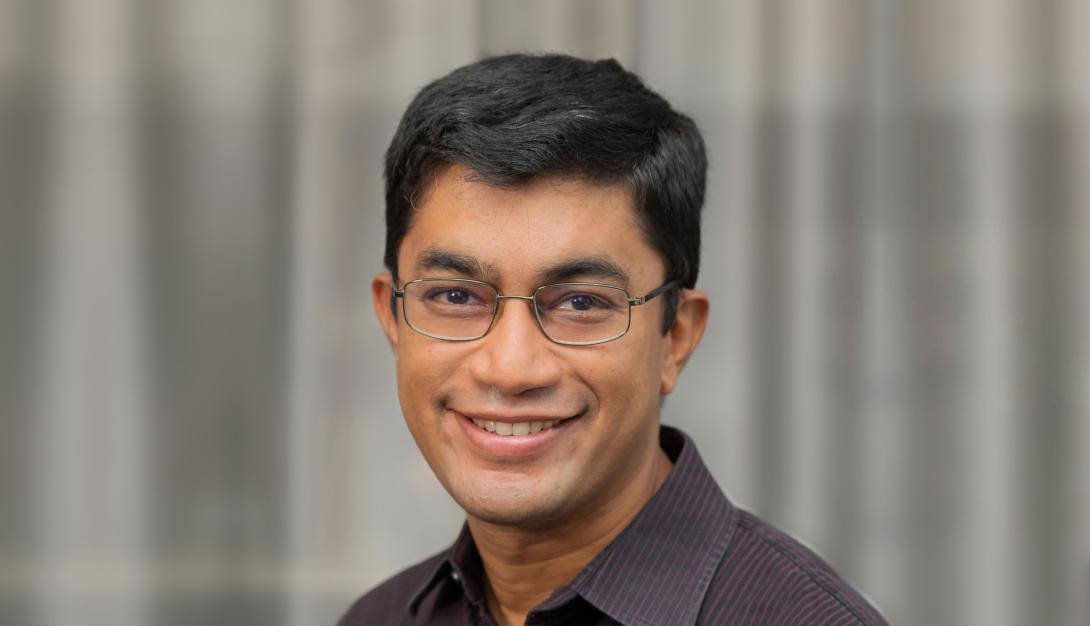
Greetings from Berkeley, where we’ve recently welcomed a merry band of cryptographers for what promises to be an outstanding summer program on...
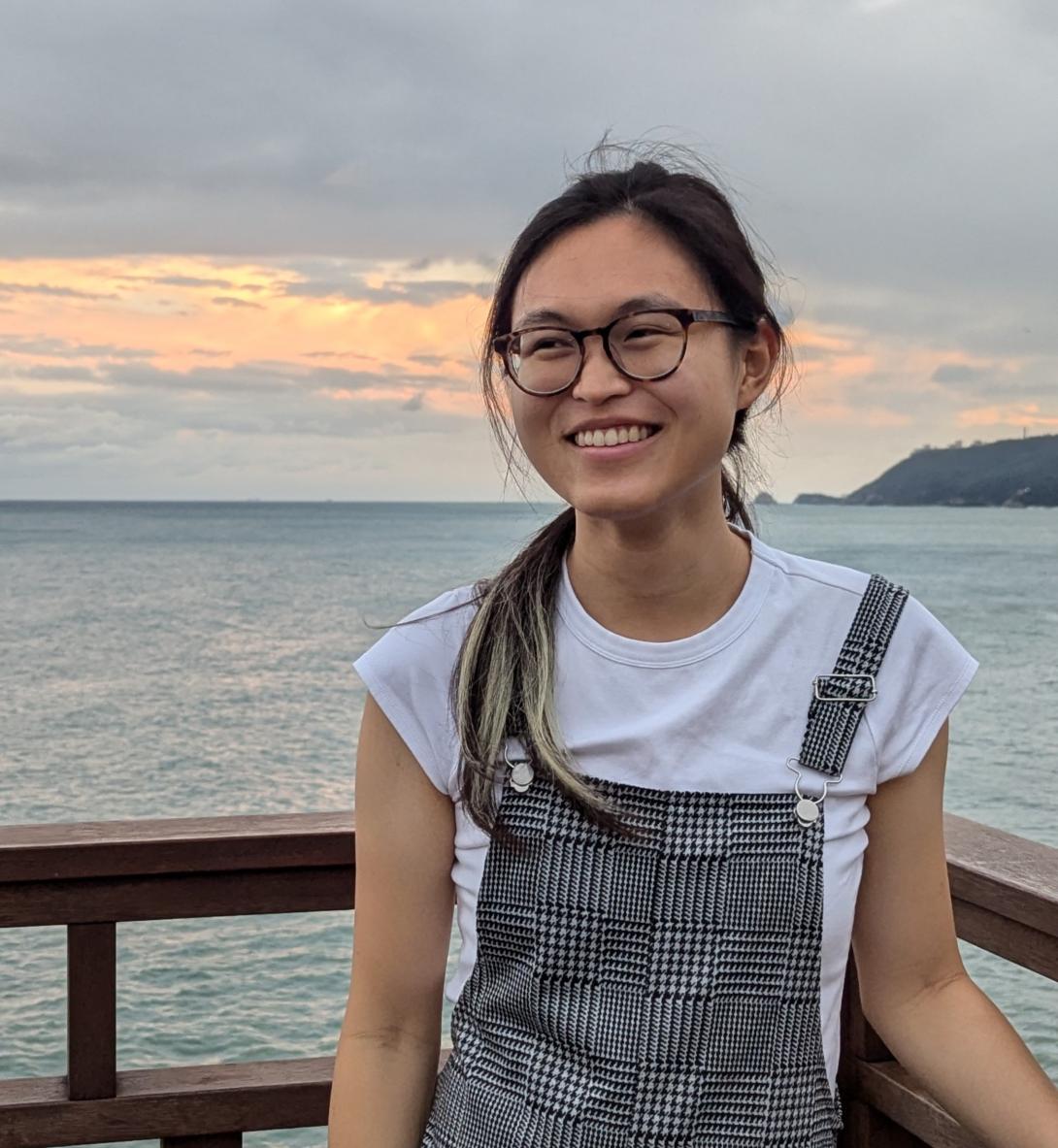
We’re delighted to share that Miller fellow and Simons