorbing the literature, you’d say, “Where will the problems arise? Oh, it’s going to be in the input data — all the variables were measured with bias. It’s going to be in the human actions that take place before — that generated it.” To a degree, that’s right. It is the human actions that result in African Americans getting less care for their level of sickness. But the problem here was not on the input data — it was on the objective function we chose. We chose to predict cost. We could have chosen to predict health. And that’s a theme that I think I’m seeing in a lot of work. Algorithmic bias in the things we’ve looked at often shows up because we’ve chosen one objective function over another.
That’s something that is very clear-cut guidance as to a very important role for many people to be at the table when designing algorithms. It’s not the technocratic part of how to build the algorithm. It’s asking, “What objective are we given?” Specifically, these algorithms predict things — what’s the thing you’re asking it to predict? Do we really believe that’s what we should be predicting? That’s something that lots of different people should weigh in on and can weigh in on. They don’t need to know the innards of machine learning to figure this out. It’s just what’s the technical given. It’s a kind of thing organizational political processes are comfortable with. I think what’s happened is all of the machinery that we use to make sure our objectives are being set well somehow slips when we call it an algorithm. I just think we should give it the same way.
If we said, “Hey, we’re going to write a vision statement,” we’d have a bunch of people around the table and say, “This is the objective of our organization.” It’s no different. I think the other subtle slip here is that people don’t realize how specific you have to be with the algorithm. I think they’re like, “Oh, well, we thought we were predicting poor health.” But in fact, we were predicting high cost.
I actually walked away optimistic after writing this paper, because I realized that this is the kind of problem that can be very consequential, but we have the levers in place to solve it.
What do you think the path forward is?
I think there are two paths forward.
One is we just don’t have enough on-the-ground real examples that really show us what the ways are in which bias can play itself out or not play itself out, where we have some other examples where the algorithm reduces bias. I think part of what’s exciting about the last three or four years is that there’s been a huge amount of interest in understanding algorithmic bias, which is great. But it’s an interest with not enough real, actually implemented things that we can study. I think theorizing is useful. But theorizing is more effective when there’s some real example which we can get in and dissect and look at. We’re still in the early days. That’s one.
Then for me, because of where I started, I actually think these tools also hold a tremendous amount of promise, not as a force for bias but as a source for reducing our own human biases. I think being able to go forward and say, “We’re now getting very careful about how algorithms can add or exaggerate biases. Let’s have another work stream, which asks, ‘How can algorithms actually serve as a powerful tool for debiasing us as people?’” I’m very optimistic about where this ends up, if everything goes well, if we’ve done a good job of putting the safeguards in place to prevent algorithmic bias. But then we’ve gone to the next phase, where we’ve actually started looking at and implementing things that serve a valuable debiasing role, that actually get rid of all of the stereotypes and everything we have inside of us.
This interview has been edited for length and clarity.
Related Articles
- Letter from the Director, September 2019
- Video Feature: Interview with “Wrong at the Root” Organizers Patricia Williams and Cynthia Dwork
- Workshop Report: Wrong at the Root (June 2019)
- This Summer at the Simons Institute
- From the Inside: Geometry of Polynomials
- From the Inside: Foundations of Data Privacy
- Research Vignette: Lower Bounds in Computational Complexity
- Research Vignette: Foundations of Data Science
- Simons Institute Launches Richard M. Karp Distinguished Lectures
- Prasad Raghavendra Appointed Interim Senior Scientist
- Shafi Goldwasser Receives Honorary Degree from Oxford