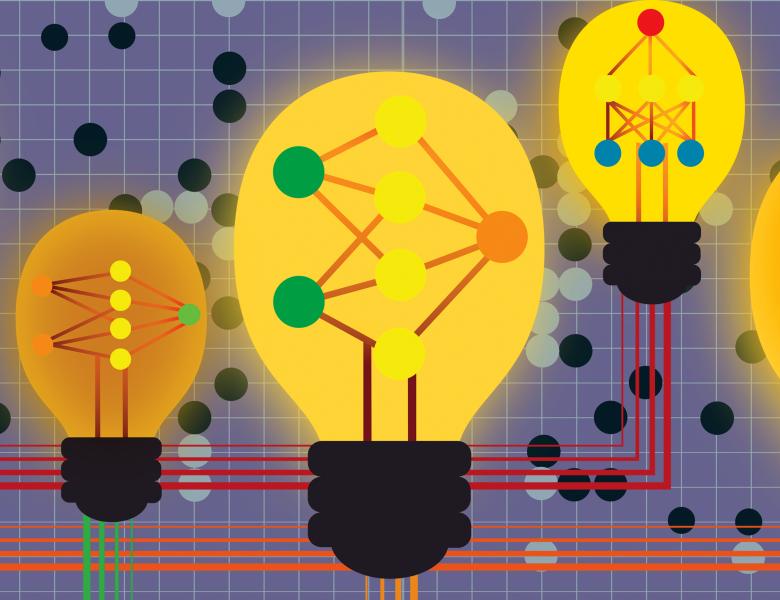
Title: On Convergence and Stability of Learning Dynamics in Continuous Games
Abstract: We study a dynamic setting in which a public information platform updates a belief estimate of a continuous game parameter based on available data of strategies and payoffs. Players adjust their strategies by accounting for the repeatedly updated belief. The long-term behavior of the resulting stochastic learning dynamics is based on endogenous and non-i.i.d. data that is generated by players’ strategic decisions. We develop new tools to tackle the dynamic interplay between parameter learning and strategy learning in continuous games. We present results on the convergence and stability of such learning dynamics and develop conditions for convergence to complete information equilibrium.
Furthermore, we apply this learning model to analyze the impact of information platforms on the strategic behavior of travelers in urban transportation systems. We show that our results can be used to design adaptive tolling mechanisms with travelers learning their routing decisions in traffic networks.
Bio: Manxi Wu is a research fellow at the University of California, Berkeley, in the learning and games program at the Simons Institute. In summer 2022, she will start as an assistant professor in Cornell’s School of Operations Research and Information Engineering. Her fields of interest are game theory, machine learning, engineering systems, and the theory of economic incentives. Her research analyzes strategic interactions between technologies and human users under the physical constraints and uncertainty of socio-technical systems. She also designs information and incentive mechanisms to improve system efficiency and resiliency. Manxi received her Ph.D. in social and engineering systems at MIT. She also holds an M.S. in Transportation at MIT and B.S. in applied mathematics from Peking University.
All scheduled dates:
Upcoming
No Upcoming activities yet