Institute Quantum Pod postdoc Ewin Tang has been awarded the 2025 Maryam Mirzakhani New...
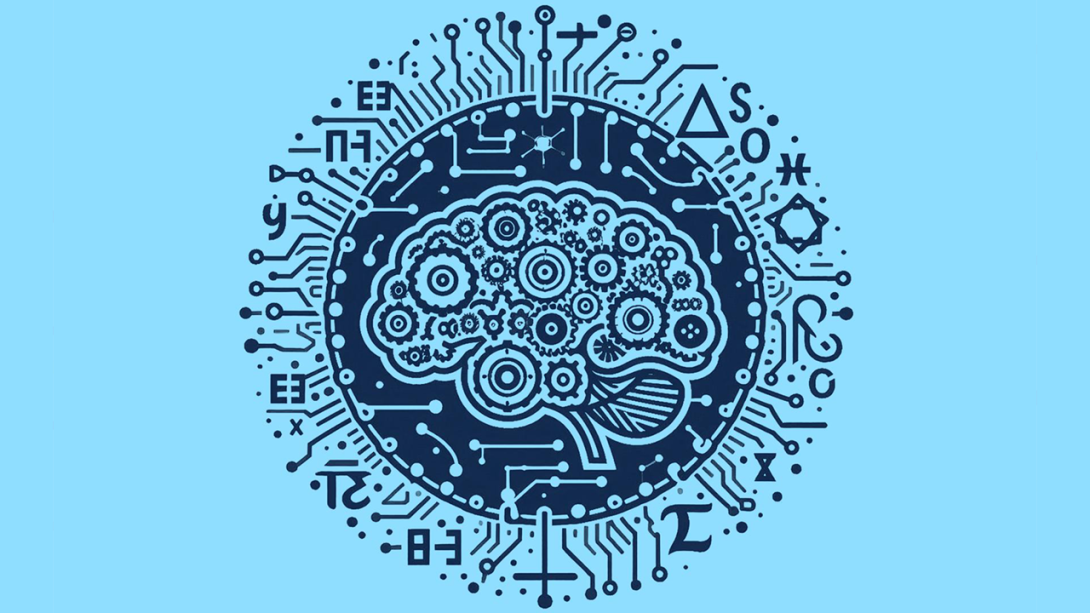
This month, we held a joint workshop with SLMath on AI for Mathematics and Theoretical Computer Science. It was unlike any other Simons Institute...
