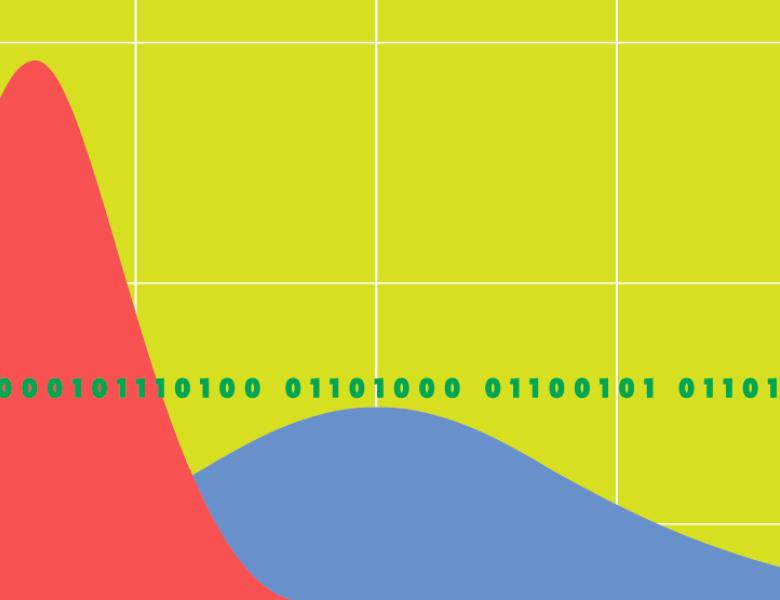
The ongoing COVID-19 pandemic represents an unprecedented global crisis and serves as a reminder of the social, economic, and health burden of infectious diseases. This panel discussion aims to explore the role that computational and statistical tools can play in supporting policy makers as they formulate and assess policies to control COVID-19. It brings together experts in network science, data-driven modeling, and feedback control theory to discuss how these tools might help to understand the progress of an epidemic, to forecast its future course, to infer properties of a disease, and to choose public policy responses.
Peter Bartlett is the Associate Director of the Simons Institute for the Theory of Computing and professor in EECS and Statistics at the University of California at Berkeley. His research interests include machine learning and statistical learning theory, and he is the co-author of the book Neural Network Learning: Theoretical Foundations. He has been Institute of Mathematical Statistics Medallion Lecturer, winner of the Malcolm McIntosh Prize for Physical Scientist of the Year, Australian Laureate Fellow, Fellow of the IMS, Fellow of the ACM, and Fellow of the Australian Academy of Science.
Klaske van Heusden is a research associate at the University of British Columbia in Vancouver. Her research interests include modeling, prediction, and control — with applications in medical devices, mechatronics, and robotics. Lately, she has been working on a robust and provably safe automated drug-delivery device for use in operating rooms.
Madhav Marathe is a passionate advocate and practitioner of transdisciplinary team science. During his 25-year professional career, he has established and led a number of large transdisciplinary projects and groups. His areas of expertise are computational epidemiology, network science, artificial intelligence, high performance computing, and data analytics. Professor Marathe has published more than 350 articles in peer reviewed journals, conferences and workshops. Over the last 20 years, his division has supported federal authorities in their effort to combat epidemics in real-time, including the H1N1 pandemic in 2009 and the Ebola outbreak in 2014. He is a Fellow of the American Association for the Advancement of Science (AAAS), Society for Industrial and Applied Mathematics (SIAM), Association for Computing Machinery (ACM), and Institute of Electrical and Electronics Engineers (IEEE). Currently, his team is developing computer models and analytical tools to support US federal agencies and the state of Virginia in their efforts to combat the COVID-19 pandemic.
Ankur Moitra is an associate professor at MIT. He received his BS in electrical and computer engineering from Cornell in 2007. He completed his MS and PhD degrees from MIT in computer science in 2009 and 2011, respectively, where he was advised by Tom Leighton and was supported by a Fannie and John Hertz Foundation Fellowship. He received a George M. Sprowls Award (best thesis) and a William A. Martin Award (best thesis) for his doctoral and master's dissertations. He then spent two years as an NSF CI Fellow at the Institute for Advanced Study, and jointly a senior postdoc in the computer science department at Princeton University. In 2013, he returned to MIT as an assistant professor in applied mathematics, and a principal investigator in CSAIL. His research spans a diverse set of topics, from statistical inference to optimization and approximation to codes and combinatorics. He is particularly interested in algorithmic problems with applications in machine learning and big data.
Shai Shalev-Shwartz is a senior fellow at Intel, the CTO of Intel’s Mobileye, and a professor in the Faculty of Computer Science and Engineering at the Hebrew University of Jerusalem. He leads software and algorithm technology development for Mobileye’s autonomous driving solutions. Professor Shalev-Shwartz is the author of Online Learning and Online Convex Optimization, and a co-author of Understanding Machine Learning: From Theory to Algorithms. His research is around the theoretical foundations of machine learning, optimization algorithms, and mathematical models of safety. He received several best paper awards, and in 2016 was ranked 17th in The-Marker's top 100 most influential people in Israel.
Anil Vullikanti is a professor in the Department of Computer Science and the Biocomplexity Institute at the University of Virginia. His research interests are in the areas of combinatorial optimization, distributed computing, dynamical systems, network science, data mining, and multi-agent systems; and in using algorithmic tools from these areas to obtain rigorous solutions for problems with immediate social impact in epidemiology and public health policy planning, and critical infrastructures. His research has been supported by an NSF CAREER and a DOE Early Career Award.
Bin Yu is the Chancellor’s Distinguished Professor and Class of 1936 Second Chair in the Departments of Statistics and of Electrical Engineering & Computer Sciences at the University of California at Berkeley, and a former chair of Statistics at UC Berkeley. Her research focuses on practice, algorithm, and theory of statistical machine learning and causal inference. Her group is engaged in interdisciplinary research with scientists from genomics, neuroscience, and precision medicine. She is a member of the U.S. National Academy of Sciences and a fellow of the American Academy of Arts and Sciences. She was a Guggenheim Fellow in 2006 and President of IMS (Institute of Mathematical Statistics) in 2014.
Theoretically Speaking is a lecture series highlighting exciting advances in theoretical computer science for a broad general audience. Events are free and open to the public. No special background is assumed. This panel discussion will be held via Zoom webinar. Please use the Q&A feature to participate.
All scheduled dates:
Upcoming
No Upcoming activities yet