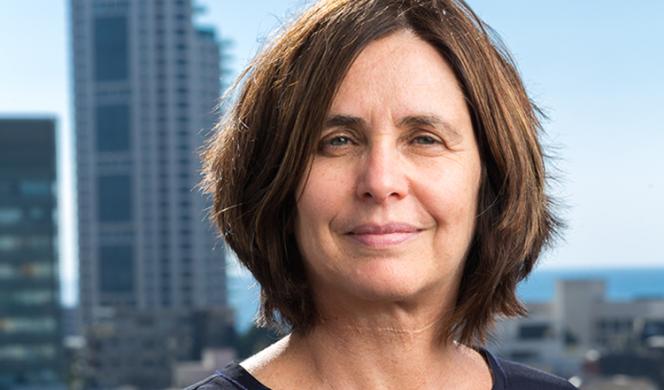
This will be my final letter to you as director of the Simons Institute for the Theory of Computing, as my six-and-a-half-year term ends at the end of this month. These were years well lived. Both the Institute and I experienced challenge, growth, and ultimately a leap into the future. I am proud to have served.

On July 1, Sampath Kannan became the Simons Institute’s new associate director. A UC Berkeley alumnus (PhD 1989), Sampath is the Henry Salvatori Professor in the Department of Computer and Information Science at the University of Pennsylvania. We sat down with him to discuss his research interests, vision for his new role, and perspectives on how developments in AI are transforming the field of theoretical computer science.

For some time, I’ve argued that a common conception of AI is misguided. This is the idea that AI systems like large language and vision models are individual intelligent agents, analogous to human agents. Instead, I’ve argued that these models are “cultural technologies” like writing, print, pictures, libraries, internet search engines, and Wikipedia.

We are heartbroken by the loss of Luca Trevisan, who served as senior scientist at the Institute from 2014 to 2019.

Appreciation and memories of Jim Simons (1938–2024), from the Simons Institute community. Featuring contributions from Avi Wigderson, Dick Karp, Shafi Goldwasser, Bob Birgeneau, Alistair Sinclair, Venkatesan Guruswami, Nikhil Srivastava, Michael Sipser, Tim Roughgarden, Vijay Vazirani, Lin Lin, Virginia Vassilevska Williams, Omer Reingold, Bonnie Berger, Ravindran Kannan, Aviv Regev, Subhash Khot, Noga Alon, Rocco Servedio, Barbara Simons, Bin Yu, Amit Sahai, Shang-Hua Teng, Dan Boneh, Shachar Lovett, Greg Valiant, and Ronitt Rubinfeld.
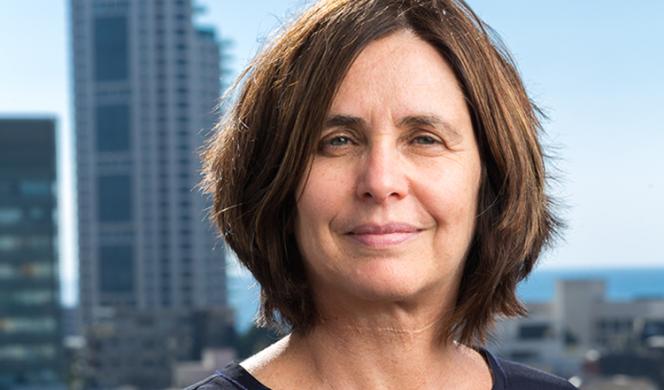
Dear friends,
Jim Simons, the larger-than-life mathematician-entrepreneur-philanthropist to whom the Simons Institute for the Theory of Computing owes its existence, passed away last month. Many of those in our research community have been reflecting on Jim’s wisdom, generosity, and humanity over the last few weeks. I share some of those remembrances with you in this issue of the newsletter, along with the obituary published by Berkeley News. We bid farewell to a great man.
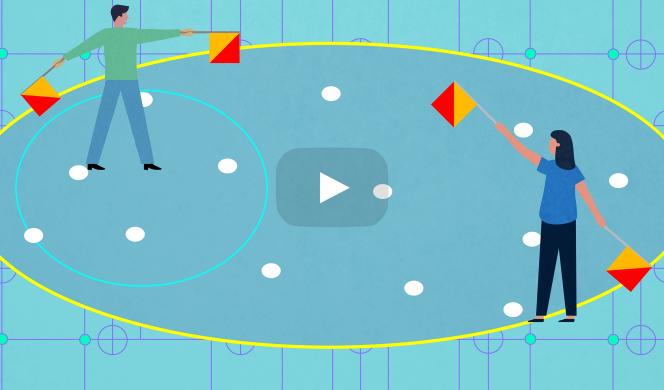
Sivakanth Gopi (Microsoft Research) describes recent developments in the theory of higher-order MDS codes and their surprising connections to central coding-theoretic concepts like list decoding and maximal recoverability.
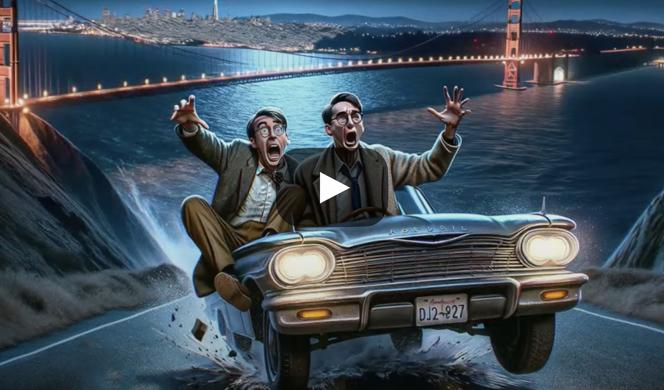
In this presentation from the Workshop in Honor of Michael Ben-Or, John Preskill (Caltech) gives a historical tour of some important developments by Ben-Or and others in quantum fault tolerance.

We share here one of our favorite talks from the workshop on Near-Term Quantum Computers: Fault Tolerance + Benchmarking + Quantum Advantage + Quantum Algorithms. Yunchao Liu (UC Berkeley) presents joint work with Zeph Landau (UC Berkeley).
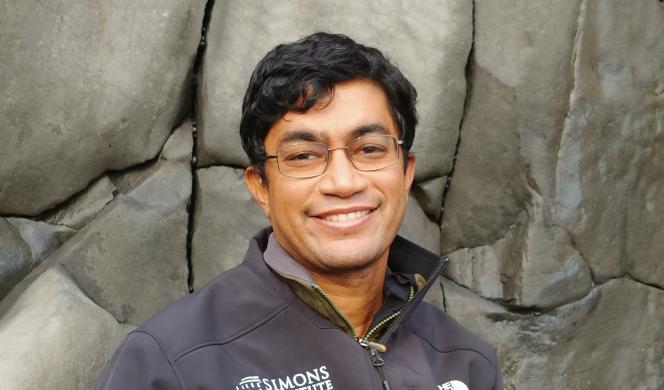
Simons Institute Senior Scientist Venkatesan Guruswami, along with Bingkai Lin, Yican Sun, and Berkeley theory graduate students Xuandi Ren and Kewen Wu, received a best paper award at the 56th ACM Symposium on Theory of Computing conference, for their paper, "Parameterized Inapproximability Hypothesis under ETH."